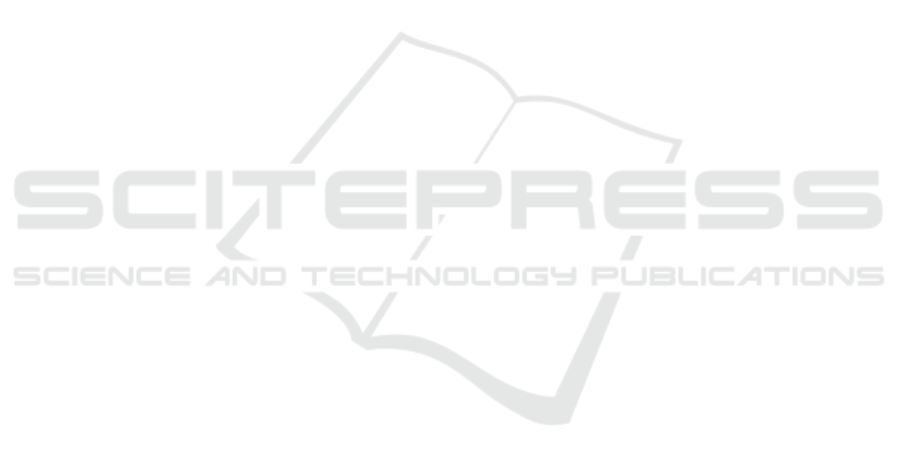
of Germany (BMBF), funding program Photonics
Research Germany (FKZ: 13N15466, 13N15710,
13N15708) and is integrated into the Leibniz Cen-
ter for Photonics in Infection Research (LPI). The
LPI initiated by Leibniz-IPHT, Leibniz-HKI, UKJ
and FSU Jena is part of the BMBF national roadmap
for research infrastructures.
REFERENCES
Ali, N., Girnus, S., R
¨
osch, P., Popp, J., and Bocklitz, T.
(2018). Sample-size planning for multivariate data: a
raman-spectroscopy-based example. Analytical chem-
istry, 90(21):12485–12492.
Azpicueta-Ruiz, L. A., Zeller, M., Figueiras-Vidal, A. R.,
Arenas-Garcia, J., and Kellermann, W. (2010). Adap-
tive combination of volterra kernels and its application
to nonlinear acoustic echo cancellation. IEEE Trans-
actions on Audio, Speech, and Language Processing,
19(1):97–110.
Bach, S., Binder, A., Montavon, G., Klauschen, F., M
¨
uller,
K.-R., and Samek, W. (2015). On pixel-wise explana-
tions for non-linear classifier decisions by layer-wise
relevance propagation. PloS one, 10(7):e0130140.
Bocklitz, T. (2019). Understanding of non-linear parametric
regression and classification models: A taylor series
based approach. In ICPRAM, pages 874–880.
Dosovitskiy, A., Beyer, L., Kolesnikov, A., Weissenborn,
D., Zhai, X., Unterthiner, T., Dehghani, M., Minderer,
M., Heigold, G., Gelly, S., et al. (2020). An image is
worth 16x16 words: Transformers for image recogni-
tion at scale. arXiv preprint arXiv:2010.11929.
Franz, M. O. and Sch
¨
olkopf, B. (2006). A unifying view
of wiener and volterra theory and polynomial kernel
regression. Neural computation, 18(12):3097–3118.
Halicek, M., Dormer, J. D., Little, J. V., Chen, A. Y., and
Fei, B. (2020). Tumor detection of the thyroid and
salivary glands using hyperspectral imaging and deep
learning. Biomedical Optics Express, 11(3):1383–
1400.
Kather, J. N., Weis, C.-A., Bianconi, F., Melchers, S. M.,
Schad, L. R., Gaiser, T., Marx, A., and Z
¨
ollner, F. G.
(2016). Multi-class texture analysis in colorectal can-
cer histology. Scientific reports, 6(1):1–11.
Khatri, C. and Rao, C. R. (1968). Solutions to some func-
tional equations and their applications to characteriza-
tion of probability distributions. Sankhy
¯
a: The Indian
Journal of Statistics, Series A, pages 167–180.
Korenberg, M. J. and Hunter, I. W. (1996). The identifi-
cation of nonlinear biological systems: Volterra ker-
nel approaches. Annals of biomedical engineering,
24(2):250–268.
Krizhevsky, A., Hinton, G., et al. (2009). Learning multiple
layers of features from tiny images.
Lin, H., Deng, F., Zhang, C., Zong, C., and Cheng, J.-X.
(2019). Deep learning spectroscopic stimulated raman
scattering microscopy. In Multiphoton Microscopy in
the Biomedical Sciences XIX, volume 10882, pages
207–214. SPIE.
Lundberg, S. M. and Lee, S.-I. (2017). A unified approach
to interpreting model predictions. Advances in neural
information processing systems, 30.
Mishra, S., Sturm, B. L., and Dixon, S. (2017). Local inter-
pretable model-agnostic explanations for music con-
tent analysis. In ISMIR, volume 53, pages 537–543.
Montavon, G., Lapuschkin, S., Binder, A., Samek, W., and
M
¨
uller, K.-R. (2017). Explaining nonlinear classifica-
tion decisions with deep taylor decomposition. Pat-
tern recognition, 65:211–222.
Niioka, H., Asatani, S., Yoshimura, A., Ohigashi, H.,
Tagawa, S., and Miyake, J. (2018). Classification of
c2c12 cells at differentiation by convolutional neural
network of deep learning using phase contrast images.
Human cell, 31(1):87–93.
Orcioni, S. (2014). Improving the approximation ability
of volterra series identified with a cross-correlation
method. Nonlinear Dynamics, 78(4):2861–2869.
Orcioni, S., Terenzi, A., Cecchi, S., Piazza, F., and Carini,
A. (2018). Identification of volterra models of tube au-
dio devices using multiple-variance method. Journal
of the Audio Engineering Society, 66(10):823–838.
Rodner, E., Bocklitz, T., von Eggeling, F., Ernst, G., Cher-
navskaia, O., Popp, J., Denzler, J., and Guntinas-
Lichius, O. (2019). Fully convolutional networks in
multimodal nonlinear microscopy images for auto-
mated detection of head and neck carcinoma: Pilot
study. Head & neck, 41(1):116–121.
Seber, G. A. (2008). A matrix handbook for statisticians.
John Wiley & Sons.
Simonyan, K., Vedaldi, A., and Zisserman, A. (2013).
Deep inside convolutional networks: Visualising im-
age classification models and saliency maps. arXiv
preprint arXiv:1312.6034.
Stegmayer, G. (2004). Volterra series and neural networks
to model an electronic device nonlinear behavior. In
2004 IEEE International Joint Conference on Neu-
ral Networks (IEEE Cat. No. 04CH37541), volume 4,
pages 2907–2910. IEEE.
Stegmayer, G., Pirola, M., Orengo, G., and Chiotti, O.
(2004). Towards a volterra series representation from
a neural network model. WSEAS Transactions on Sys-
tems, 3(2):432–437.
Sundararajan, M., Taly, A., and Yan, Q. (2017). Axiomatic
attribution for deep networks. In International confer-
ence on machine learning, pages 3319–3328. PMLR.
Vaswani, A., Shazeer, N., Parmar, N., Uszkoreit, J., Jones,
L., Gomez, A. N., Kaiser, Ł., and Polosukhin, I.
(2017). Attention is all you need. Advances in neural
information processing systems, 30.
ICPRAM 2023 - 12th International Conference on Pattern Recognition Applications and Methods
606