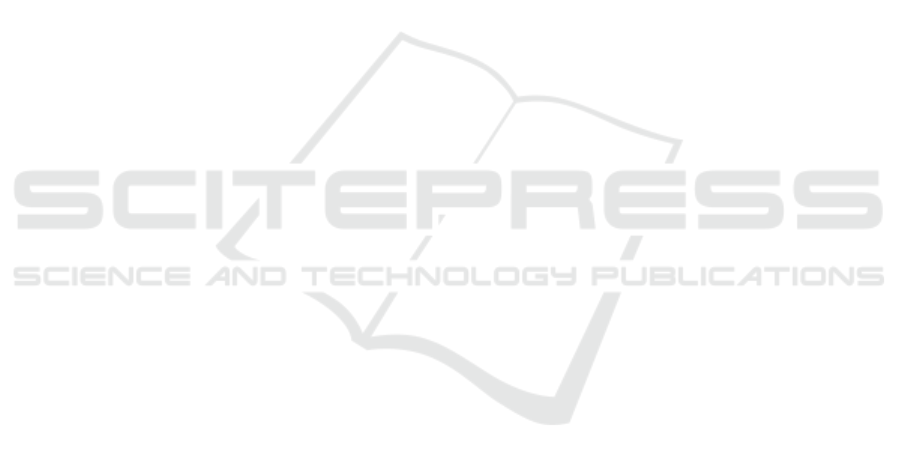
Bank D., Koenigstein N., G. R. (2021). Autoencoders. [On-
line]. Available: https://arxiv.org/abs/2003.05991
Devlin, J., Chang, M.-W., Lee, K., and Toutanova, K.
(2019). Bert: Pre-training of deep bidirectional trans-
formers for language understanding. [Online]. Avail-
able: https://arxiv.org/abs/1810.04805
Goodfellow, I. J., Pouget-Abadie, J., Mirza, M., Xu, B.,
Warde-Farley, D., Ozair, S., Courville, A., and Ben-
gio, Y. (2014). Generative adversarial networks. [On-
line]. Available: https://arxiv.org/abs/1406.2661
Hautamaki, V., Karkkainen, I., and Franti, P. (2004). Out-
lier detection using k-nearest neighbour graph. In Pro-
ceedings of the 17th International Conference on Pat-
tern Recognition, 2004. ICPR 2004., volume 3, pages
430–433 Vol.3.
He, K., Zhang, X., Ren, S., and Sun, J. (2015). Deep resid-
ual learning for image recognition. [Online]. Avail-
able: https://arxiv.org/abs/1512.03385
Hearst, M., Dumais, S., Osuna, E., Platt, J., and Scholkopf,
B. (1998). Support vector machines. IEEE Intelligent
Systems and their Applications, 13(4):18–28.
Hochreiter, S. and Schmidhuber, J. (1997). Long short-term
memory. Neural computation, 9(8):1735–1780.
Kieu, T., Yang, B., Guo, C., and Jensen, C. S. (2019).
Outlier detection for time series with recurrent au-
toencoder ensembles. In Proceedings of the Twenty-
Eighth International Joint Conference on Artificial In-
telligence, IJCAI-19, pages 2725–2732. International
Joint Conferences on Artificial Intelligence Organiza-
tion.
Krizhevsky, A., Sutskever, I., and Hinton, G. E. (2012).
Imagenet classification with deep convolutional neu-
ral networks. In Pereira, F., Burges, C. J. C., Bottou,
L., and Weinberger, K. Q., editors, Advances in Neu-
ral Information Processing Systems 25, pages 1097–
1105. Curran Associates, Inc.
Lee, K., Lee, H., Lee, K., and Shin, J. (2018). Train-
ing confidence-calibrated classifiers for detecting
out-of-distribution samples. [Online]. Available:
https://arxiv.org/abs/1711.09325
Mao, F., Wu, X., Xue, H., and Zhang, R. (2021). Hi-
erarchical video frame sequence representation with
deep convolutional graph network. Computer Vision
– ECCV 2018 Workshops, page 262–270.
Mathur, A. P. and Tippenhauer, N. O. (2016). Swat: a wa-
ter treatment testbed for research and training on ics
security. In 2016 International Workshop on Cyber-
physical Systems for Smart Water Networks (CySWa-
ter), pages 31–36.
Munir, M., Siddiqui, S. A., Dengel, A., and Ahmed, S.
(2019). Deepant: A deep learning approach for un-
supervised anomaly detection in time series. IEEE
Access, 7:1991–2005.
Ranjan, C., Reddy, M., Mustonen, M., Paynabar, K., and
Pourak, K. (2019). Dataset: Rare event classifica-
tion in multivariate time series. [Online]. Available:
https://arxiv.org/abs/1809.10717
Russo, S., Disch, A., Blumensaat, F., and Villez, K. (2020).
Anomaly detection using deep autoencoders for in-
situ wastewater systems monitoring data. [Online].
Available: https://arxiv.org/abs/2002.03843
Schlegl, T., Seeb
¨
ock, P., Waldstein, S. M., Schmidt-
Erfurth, U., and Langs, G. (2017). Unsupervised
anomaly detection with generative adversarial net-
works to guide marker discovery. [Online]. Available:
https://arxiv.org/abs/1703.05921
Sch
¨
olkopf, B., Williamson, R. C., Smola, A. J., Shawe-
Taylor, J., Platt, J. C., et al. (1999). Support vector
method for novelty detection. In NIPS, volume 12,
pages 582–588. Citeseer.
Taormina, R., Galelli, S., Tippenhauer, N. O., Salomons,
E., Ostfeld, A., Eliades, D. G., Aghashahi, M., Sun-
dararajan, R., Pourahmadi, M., Banks, M. K., et al.
(2018). Battle of the attack detection algorithms: Dis-
closing cyber attacks on water distribution networks.
Journal of Water Resources Planning and Manage-
ment, 144(8):04018048.
Thanh-Tung, H. and Tran, T. (2020). On catas-
trophic forgetting and mode collapse in genera-
tive adversarial networks. [Online]. Available:
https://arxiv.org/abs/1807.04015
Vaswani, A., Shazeer, N., Parmar, N., Uszkoreit, J., Jones,
L., Gomez, A. N., Kaiser, L., and Polosukhin, I.
(2017). Attention is all you need. [Online]. Available:
https://arxiv.org/abs/1706.03762
Wang, Y., Wong, J., and Miner, A. (2004). Anomaly in-
trusion detection using one class svm. In Proceedings
from the Fifth Annual IEEE SMC Information Assur-
ance Workshop, 2004., pages 358–364.
Zhang, C., Song, D., Chen, Y., Feng, X., Lumezanu,
C., Cheng, W., Ni, J., Zong, B., Chen, H., and
Chawla, N. V. (2018a). A deep neural network
for unsupervised anomaly detection and diagnosis in
multivariate time series data. [Online]. Available:
https://arxiv.org/abs/1811.08055
Zhang, Z., Song, Y., and Qi, H. (2018b). Decoupled learn-
ing for conditional adversarial networks. [Online].
Available: https://arxiv.org/abs/1801.06790
Anomaly Detection for Multivariate Industrial Sensor Data via Decoupled Generative Adversarial Network
1035