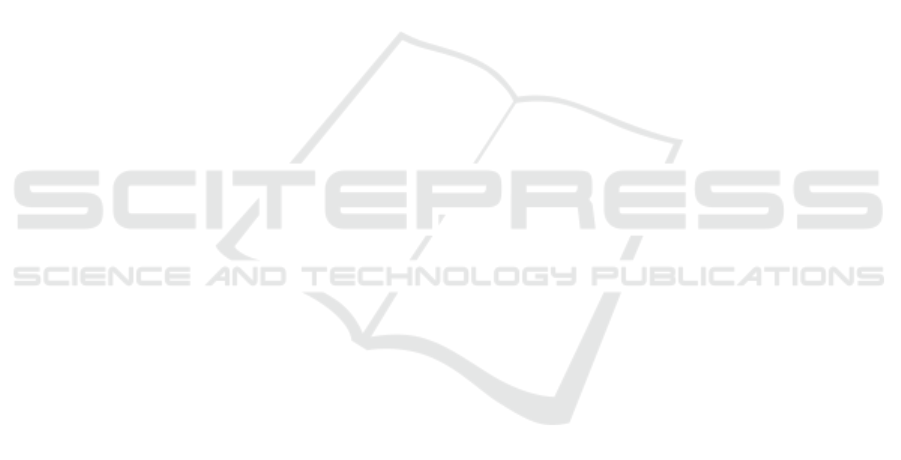
and there is a relationship between the information
thus obtained and the morphological information dis-
played in HE images. Moreover, initial experiments
show that this relationship can be modeled by ma-
chine learning models such as neural networks.
However, it’s just the beginning of our research on
this topic. Challenges still exist, including:
1. The registration of tissue pairs needs to be done
manually, or human intervention is needed to find
the correct parameters of the automated approach,
which additionally needs to be visually inspected.
In the future, it would be necessary to develop
an automatic registration approach also with sub-
sequent validation of the correctness of the key-
points matching.
2. The relationship between HE and IHC-stained tis-
sue can be very complex due to the diversity of
tissues, cells and antibodies used to produce IHC.
Our research has so far focused on only one spe-
cific protein, Ki67, so it would be necessary to
verify whether this approach works on other types
of HE and IHC pairs.
Our future work will mainly focus on the follow-
ing aspects. Firstly, enlarge our dataset to contain
more samples, so the model trained on the new dataset
will have stronger ability of generalization. In this
step, we will also include new tissue samples whose
HE and IHC sections followed each other immedi-
ately to ensure as much tissue identity on them as
possible. Secondly, conduct more experiments and
optimize our model.
ACKNOWLEDGEMENTS
This publication has been produced with the support
of the Integrated Infrastructure Operational Program
for the projects ”New possibilities for the manage-
ment of serious diseases in medical and preventive
care with regard to the safety of health profession-
als”, ITMS:313011AUA5 and “Integrated strategy in
the development of personalized medicine of selected
malignant tumor diseases and its impact on life qual-
ity”, ITMS code: 313011V446, both co-financed by
the European Regional Development Fund.
REFERENCES
Abousamra, S., Gupta, R., Hou, L., Batiste, R., Zhao, T.,
Shankar, A., Rao, A., Chen, C., Samaras, D., Kurc, T.,
and Saltz, J. (2021). Deep learning-based mapping of
tumor infiltrating lymphocytes in whole slide images
of 23 types of cancer. Front. Oncol., 11:806603.
Al-Dulaimi, K., Banks, J., Nugyen, K., Al-Sabaawi, A.,
Tomeo-Reyes, I., and Chandran, V. (2021). Segmen-
tation of white blood cell, nucleus and cytoplasm in
digital haematology microscope images: A review-
challenges, current and future potential techniques.
IEEE Rev. Biomed. Eng., 14:290–306.
Boukhar, S. A., Gosse, M. D., Bellizzi, A. M., and Rajan
K D, A. (2021). Ki-67 Proliferation Index Assess-
ment in Gastroenteropancreatic Neuroendocrine Tu-
mors by Digital Image Analysis With Stringent Case
and Hotspot Level Concordance Requirements. Amer-
ican Journal of Clinical Pathology, 156(4):607–619.
Hameed, Z., Zahia, S., Garcia-Zapirain, B., Javier Aguirre,
J., and Mar
´
ıa Vanegas, A. (2020). Breast can-
cer histopathology image classification using an en-
semble of deep learning models. Sensors (Basel),
20(16):4373.
Han, J., Kamber, M., and Pei, J. (2012). 10 - cluster analy-
sis: Basic concepts and methods. In Han, J., Kamber,
M., and Pei, J., editors, Data Mining (Third Edition),
The Morgan Kaufmann Series in Data Management
Systems, pages 443–495. Morgan Kaufmann, Boston,
third edition edition.
He, L., Long, L. R., Antani, S., and Thoma, G. R. (2012).
Histology image analysis for carcinoma detection
and grading. Computer Methods and Programs in
Biomedicine, 107(3):538–556.
Klimo, M., Luk
´
a
ˇ
c, P., and Tar
´
abek, P. (2021). Deep neu-
ral networks classification via binary error-detecting
output codes. Appl. Sci. (Basel), 11(8):3563.
Klimo, M., Tar
´
abek, P.,
ˇ
Such, O., Smie
ˇ
sko, J., and
ˇ
Skvarek,
O. (2016). Implementation of a deep relu neuron net-
work with a memristive circuit. International Journal
of Unconventional Computing, 12(4):319 – 337.
Kos, Z. and Dabbs, D. J. (2016). Biomarker assessment and
molecular testing for prognostication in breast cancer.
Histopathology, 68(1):70–85.
Kouzehkanan, Z. M., Saghari, S., Tavakoli, S., Rostami,
P., Abaszadeh, M., Mirzadeh, F., Satlsar, E. S., Ghei-
dishahran, M., Gorgi, F., Mohammadi, S., and Hos-
seini, R. (2022). A large dataset of white blood cells
containing cell locations and types, along with seg-
mented nuclei and cytoplasm. Sci. Rep., 12(1):1123.
Li, L. T., Jiang, G., Chen, Q., and Zheng, J. N. (2015). Ki67
is a promising molecular target in the diagnosis of can-
cer (review). Mol. Med. Rep., 11(3):1566–1572.
Liu, Y., Li, X., Zheng, A., Zhu, X., Liu, S., Hu, M., Luo,
Q., Liao, H., Liu, M., He, Y., and Chen, Y. (2020).
Predict ki-67 positive cells in H&E-stained images us-
ing deep learning independently from IHC-stained im-
ages. Front. Mol. Biosci., 7:183.
Lowe, D. G. (2004). Distinctive image features from scale-
invariant keypoints. International Journal of Com-
puter Vision, 60(2):91–110.
Madhulatha, T. S. (2012). An overview on clustering meth-
ods. CoRR, abs/1205.1117.
Naik, N., Madani, A., Esteva, A., Keskar, N. S., Press,
M. F., Ruderman, D., Agus, D. B., and Socher, R.
(2020). Deep learning-enabled breast cancer hor-
Semi-Automated Workflow for Computer-Generated Scoring of Ki67 Positive Cells from HE Stained Slides
299