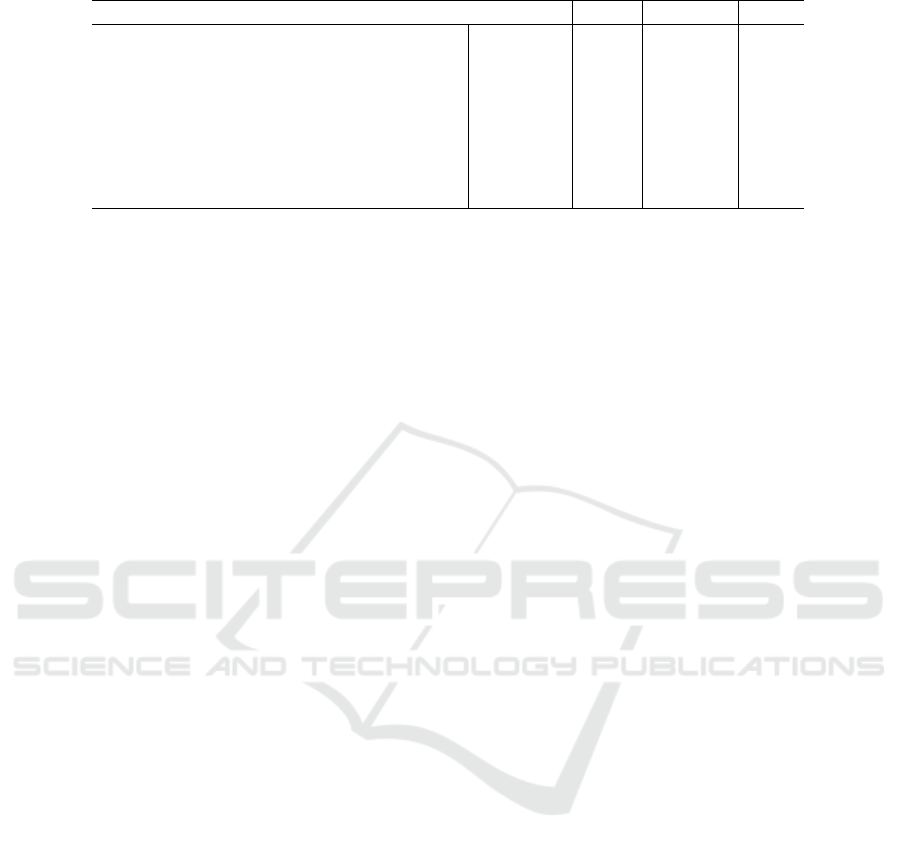
Table 3: Comparison of the accuracy of our approach with methods from the state of the art.
Methods MSRC v1 Wang Holidays UKB
BoVW (Csurka et al., 2004) 0.48 0.41 0.50 2.95
SaCoCo(Iakovidou et al., 2019) - 0.51 0.76 3.33
CEDD (Chatzichristofis and Boutalis, 2008) - 0.54 0.72 3.24
VLAD (J
´
egou et al., 2010) - - 0.53 3.17
N-Gram (Pedrosa and Traina, 2013) - 0.34 - -
Grid (Ouni et al., 2021) 0.67 0.62 0.64 3.57
Fisher (Perronnin and Dance, 2007) - - 0.69 3.07
Ours 0.69 0.65 0.68 3.61
4 CONCLUSION
In this paper, we present an effective bag of visual
phrase model dependent on grouping approach. We
show that the utilization of density clustering ap-
proach joined with BoVW model increment the CBIR
precision. Utilizing two descriptors (KAZE, SURF),
our methodology accomplish a superior outcomes
compared to the state of the art methods.
REFERENCES
Ankerst, M., Breunig, M., Kriegel, H., Ng, R., and Sander,
J. (2008). Ordering points to identify the clustering
structure. In Proc. ACM SIGMOD, volume 99.
Arandjelovic, R. and Zisserman, A. (2013). All about vlad.
In Proceedings of the IEEE conference on Computer
Vision and Pattern Recognition, pages 1578–1585.
Bay, H., Tuytelaars, T., and Van Gool, L. (2006). Surf:
Speeded up robust features. In European conference
on computer vision, pages 404–417. Springer.
Chatzichristofis, S. A. and Boutalis, Y. S. (2008). Cedd:
Color and edge directivity descriptor: A compact de-
scriptor for image indexing and retrieval. In Interna-
tional conference on computer vision systems, pages
312–322. Springer.
Chen, T., Yap, K.-H., and Zhang, D. (2014). Discriminative
soft bag-of-visual phrase for mobile landmark recog-
nition. IEEE Transactions on Multimedia, 16(3):612–
622.
Csurka, G., Dance, C., Fan, L., Willamowski, J., and Bray,
C. (2004). Visual categorization with bags of key-
points. In Workshop on statistical learning in com-
puter vision, ECCV, volume 1, pages 1–2. Prague.
Iakovidou, C., Anagnostopoulos, N., Lux, M.,
Christodoulou, K., Boutalis, Y., and Chatzichristofis,
S. A. (2019). Composite description based on salient
contours and color information for cbir tasks. IEEE
Transactions on Image Processing, 28(6):3115–3129.
J
´
egou, H., Douze, M., Schmid, C., and P
´
erez, P. (2010).
Aggregating local descriptors into a compact image
representation. In 2010 IEEE computer society con-
ference on computer vision and pattern recognition,
pages 3304–3311. IEEE.
Krizhevsky, A., Sutskever, I., and Hinton, G. E. (2012). Im-
agenet classification with deep convolutional neural
networks. In Advances in neural information process-
ing systems, pages 1097–1105.
Leutenegger, S., Chli, M., and Siegwart, R. Y. (2011).
Brisk: Binary robust invariant scalable keypoints. In
Computer Vision (ICCV), 2011 IEEE International
Conference on, pages 2548–2555. IEEE.
Lindeberg, T. (2012). Scale invariant feature transform.
Mehmood, Z., Anwar, S. M., Ali, N., Habib, H. A., and
Rashid, M. (2016). A novel image retrieval based on
a combination of local and global histograms of visual
words. Mathematical Problems in Engineering, 2016.
Ouni, A., Royer, E., Chevaldonn
´
e, M., and Dhome, M.
(2021). Robust visual vocabulary based on grid clus-
tering. In Intelligent Decision Technologies, pages
221–230. Springer.
Pedrosa, G. V. and Traina, A. J. (2013). From bag-of-
visual-words to bag-of-visual-phrases using n-grams.
In 2013 XXVI Conference on Graphics, Patterns and
Images, pages 304–311. IEEE.
Perronnin, F. and Dance, C. (2007). Fisher kernels on visual
vocabularies for image categorization. In 2007 IEEE
conference on computer vision and pattern recogni-
tion, pages 1–8. IEEE.
Ren, Y., Bugeau, A., and Benois-Pineau, J. (2013). Visual
object retrieval by graph features.
Rublee, E., Rabaud, V., Konolige, K., and Bradski, G.
(2011). Orb: An efficient alternative to sift or surf.
In Computer Vision (ICCV), 2011 IEEE international
conference on, pages 2564–2571. IEEE.
Schubert, E., Sander, J., Ester, M., Kriegel, H. P., and Xu,
X. (2017). Dbscan revisited, revisited: why and how
you should (still) use dbscan. ACM Transactions on
Database Systems (TODS), 42(3):1–21.
Simonyan, K. and Zisserman, A. (2014). Very deep con-
volutional networks for large-scale image recognition.
arXiv preprint arXiv:1409.1556.
Szegedy, C., Ioffe, S., Vanhoucke, V., and Alemi, A. A.
(2017). Inception-v4, inception-resnet and the impact
of residual connections on learning. In Thirty-first
AAAI conference on artificial intelligence.
Wang, J. Z., Li, J., and Wiederhold, G. (2001). Simplicity:
An Unsupervised IR Approach Based Density Clustering Algorithm
495