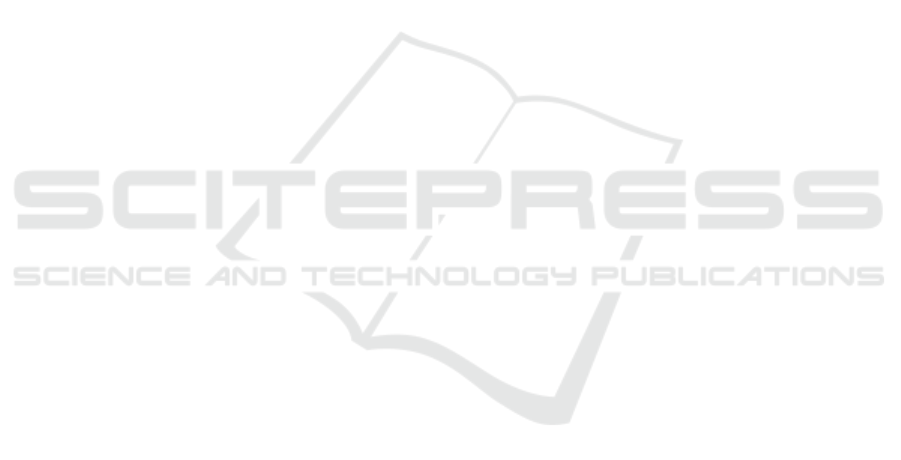
ACKNOWLEDGEMENTS
This research is supported by the Beneva Insur-
ance and Natural Sciences and Engineering Research
Council of Canada (NSERC).
REFERENCES
Abowd, J. M. (2018). Protecting the confidentiality of amer-
ica’s statistics: Adopting modern disclosure avoid-
ance methods at the census bureau. Census Blogs:
Research Matters.
Adams, A. (1999). The implications of users’ multimedia
privacy perceptions on communication and informa-
tion privacy policies. In Proceedings of Telecommuni-
cations Policy Research Conference, pages 1–23.
Azencott, C.-A. (2018). Machine learning and ge-
nomics: precision medicine versus patient privacy.
Philosophical Transactions of the Royal Society A:
Mathematical, Physical and Engineering Sciences,
376(2128):20170350.
Berger, B. and Cho, H. (2019). Emerging technologies to-
wards enhancing privacy in genomic data sharing.
Chang, K. C., Zaeem, R. N., and Barber, K. S. (2021). An
identity asset sensitivity model in self-sovereign iden-
tities.
Chen, K.-C., Yu, C.-M., Tai, B.-C., Li, S.-C., Tsou, Y.-T.,
Huang, Y., and Lin, C.-M. (2017). Data-driven ap-
proach for evaluating risk of disclosure and utility in
differentially private data release. In 2017 IEEE 31st
International Conference on Advanced Information
Networking and Applications (AINA), pages 1130–
1137. IEEE.
Dankar, F. K. and Badji, R. (2017). A risk-based framework
for biomedical data sharing. Journal of Biomedical
Informatics, 66:231–240.
Dwork, C. and Lei, J. (2009). Differential privacy and ro-
bust statistics. In Proceedings of the forty-first annual
ACM symposium on Theory of computing, pages 371–
380.
Dwork, C., McSherry, F., Nissim, K., and Smith, A. (2006).
Calibrating noise to sensitivity in private data anal-
ysis. Lecture Notes in Computer Science (including
subseries Lecture Notes in Artificial Intelligence and
Lecture Notes in Bioinformatics), 3876 LNCS:265–
284.
Dwork, C. and Roth, A. (2014). The algorithmic foun-
dations of differential privacy. Found. Trends Theor.
Comput. Sci., 9(3-4):211–407.
Dwork, C. and Smith, A. (2010). Differential privacy for
statistics: What we know and what we want to learn.
Journal of Privacy and Confidentiality, 1(2).
Eltoft, T., Kim, T., and Lee, T.-W. (2006). On the multi-
variate laplace distribution. IEEE Signal Processing
Letters, 13(5):300–303.
Gibbs, S. (2018). https://www.theguardian.com/
technology.
Goldman, E. (2020). An introduction to the california con-
sumer privacy act (ccpa). Santa Clara Univ. Legal
Studies Research Paper.
GoogleDP, R. (2018). https://github.com/google/
differential-privacy.
Greenberg, A. (2016). Apple’s’ differential privacy’is
about collecting your data—but not your data.(2016).
URL www. wired. com/2016/06/apples-differential-
privacy-collecting-data.
Hawes, M. (2020). Differential privacy and the 2020 de-
cennial census. In APHA’s 2020 VIRTUAL Annual
Meeting and Expo (Oct. 24-28). APHA.
Hayes, J., Balle, B., and Kumar, M. P. (2022). Learning
to be adversarially robust and differentially private.
arXiv preprint arXiv:2201.02265.
Jiang, Y., Wang, C., Wu, Z., Du, X., and Wang, S. (2018).
Privacy-preserving biomedical data dissemination via
a hybrid approach. In AMIA Annual Symposium Pro-
ceedings, volume 2018, page 1176. American Medi-
cal Informatics Association.
Johnson, N., Near, J. P., and Song, D. (2018). Towards prac-
tical differential privacy for sql queries. Proceedings
of the VLDB Endowment, 11(5):526–539.
Kim, J. W., Jang, B., and Yoo, H. (2018). Privacy-
preserving aggregation of personal health data
streams. PloS one, 13(11):e0207639.
Lee, J. and Clifton, C. (2011). How much is enough? choos-
ing epsilon for differential privacy. pages 325–340.
Liau, D., Zaeem, R. N., and Barber, K. S. (2019). Eval-
uation framework for future privacy protection sys-
tems: A dynamic identity ecosystem approach. In
2019 17th International Conference on Privacy, Se-
curity and Trust (PST), pages 1–3.
McClure, D. and Reiter, J. P. (2012). Differential privacy
and statistical disclosure risk measures: An investi-
gation with binary synthetic data. Trans. Data Priv.,
5(3):535–552.
Naldi, M. and D’Acquisto, G. (2015). Differential privacy:
An estimation theory-based method for choosing ep-
silon. arXiv preprint arXiv:1510.00917.
Quinton, S. and Reynolds, N. (2018). Characteristics of
digital data.
Regulation, G. D. P. (2018). General data protection regula-
tion (gdpr). Intersoft Consulting, Accessed in October,
24(1).
Subramanian, R. (2022). Applications of differential pri-
vacy to healthcare. Available at SSRN 4005908.
Tsou, Y.-T., Chen, H.-L., and Chang, Y.-H. (2019). Rod:
Evaluating the risk of data disclosure using noise esti-
mation for differential privacy. IEEE Transactions on
Big Data.
Winslett, M., Yang, Y., and Zhang, Z. (2012). Demonstra-
tion of damson: Differential privacy for analysis of
large data. In 2012 IEEE 18th International Confer-
ence on Parallel and Distributed Systems, pages 840–
844. IEEE.
Zaeem, R. N., Budalakoti, S., Barber, K. S., Rasheed, M.,
and Bajaj, C. (2016). Predicting and explaining iden-
tity risk, exposure and cost using the ecosystem of
identity attributes. pages 1–8.
Differential Privacy: Toward a Better Tuning of the Privacy Budget () Based on Risk
791