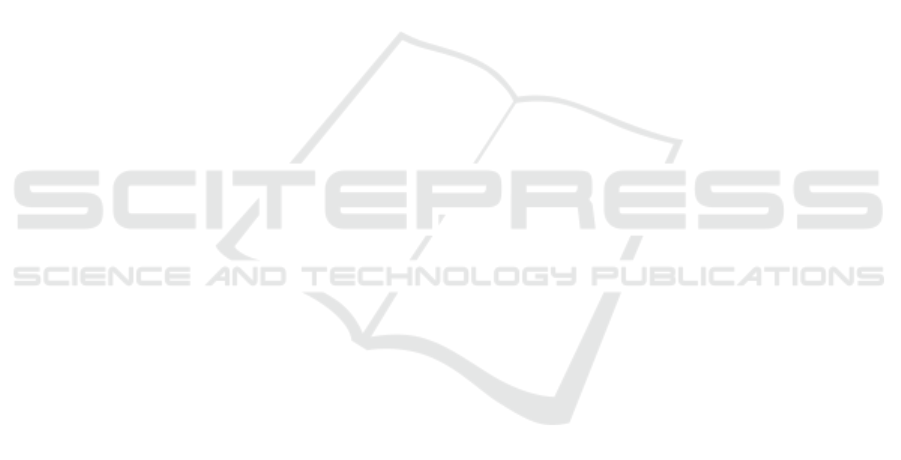
through the projects Learn Real (ANR-18-CHR3-
0002-01), Chiron (ANR-20-IADJ-0001-01), Aristo-
tle (ANR-21-FAI1-0009-01), and the joint support of
the French national program of investment of the fu-
ture and the regions through the PSPC FAIR Waste
project.
REFERENCES
Ayinde, B. O., Inanc, T., and Zurada, J. M. (2019). Regu-
larizing deep neural networks by enhancing diversity
in feature extraction. IEEE Transactions on Neural
Networks and Learning Systems.
Beery, S., Van Horn, G., and Perona, P. (2018). Recognition
in terra incognita. In IEEE/CVF European conference
on computer vision.
Benz, P., Zhang, C., Karjauv, A., and Kweon, I. S. (2021).
Revisiting batch normalization for improving corrup-
tion robustness. In IEEE/CVF Winter Conference on
Applications of Computer Vision.
Carlucci, F. M., D’Innocente, A., Bucci, S., Caputo, B., and
Tommasi, T. (2019). Domain generalization by solv-
ing jigsaw puzzles. In IEEE/CVF Conference on Com-
puter Vision and Pattern Recognition.
DeGrave, A. J., Janizek, J. D., and Lee, S.-I. (2021). Ai for
radiographic covid-19 detection selects shortcuts over
signal. Nature Machine Intelligence.
Finn, C., Abbeel, P., and Levine, S. (2017). Model-agnostic
meta-learning for fast adaptation of deep networks. In
International Conference on Machine Learning.
Galanti, T. and Poggio, T. (2022). Sgd noise and implicit
low-rank bias in deep neural networks. arXiv preprint
arXiv:2206.05794.
Geirhos, R., Jacobsen, J.-H., Michaelis, C., Zemel, R.,
Brendel, W., Bethge, M., and Wichmann, F. A. (2020).
Shortcut learning in deep neural networks. Nature
Machine Intelligence.
Gokhale, T., Anirudh, R., Thiagarajan, J. J., Kailkhura, B.,
Baral, C., and Yang, Y. (2022). Improving diversity
with adversarially learned transformations for domain
generalization. arXiv preprint arXiv:2206.07736.
Gulrajani, I. and Lopez-Paz, D. (2021). In search of lost
domain generalization. In International Conference
on Learning Representations.
Havasi, M., Jenatton, R., Fort, S., Liu, J. Z., Snoek, J., Lak-
shminarayanan, B., Dai, A. M., and Tran, D. (2021).
Training independent subnetworks for robust predic-
tion. In International Conference on Learning Repre-
sentations.
He, K., Zhang, X., Ren, S., and Sun, J. (2016). Deep resid-
ual learning for image recognition. In IEEE/CVF Con-
ference on Computer Vision and Pattern Recognition.
Hermann, K., Chen, T., and Kornblith, S. (2020). The ori-
gins and prevalence of texture bias in convolutional
neural networks. Advances in Neural Information
Processing Systems.
Hermann, K. and Lampinen, A. (2020). What shapes
feature representations? exploring datasets, architec-
tures, and training. Advances in Neural Information
Processing Systems.
Hoffman, J., Tzeng, E., Park, T., Zhu, J.-Y., Isola, P.,
Saenko, K., Efros, A., and Darrell, T. (2018). Cy-
cada: Cycle-consistent adversarial domain adaptation.
In International Conference on Machine Learning.
Hu, X., Uzunbas, G., Chen, S., Wang, R., Shah, A., Nevatia,
R., and Lim, S.-N. (2021). Mixnorm: Test-time adap-
tation through online normalization estimation. arXiv
preprint arXiv:2110.11478.
Huang, R., Xu, B., Schuurmans, D., and Szepesv
´
ari, C.
(2015). Learning with a strong adversary. arXiv
preprint arXiv:1511.03034.
Huang, Z., Wang, H., Xing, E. P., and Huang, D. (2020).
Self-challenging improves cross-domain generaliza-
tion. In IEEE/CVF European Conference on Com-
puter Vision.
Huh, M., Mobahi, H., Zhang, R., Cheung, B., Agrawal, P.,
and Isola, P. (2021). The low-rank simplicity bias in
deep networks. arXiv preprint arXiv:2103.10427.
Jackson, P. T., Abarghouei, A. A., Bonner, S., Breckon,
T. P., and Obara, B. (2019). Style augmentation: data
augmentation via style randomization. In IEEE/CVF
Conference on Computer Vision and Pattern Recogni-
tion Workshops.
Krueger, D., Caballero, E., Jacobsen, J.-H., Zhang, A., Bi-
nas, J., Priol, R. L., and Courville, A. (2020). Out-of-
distribution generalization via risk extrapolation (rex).
arXiv preprint arXiv:2003.00688.
Kurakin, A., Goodfellow, I. J., and Bengio, S. (2016). Ad-
versarial machine learning at scale. In International
Conference on Learning Representations.
Li, D., Yang, Y., Song, Y.-Z., and Hospedales, T. M. (2017).
Deeper, broader and artier domain generalization. In
IEEE/CVF International Conference on Computer Vi-
sion.
Li, D., Yang, Y., Song, Y.-Z., and Hospedales, T. M.
(2018a). Learning to generalize: Meta-learning for
domain generalization. In Thirty-Second AAAI Con-
ference on Artificial Intelligence.
Li, H., Jialin Pan, S., Wang, S., and Kot, A. C. (2018b).
Domain generalization with adversarial feature learn-
ing. In IEEE/CVF Conference on Computer Vision
and Pattern Recognition.
Moyer, D., Gao, S., Brekelmans, R., Galstyan, A., and
Ver Steeg, G. (2018). Invariant representations with-
out adversarial training. In Advances in Neural Infor-
mation Processing Systems.
Nado, Z., Padhy, S., Sculley, D., D’Amour, A., Lak-
shminarayanan, B., and Snoek, J. (2020). Eval-
uating prediction-time batch normalization for ro-
bustness under covariate shift. arXiv preprint
arXiv:2006.10963.
Nam, H., Lee, H., Park, J., Yoon, W., and Yoo, D. (2021).
Reducing domain gap by reducing style bias. In
IEEE/CVF Conference on Computer Vision and Pat-
tern Recognition.
VISAPP 2023 - 18th International Conference on Computer Vision Theory and Applications
356