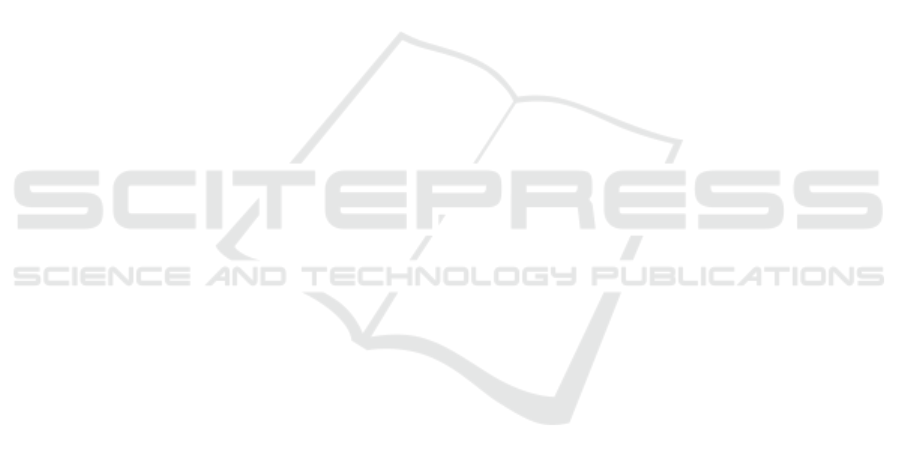
Hacker, W. (2018). Menschengerechtes Arbeiten in der digi-
talisierten Welt: Eine Wissenschaftliche Handreichung,
volume Band 49 of Mensch - Technik - Organisation.
vdf Hochschulverlag AG an der ETH Zürich, Zürich,
1. auflage edition.
Hamzeh, R., Zhong, R., Xu, X. W., Kajati, E., and Zolotova,
I. (2018). A Technology Selection Framework for Man-
ufacturing Companies in the Context of Industry 4.0.
In 2018 World Symposium on Digital Intelligence for
Systems and Machines (DISA), pages 267–276. IEEE.
Hattingh, M., Marshall, L., Holmner, M., and Naidoo, R.
(2019). Data Science Competency in Organisations. In
de Villiers, C. and Smuts, H., editors, Proceedings of
the South African Institute of Computer Scientists and
Information Technologists 2019 on ZZZ - SAICSIT ’19,
pages 1–8, New York, New York, USA. ACM Press.
Hesenius, M., Schwenzfeier, N., Meyer, O., Koop, W., and
Gruhn, V. (2019). Towards a Software Engineering
Process for Developing Data-Driven Applications. In
2019 IEEE/ACM 7th International Workshop on Re-
alizing Artificial Intelligence Synergies in Software
Engineering (RAISE), pages 35–41. IEEE.
Hou, Z. and Pan, C. (2018). Data Mining Method and Em-
pirical Research for Extension Architecture Design. In
2018 International Conference on Intelligent Trans-
portation, Big Data & Smart City (ICITBS), pages
275–278. IEEE.
IBM Corporation (2016). Analytics Solutions Unified
Method: Implementations with Agile principles.
IEEE Computer Society/Software & Systems Engineering
Standards Committee (2019). Software, systems and
enterprise — Architecture processes: International
Standard: ISO/IEC/IEEE 42020:2019.
ISO, IEC, and IEEE (2018). ISO/IEC/IEEE 29148:2018(E):
ISO/IEC/IEEE International Standard - Systems and
software engineering – Life cycle processes – Require-
ments engineering.
Kienzler, R. (2019a). Architectural decisions guidelines: An
architectural decisions guide for data science.
Kienzler, R. (2019b). The lightweight IBM Cloud Garage
Method for data science: A process model to map
individual technology components to the reference ar-
chitecture.
Konstan, J. A. and Adomavicius, G. (2013). Toward identifi-
cation and adoption of best practices in algorithmic rec-
ommender systems research. In Bellogín, A., Catells,
P., Said, A., and Tikk, D., editors, Proceedings of the
International Workshop on Reproducibility and Repli-
cation in Recommender Systems Evaluation - RepSys
’13, pages 23–28, New York, New York, USA. ACM
Press.
Kutzias, D., Dukino, C., and Kett, H. (2021). Towards a
Continuous Process Model for Data Science Projects.
In Leitner, C., Ganz, W., Satterfield, D., and Bassano,
C., editors, Advances in the Human Side of Service
Engineering, volume 266 of Lecture Notes in Networks
and Systems, pages 204–210. Springer International
Publishing, Cham.
Kutzias, D., Falkner, J., and Kett, H. (2019). On the Com-
plexity of Cloud and IoT Integration: Architectures,
Challenges and Solution Approaches. In Proceed-
ings of the 4th International Conference on Internet
of Things, Big Data and Security, pages 376–384.
SCITEPRESS - Science and Technology Publications.
Link, M., Dukino, C., Ganz, W., Hamann, K., and Schnalzer,
K. (2020). The Use of AI-Based Assistance Systems
in the Service Sector: Opportunities, Challenges and
Applications. In Nunes, I. L., editor, Advances in
Human Factors and Systems Interaction, volume 1207
of Advances in Intelligent Systems and Computing,
pages 10–16. Springer International Publishing, Cham.
Mariscal, G., Marbán, Ó., and Fernández, C. (2010). A sur-
vey of data mining and knowledge discovery process
models and methodologies. The Knowledge Engineer-
ing Review, 25(2):137–166.
Martinez-Plumed, F., Contreras-Ochando, L., Ferri, C., Her-
nandez Orallo, J., Kull, M., Lachiche, N., Ramirez
Quintana, M. J., and Flach, P. A. (2020). CRISP-
DM Twenty Years Later: From Data Mining Processes
to Data Science Trajectories. IEEE Transactions on
Knowledge and Data Engineering, page 1.
Mayring, P. (2019). Qualitative Content Analysis: Demar-
cation, Varieties, Developments. Forum: Qualitative
Social Research, 20(3).
Microsoft (2020). Team Data Science Process Documenta-
tion.
Min, H. (1991). Selection of Software: The Analytic Hi-
erarchy Process. International Journal of Physical
Distribution & Logistics Management,, 1991(22):42–
52.
Nguyen, A., Yosinski, J., and Clune, J. (2014). Deep Neural
Networks are Easily Fooled: High Confidence Predic-
tions for Unrecognizable Images.
Oxenham, S. (2016). Legal maze threatens to slow data
science. nature, 2016(536):16–17.
Paleyes, A., Urma, R., and Lawrence, N. D. (2020). Chal-
lenges in deploying machine learning: a survey of case
studies. CoRR, abs/2011.09926.
Partnership on AI (2018). AI, Labor, and Economy Case
Studies: Compendium Synthesis.
Pedersen, T. B. (2007). Warehousing The World – A Few
Remaining Challenges. ACM, New York, NY.
Pérez, M. S., Sánchez, A., Robles, V., Herrero, P., and Peña,
J. M. (2007). Design and implementation of a data
mining grid-aware architecture. Future Generation
Computer Systems, 23(1):42–47.
Ramesh, B. (2015). Big Data Architecture. In Mohanty, H.,
Bhuyan, P., and Chenthati, D., editors, Big Data, vol-
ume 11 of Studies in Big Data, pages 29–59. Springer
India, New Delhi.
Randell, B. (1979). Software engineering in 1968. Comput-
ing Laboratory Technical Report Series.
Reggio, G. and Astesiano, E. (2020). Big-data/analytics
projects failure: A literature review. In 2020 46th
Euromicro Conference on Software Engineering and
Advanced Applications (SEAA), pages 246–255.
Rindfleisch, A. (2020). The Second Digital Revolution.
Marketing Letters, 31(1):13–17.
Rudolph, E., Schönfelder, E., and Hacker, W. (1987).
Tätigkeitsbewertungssystem - Geistige Arbeit.
Comparative Analysis of Process Models for Data Science Projects
1061