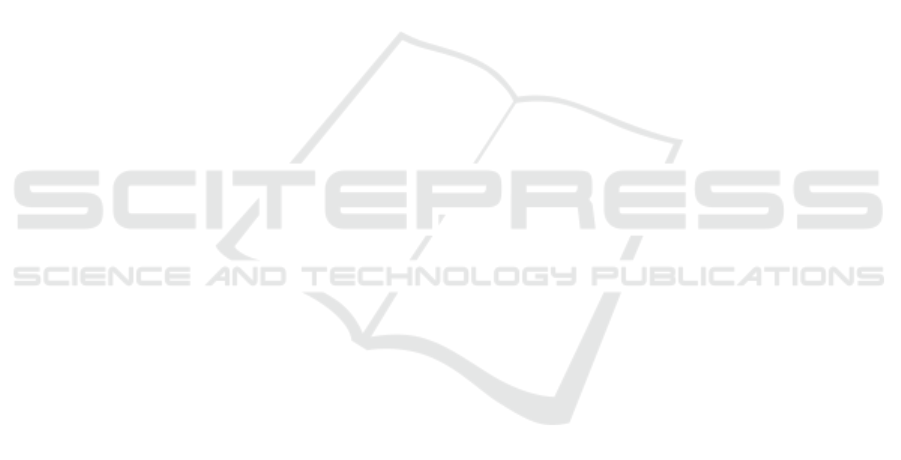
editing with text-guided diffusion models. arXiv
preprint arXiv:2112.10741.
openverse. Openverse website. https://wordpress.org/
openverse/. Accessed: 2022-12-04.
Radford, A., Kim, J. W., Hallacy, C., Ramesh, A., Goh, G.,
Agarwal, S., Sastry, G., Askell, A., Mishkin, P., Clark,
J., et al. (2021). Learning transferable visual models
from natural language supervision. In International
Conference on Machine Learning, pages 8748–8763.
PMLR.
Radford, A., Narasimhan, K., Salimans, T., Sutskever, I.,
et al. (2018). Improving language understanding by
generative pre-training.
Ramesh, A., Dhariwal, P., Nichol, A., Chu, C., and
Chen, M. (2022). Hierarchical text-conditional im-
age generation with clip latents. arXiv preprint
arXiv:2204.06125.
Ramesh, A., Pavlov, M., Goh, G., Gray, S., Voss, C., Rad-
ford, A., Chen, M., and Sutskever, I. (2021). Zero-shot
text-to-image generation. In International Conference
on Machine Learning, pages 8821–8831. PMLR.
Reed, S., Akata, Z., Yan, X., Logeswaran, L., Schiele, B.,
and Lee, H. (2016). Generative adversarial text to im-
age synthesis. In International conference on machine
learning, pages 1060–1069. PMLR.
Rombach, R., Blattmann, A., Lorenz, D., Esser, P., and
Ommer, B. (2022). High-resolution image synthesis
with latent diffusion models. In Proceedings of the
IEEE/CVF Conference on Computer Vision and Pat-
tern Recognition, pages 10684–10695.
Ronneberger, O., Fischer, P., and Brox, T. (2015). U-net:
Convolutional networks for biomedical image seg-
mentation. In International Conference on Medical
image computing and computer-assisted intervention,
pages 234–241. Springer.
Saharia, C., Chan, W., Saxena, S., Li, L., Whang, J., Den-
ton, E., Ghasemipour, S. K. S., Ayan, B. K., Mahdavi,
S. S., Lopes, R. G., et al. (2022). Photorealistic text-
to-image diffusion models with deep language under-
standing. arXiv preprint arXiv:2205.11487.
Saharia, C., Ho, J., Chan, W., Salimans, T., Fleet, D. J.,
and Norouzi, M. (2021). Image super-resolution via
iterative refinement.
Shen, S., Li, L. H., Tan, H., Bansal, M., Rohrbach, A.,
Chang, K.-W., Yao, Z., and Keutzer, K. (2021). How
much can clip benefit vision-and-language tasks?
arXiv preprint arXiv:2107.06383.
Sohl-Dickstein, J., Weiss, E., Maheswaranathan, N., and
Ganguli, S. (2015). Deep unsupervised learning us-
ing nonequilibrium thermodynamics. In International
Conference on Machine Learning, pages 2256–2265.
PMLR.
Song, Y., Sohl-Dickstein, J., Kingma, D. P., Kumar, A.,
Ermon, S., and Poole, B. (2020). Score-based gen-
erative modeling through stochastic differential equa-
tions. arXiv preprint arXiv:2011.13456.
Szegedy, C., Vanhoucke, V., Ioffe, S., Shlens, J., and Wo-
jna, Z. (2016). Rethinking the inception architecture
for computer vision. In Proceedings of the IEEE con-
ference on computer vision and pattern recognition,
pages 2818–2826.
Van Den Oord, A., Vinyals, O., et al. (2017). Neural discrete
representation learning. Advances in neural informa-
tion processing systems, 30.
Vaswani, A., Shazeer, N., Parmar, N., Uszkoreit, J., Jones,
L., Gomez, A. N., Kaiser, Ł., and Polosukhin, I.
(2017). Attention is all you need. Advances in neural
information processing systems, 30.
Wang, H., Ge, S., Lipton, Z., and Xing, E. P. (2019). Learn-
ing robust global representations by penalizing local
predictive power. In Advances in Neural Information
Processing Systems, pages 10506–10518.
Wikiart. Wikiart website. https://www.wikiart.org/. Ac-
cessed: 2022-12-04.
wikimedia. Wikimedia commons website. https://
commons.wikimedia.org. Accessed: 2022-12-04.
Zhang, H., Xu, T., Li, H., Zhang, S., Wang, X., Huang,
X., and Metaxas, D. N. (2018). Stackgan++: Realis-
tic image synthesis with stacked generative adversar-
ial networks. IEEE transactions on pattern analysis
and machine intelligence, 41(8):1947–1962.
ICPRAM 2023 - 12th International Conference on Pattern Recognition Applications and Methods
596