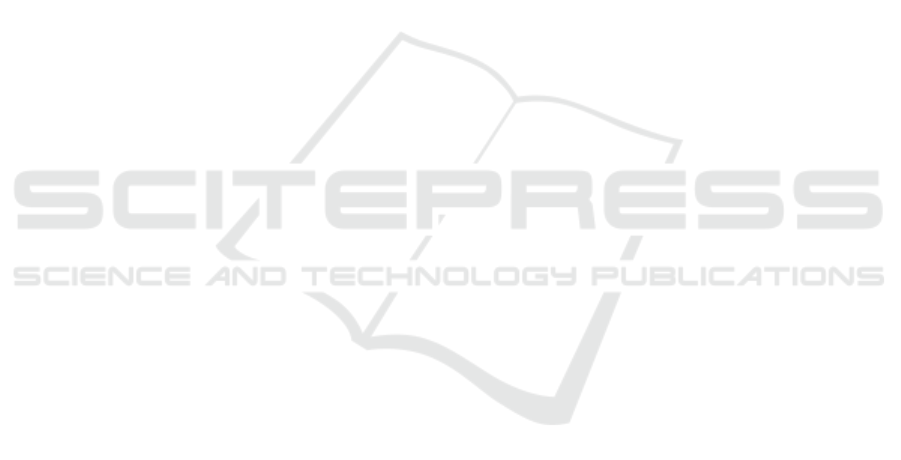
feedback during the learning phase to identify useful
prototypes as a potential next step. This could also
strengthen the need to demonstrate and validate which
properties are actually required for interpretability
and for effective internal assessment of models. As
observed already, given relevant prototypes, OOD de-
tection could largely benefit from interpretable proto-
types which calls for finding better techniques, partic-
ularly in ‘Near OOD’ regime.
REFERENCES
Adadi, A. and Berrada, M. (2018). Peeking Inside the
Black-Box: A Survey on Explainable Artificial Intel-
ligence (XAI). IEEE Access, 6:52138–52160.
Bach, S., Binder, A., Montavon, G., Klauschen, F., M
¨
uller,
K.-R., and Samek, W. (2015). On Pixel-Wise Ex-
planations for Non-Linear Classifier Decisions by
Layer-Wise Relevance Propagation. PLOS ONE,
10(7):e0130140.
Chen, C., Li, O., Tao, D., Barnett, A., Rudin, C., and Su,
J. K. (2019). This Looks Like That: Deep Learning for
Interpretable Image Recognition. In Proc. NeurIPS,
volume 32. Curran Associates, Inc.
Fang, Z., Kuang, K., Lin, Y., Wu, F., and Yao, Y.-F. (2020).
Concept-based Explanation for Fine-grained Images
and Its Application in Infectious Keratitis Classifica-
tion. In Proc. ACM Multimedia. Association for Com-
puting Machinery.
Gautam, S., H
¨
ohne, M. M.-C., Hansen, S., Jenssen, R.,
and Kampffmeyer, M. (2021). This looks more like
that: Enhancing Self-Explaining Models by Prototyp-
ical Relevance Propagation. arXiv:2108.12204.
Hendrycks, D., Mazeika, M., Kadavath, S., and Song, D.
(2019). Using self-supervised learning can improve
model robustness and uncertainty. arXiv preprint
arXiv:1906.12340.
Johnson, J., Hariharan, B., van der Maaten, L., Fei-Fei, L.,
Lawrence Zitnick, C., and Girshick, R. (2017). Clevr:
A diagnostic dataset for compositional language and
elementary visual reasoning. In Proceedings of the
IEEE Conference on Computer Vision and Pattern
Recognition (CVPR).
Koh, P. W., Nguyen, T., Tang, Y. S., Mussmann, S., Pierson,
E., Kim, B., and Liang, P. (2020). Concept Bottleneck
Models. arXiv:2007.04612.
Li, O., Liu, H., Chen, C., and Rudin, C. (2018). Deep Learn-
ing for Case-Based Reasoning Through Prototypes: A
Neural Network That Explains Its Predictions. Proc.
AAAI, 32(1).
Nauta, M., van Bree, R., and Seifert, C. (2021). Neural
Prototype Trees for Interpretable Fine-grained Image
Recognition. In Proc. CVPR, pages 14928–14938,
Nashville, TN, USA. IEEE.
Netzer, Y., Wang, T., Coates, A., Bissacco, A., Wu, B., and
Ng, A. Y. (2011). Reading digits in natural images
with unsupervised feature learning.
Nguyen, A., Yosinski, J., and Clune, J. (2019). Under-
standing Neural Networks via Feature Visualization:
A Survey. In Explainable AI: Interpreting, Explain-
ing and Visualizing Deep Learning, Lecture Notes
in Computer Science, pages 55–76. Springer Interna-
tional Publishing, Cham.
P
´
aez, A. (2019). The pragmatic turn in explainable artificial
intelligence (XAI). Minds and Machines, 29(3):441–
459.
Rudin, C. (2019). Stop explaining black box machine learn-
ing models for high stakes decisions and use inter-
pretable models instead. Nature Machine Intelligence,
1(5):206–215.
Shrikumar, A., Greenside, P., and Kundaje, A. (2017).
Learning important features through propagating ac-
tivation differences. In Precup, D. and Teh, Y. W.,
editors, Proceedings of the 34th International Con-
ference on Machine Learning, volume 70 of Pro-
ceedings of Machine Learning Research, pages 3145–
3153. PMLR.
Stammer, W., Memmel, M., Schramowski, P., and Kerst-
ing, K. (2022). Interactive Disentanglement: Learn-
ing Concepts by Interacting with their Prototype Rep-
resentations. arXiv:2112.02290.
Sundararajan, M., Taly, A., and Yan, Q. (2017). Axiomatic
Attribution for Deep Networks. In Proc. ICML, pages
3319–3328. PMLR.
Tjoa, E. and Guan, C. (2020). A Survey on Explainable
Artificial Intelligence (XAI): Towards Medical XAI.
arXiv:1907.07374.
Wah, C., Branson, S., Welinder, P., Perona, P., and Be-
longie, S. (2011). The caltech-ucsd birds-200-2011
dataset.
Zhang, Q., Wu, Y. N., and Zhu, S.-C. (2018). Interpretable
Convolutional Neural Networks. In Proc. CVPR,
pages 8827–8836.
Zhang, Q.-s. and Zhu, S.-c. (2018). Visual interpretability
for deep learning: A survey. Frontiers Inf Technol
Electronic Eng, 19(1):27–39.
Zhou, B., Khosla, A., Lapedriza, A., Oliva, A., and Tor-
ralba, A. (2016). Learning Deep Features for Dis-
criminative Localization. In Proc. CVPR, pages 2921–
2929.
Towards Human-Interpretable Prototypes for Visual Assessment of Image Classification Models
887