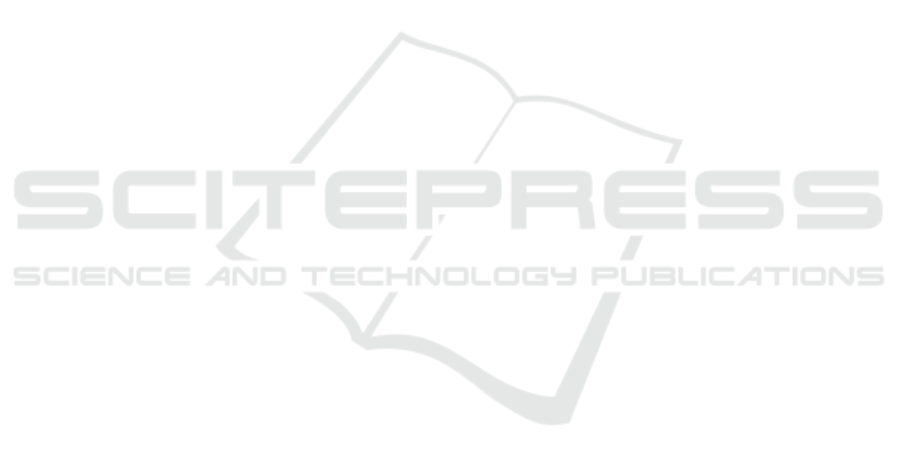
markov decision process approach. Artificial Intelli-
gence in Medicine, 57(1):9–19.
Chen, L. and Yang, G. (2015). Recent advances in circa-
dian rhythms in cardiovascular system. Frontiers in
Pharmacology, 6.
Cottura, N., Howarth, A., Rajoli, R. K., and Siccardi, M.
(2020). The current landscape of novel formulations
and the role of mathematical modeling in their de-
velopment. The Journal of Clinical Pharmacology,
60(S1):S77–S97.
Dickerson, J., Hingorani, A., Ashby, M., Palmer, C., and
Brown, M. (1999). Optimisation of antihypertensive
treatment by crossover rotation of four major classes.
Lancet, 353:2008–2013.
Donnelly, R., Elliott, H., and Meredith, P. (1992). Antihy-
pertensive drugs: Individualized analysis and clinical
relevance of kinetic-dynamic relationships. Pharma-
cology & Therapeutics, 53(1):67–79.
Duffy J, Hirsch M, K. A. G. C. P. L. P. M. S. M. W. P.
K. K. Z. S. and RJ, M. (2017). Outcome reporting
across randomised controlled trials evaluating thera-
peutic interventions for pre-eclampsia. BJOG: An
International Journal of Obstetrics & Gynaecology,
124(12):1829–1839.
Fagard, R. and AL. (2013). 2013 esh/esc guidelines for the
management of arterial hypertension. European Heart
Journal, pages 1–72.
Filip Mussen, Sam Salek, S. W. (2009). Benefit-Risk
Appraisal of Medicines: A Systematic Approach to
Decision-making. Wiley-Blackwell.
Gao Q, Gao W, Xia Q, Xie C, Ma J, Xie L. (2019). Ef-
fectiveness of therapeutic strategies for patients with
neck pain: Protocol for a systematic review and net-
work meta-analysis. Medicine, 98(11):e14526.
Giles, T. (2006). Circadian rhythm of blood pressure and the
relation to cardiovascular events. Journal of hyperten-
sion. Supplement : official journal of the International
Society of Hypertension, 24:S11–6.
Graham, I. and AL. (2007). European guidelines on cardio-
vascular disease prevention in clinical practice: exec-
utive summary. European Heart Journal.
Gueyffier, F., Subtil, F., Bejan-Angoulvant, T., Baguet, J.,
Boivin, J., Mercier, A., Leftheriotis, G., Gagnol, J.,
Fauvel, J., Giraud, C., Bricca, G., Maucort-Boulch,
D., and Erpeldinger, S. (2015). Can we identify re-
sponse markers to antihypertensive drugs? first results
from the ideal trial. Journal of Human Hypertension,
pages 7–22.
Gumel, A., Zhang, X.-W., Shivakumar, P., Garba, M., and
Sahai, B. (2002). A new mathematical model for as-
sessing therapeutic strategies for hiv infection. Jour-
nal of Theoretical Medicine, 4.
Holford, N. H. and Sheiner, L. B. (1982). Kinetics of phar-
macologic response. Pharmacology & Therapeutics,
16(2):143–166.
Isern, D., S
´
anchez, D., and Moreno, A. (2007). Hecase2: a
multi-agent ontology-driven guideline enactment en-
gine. In CEEMAS 2007, volume 4696, pages 322–
324, Leipzig, Germany. Springer Verlag.
Ivanny, M. (2018). Optimization of sudden cardiac death
prevention in type 2 diabetes in France : a public
health simulation study on a realistic virtual popula-
tion. PhD thesis, UCB Lyon 1.
Kotecha, R. R., Hsu, D. J., Lee, C.-H., Patil, S., and Voss,
M. H. (2021). In silico modeling of combination sys-
temic therapy for advanced renal cell carcinoma. Jour-
nal for ImmunoTherapy of Cancer, 9(12).
Nayyar, A., Gadhavi, L., and Zaman, N. (2021). Chapter 2 -
machine learning in healthcare: review, opportunities
and challenges. In Singh, K. K., Elhoseny, M., Singh,
A., and Elngar, A. A., editors, Machine Learning and
the Internet of Medical Things in Healthcare, pages
23–45. Academic Press.
Osude, N., Durazo-Arvizu, R., Markossian, T., Liu, K., Mi-
chos, E. D., Rakotz, M., Wozniak, G., Egan, B., and
Kramer, H. (2021). Age and sex disparities in hyper-
tension control: The multi-ethnic study of atheroscle-
rosis (mesa). American Journal of Preventive Cardi-
ology, 8:100230.
Patel, K., Dodds, M., Gonc¸alves, A., Kamal, M. A., Rayner,
C. R., Kirkpatrick, C. M., and Smith, P. F. (2021).
Using in silico viral kinetic models to guide thera-
peutic strategies during a pandemic: An example in
sars-cov-2. British Journal of Clinical Pharmacology,
87(9):3425–3438.
Phan, A.-C., Phan, T.-C., and Trieu, T.-N. (2022). A sys-
tematic approach to healthcare knowledge manage-
ment systems in the era of big data and artificial in-
telligence. Applied Sciences, 12(9).
Richard J. Chen, Ming Y. Lu, T. Y. C. D. F. K. W. .
F. M. (2021). Synthetic data in machine learning for
medicine and healthcare. Nature Biomedical Engi-
neering, 5:493–497.
Roth, G. A. and al. (2020). Global burden of cardiovascular
diseases and risk factors, 1990–2019: Update from the
gbd 2019 study. Journal of the American College of
Cardiology, 76(25):2982–3021.
Sackett, D. L., Rosenberg, W. M. C., Gray, J. A. M.,
Haynes, R. B., and Richardson, W. S. (1996). Ev-
idence based medicine: what it is and what it isn’t.
BMJ, 312(7023):71–72.
Schoepflin, D., Holst, D., Gomse, M., and Sch
¨
uppstuhl,
T. (2021). Synthetic training data generation for vi-
sual object identification on load carriers. Procedia
CIRP, 104:1257–1262. 54th CIRP CMS 2021 - To-
wards Digitalized Manufacturing 4.0.
Schreiber, G., Akkermans, H., Anjewierden, A., de Hoog,
R., Shadbolt, N. R., Van de Velde, W., and Wielinga,
B. J. (1999). Knowledge Engineering and Manage-
ment: The CommonKADS Methodology. The MIT
Press.
Sheridan, D. J. and Julian, D. G. (2016). Achievements and
limitations of evidence-based medicine. Journal of the
American College of Cardiology, 68(2):204–213.
Shortliffe, E. (1976). Computer-Based Medical Consulta-
tions: MYCIN. Elsevier/North Holland, New York.
T., M. and A., R. (2002). Resource implications and health
benefits of primary prevention strategies for cardio-
A Knowledge-Based Approach for Evaluating Impact of Therapeutic Strategies
1045