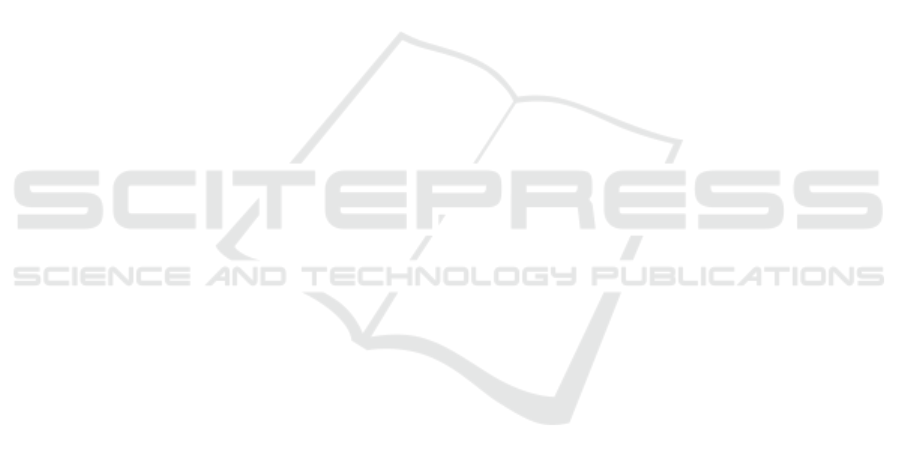
dings for retrieval from a large knowledge base. arXiv
preprint arXiv:1810.10176.
Cakaloglu, T. and Xu, X. (2019). A multi-resolution word
embedding for document retrieval from large unstruc-
tured knowledge bases. arXiv preprint.
Cakaloglu, T., Xu, X., and Raghavan, R. (2022). Emboost:
Embedding boosting to learn multilevel abstract text
representation for document retrieval. In Rocha, A. P.,
Steels, L., and van den Herik, H. J., editors, Proceed-
ings of the 14th International Conference on Agents
and Artificial Intelligence, ICAART 2022, Volume 3,
Online Streaming, February 3-5, 2022, pages 352–360.
SCITEPRESS.
Callan, J., Hoy, M., Yoo, C., and Zhao, L. (2009). Clueweb09
data set.
Chen, D., Fisch, A., Weston, J., and Bordes, A. (2017a).
Reading wikipedia to answer open-domain questions.
In ACL.
Chen, Q., Hu, Q., Huang, X., He, L., and An, W. (2017b).
Enhancing recurrent neural networks with positional
attention for question answering. In SIGIR.
Conneau, A., Kiela, D., Schwenk, H., Barrault, L., and Bor-
des, A. (2017). Supervised learning of universal sen-
tence representations from natural language inference
data. In EMNLP.
Devlin, J., Chang, M.-W., Lee, K., and Toutanova, K.
(2018). Bert: Pre-training of deep bidirectional trans-
formers for language understanding. arXiv preprint
arXiv:1810.04805.
Dhingra, B., Mazaitis, K., and Cohen, W. W. (2017). Quasar:
Datasets for question answering by search and reading.
arXiv preprint arXiv:1707.03904.
dos Santos, C. N., Tan, M., Xiang, B., and Zhou, B. (2016).
Attentive pooling networks. CoRR, abs/1602.03609.
Guo, J., Fan, Y., Ai, Q., and Croft, W. B. (2016). A deep rel-
evance matching model for ad-hoc retrieval. In CIKM.
He, K., Zhang, X., Ren, S., and Sun, J. (2015). Delving deep
into rectifiers: Surpassing human-level performance
on imagenet classification. In 2015 IEEE International
Conference on Computer Vision (ICCV), pages 1026–
1034.
Heilman, M. and Smith, N. A. (2010). Tree edit models
for recognizing textual entailments, paraphrases, and
answers to questions. In HLT-NAACL.
Htut, P. M., Bowman, S. R., and Cho, K. (2018). Training a
ranking function for open-domain question answering.
In NAACL-HLT.
Hu, B., Lu, Z., Li, H., and Chen, Q. (2014). Convolutional
neural network architectures for matching natural lan-
guage sentences. In NIPS.
Huang, G., Liu, Z., v. d. Maaten, L., and Weinberger, K. Q.
(2017). Densely connected convolutional networks.
In 2017 IEEE Conference on Computer Vision and
Pattern Recognition (CVPR), pages 2261–2269.
Hui, K., Yates, A., Berberich, K., and de Melo, G. (2017).
Pacrr: A position-aware neural ir model for relevance
matching. In EMNLP.
Ioffe, S. and Szegedy, C. (2015). Batch normalization: Ac-
celerating deep network training by reducing internal
covariate shift. In Proceedings of the 32Nd Interna-
tional Conference on International Conference on Ma-
chine Learning - Volume 37, ICML’15, pages 448–456.
JMLR.org.
Kim, S., Hong, J.-H., Kang, I., and Kwak, N. (2018).
Semantic sentence matching with densely-connected
recurrent and co-attentive information. CoRR,
abs/1805.11360.
Kingma, D. P. and Ba, J. (2014). Adam: A
method for stochastic optimization. arXiv preprint
arXiv:1412.6980.
Le, Q. V. and Mikolov, T. (2014). Distributed representations
of sentences and documents. In ICML.
Lu, Z. and Li, H. (2013). A deep architecture for matching
short texts. In NIPS 2013.
Manning, C. D., Raghavan, P., and Sch
¨
utze, H. (2008). In-
troduction to Information Retrieval. Cambridge Uni-
versity Press.
McDonald, R., Ding, Y., and Androutsopoulos, I. (2018).
Deep relevance ranking using enhanced document-
query interactions. In EMNLP.
Mikolov, T., Grave, E., Bojanowski, P., Puhrsch, C., and
Joulin, A. (2018). Advances in pre-training distributed
word representations. In Proceedings of the Interna-
tional Conference on Language Resources and Evalua-
tion (LREC 2018).
Palangi, H., Deng, L., Shen, Y., Gao, J., He, X., Chen,
J., Song, X., and Ward, R. K. (2016). Deep sen-
tence embedding using long short-term memory net-
works: Analysis and application to information re-
trieval. IEEE/ACM Transactions on Audio, Speech,
and Language Processing, 24:694–707.
Peters, M., Neumann, M., Iyyer, M., Gardner, M., Clark,
C., Lee, K., and Zettlemoyer, L. (2018). Deep con-
textualized word representations. In Proceedings of
the 2018 Conference of the North American Chapter
of the Association for Computational Linguistics: Hu-
man Language Technologies, Volume 1 (Long Papers),
pages 2227–2237.
Rajpurkar, P., Zhang, J., Lopyrev, K., and Liang, P. (2016).
Squad: 100, 000+ questions for machine comprehen-
sion of text. arXiv preprint arXiv:1606.05250.
Rao, J., He, H., and Lin, J. J. (2016). Noise-contrastive esti-
mation for answer selection with deep neural networks.
In CIKM.
Robertson, S. and Zaragoza, H. (2009). The probabilistic
relevance framework: Bm25 and beyond. Foundations
and Trends in Information Retrieval, 3:333–389.
Salton, G. and McGill, M. J. (1986). Introduction to modern
information retrieval.
Santoro, A., Raposo, D., Barrett, D. G. T., Malinowski,
M., Pascanu, R., Battaglia, P. W., and Lillicrap, T. P.
(2017). A simple neural network module for relational
reasoning. In NIPS.
Schroff, F., Kalenichenko, D., and Philbin, J. (2015).
Facenet: A unified embedding for face recognition and
clustering. 2015 IEEE Conference on Computer Vision
and Pattern Recognition (CVPR), pages 815–823.
ICAART 2023 - 15th International Conference on Agents and Artificial Intelligence
476