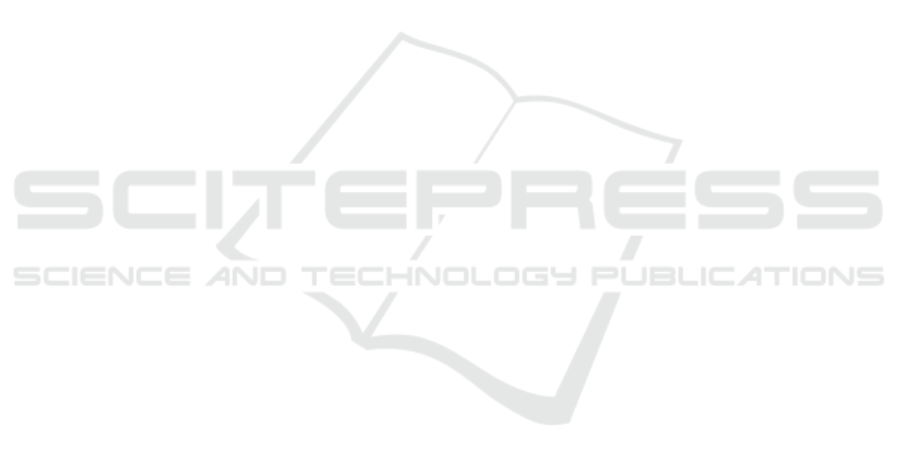
Chawla, N. V., Japkowicz, N., and Kotcz, A. (2004). Edi-
torial: Special issue on learning from imbalanced data
sets. SIGKDD Explor. Newsl., 6(1):1–6.
Chen, T. and Guestrin, C. (2016). XGBoost: A Scalable
Tree Boosting System. In Proceedings of the 22nd
ACM SIGKDD Int. Conf. on Knowledge Discovery
and Data Mining, pages 785–794, New York, NY,
USA. ACM.
Das, M. (2021). WHO launches strategy to accelerate
elimination of cervical cancer. The Lancet Oncology,
22(1):20–21.
Diniz, D. N., Rezende, M. T., Bianchi, A. G., Carneiro,
C. M., Luz, E. J., Moreira, G. J., Ushizima, D. M.,
de Medeiros, F. N., and Souza, M. J. (2021a). A deep
learning ensemble method to assist cytopathologists
in pap test image classification. Journal of Imaging,
7(7).
Diniz, D. N., Rezende, M. T., Bianchi, A. G. C., Carneiro,
C. M., Ushizima, D. M., de Medeiros, F. N. S., and
Souza, M. J. F. (2021b). A Hierarchical Feature-Based
Methodology to Perform Cervical Cancer Classifica-
tion. Applied Sciences, 11(9):2–19.
Diniz, D. N., Vitor, R. F., Bianchi, A. G. C., Delabrida,
S., Carneiro, C. M., Ushizima, D. M., de Medeiros,
F. N. S., and Souza, M. J. F. (2021c). An ensemble
method for nuclei detection of overlapping cervical
cells. Expert Systems with Applications, 185:115642.
Dong, N., Zhao, L., Wu, C., and Chang, J. (2020). Inception
v3 based cervical cell classification combined with ar-
tificially extracted features. Applied Soft Computing,
93:106311.
Geron, A. (2022). Hands-on machine learning with Scikit-
Learn, Keras, and TensorFlow. O’Reilly Media, Inc.
Han, H., Wang, W.-Y., and Mao, B.-H. (2005). Borderline-
SMOTE: a new over-sampling method in imbalanced
data sets learning. In Int. conf. on intelligent comput-
ing, pages 878–887. Springer.
Jantzen, J., Norup, J., Dounias, G., and Bjerregaard, B.
(2005). Pap-smear Benchmark Data For Pattern Clas-
sification. Proc. NiSIS 2005, Albufeira, Portugal,
pages 1–9.
Jiang, H., Zhou, Y., Lin, Y., Chan, R. C., Liu, J., and Chen,
H. (2022). Deep learning for computational cytology:
A survey. Medical Image Analysis, page 102691.
Konatar, I., Popovic, T., and Popovic, N. (2020). Box-
Counting Method in Python for Fractal Analysis of
Biomedical Images. 2020 24th Int. Conf. on Informa-
tion Technology, IT 2020, (February).
Kuhl, F. P. and Giardina, C. R. (1982). Elliptic fourier fea-
tures of a closed contour. Computer Graphics and Im-
age Processing, 18(3):236–258.
Li, X., Xu, Z., Shen, X., Zhou, Y., Xiao, B., and Li, T.-
Q. (2021). Detection of Cervical Cancer Cells in
Whole Slide Images Using Deformable and Global
Context Aware Faster RCNN-FPN. Current Oncol-
ogy, 28(5):3585–3601.
Mariarputham, E. J. and Stephen, A. (2015). Nominated
Texture Based Cervical Cancer Classification. Com-
putational and Mathematical Methods in Medicine,
2015:1–10.
Marinakis, Y., Dounias, G., and Jantzen, J. (2009). Pap
smear diagnosis using a hybrid intelligent scheme fo-
cusing on genetic algorithm based feature selection
and nearest neighbor classification. Computers in Bi-
ology and Medicine, 39(1):69–78.
Nayar, R. and Wilbur, D. C. (2015). The bethesda system for
reporting cervical cytology: Definitions, criteria, and
explanatory notes. Springer International Publishing.
Po-Hsiang Tsui, Yin-Yin Liao, Chien-Cheng Chang, Wen-
Hung Kuo, King-Jen Chang, and Chih-Kuang Yeh
(2010). Classification of Benign and Malignant Breast
Tumors by 2-D Analysis Based on Contour Descrip-
tion and Scatterer Characterization. IEEE Trans. on
Med. Imaging, 29(2):513–522.
Rahaman, M. M., Li, C., Yao, Y., Kulwa, F., Wu, X., Li, X.,
and Wang, Q. (2021). DeepCervix: A deep learning-
based framework for the classification of cervical cells
using hybrid deep feature fusion techniques. Comput-
ers in Biology and Medicine, 136:104649.
Rezende, M. T., Silva, R., Bernardo, F. d. O., Tobias,
A. H., Oliveira, P. H., Machado, T. M., Costa, C. S.,
Medeiros, F. N., Ushizima, D. M., Carneiro, C. M.,
and Bianchi, A. G. (2021). Cric searchable image
database as a public platform for conventional pap
smear cytology data. Scientific Data, 8(1):151.
Sung, H., Ferlay, J., Siegel, R. L., Laversanne, M., Soerjo-
mataram, I., Jemal, A., and Bray, F. (2021). Global
cancer statistics 2020: Globocan estimates of in-
cidence and mortality worldwide for 36 cancers in
185 countries. CA: a cancer journal for clinicians,
71(3):209–249.
Teixeira, J. B. A., Rezende, M. T., Diniz, D. N., Carneiro,
C. M., Luz, E. J. d. S., Souza, M. J. F., Ushizima,
D. M., de Medeiros, F. N. S., and Bianchi, A. G. C.
(2022). Segmentac¸
˜
ao autom
´
atica de n
´
ucleos cervicais
em imagens de Papanicolaou. In Anais do XXII Simp.
Bras. de Computac¸
˜
ao Aplicada
`
a Sa
´
ude, pages 346–
357. Soc. Bras. de Computac¸
˜
ao.
Umadi, A., Nagarajan, K., Venkatesha, J. B., Ganesh, A.,
and George, K. (2020). Automated Segmentation of
Overlapping Cells in Cervical Cytology Images Using
Deep Learning. In 2020 IEEE 17th India Council Int.
Conf.e, INDICON 2020, pages 1–7. IEEE.
Williams, A. (2021). Cervical cancer: what’s new in
squamous cell neoplasia. Diagnostic Histopathology,
27(12):478–482.
Yakkundimath, R., Jadhav, V., Anami, B., and Malvade,
N. (2022). Co-occurrence histogram based ensem-
ble of classifiers for classification of cervical cancer
cells. Journal of Electronic Science and Technology,
20(3):100170.
Zhang, L., Kong, H., Ting Chin, C., Liu, S., Fan, X., Wang,
T., and Chen, S. (2014). Automation-assisted cervi-
cal cancer screening in manual liquid-based cytology
with hematoxylin and eosin staining. Cytometry Part
A, 85(3):214–230.
Zhao, Y., Fu, C., Xu, S., Cao, L., and Ma, H.-f.
(2022). LFANet: Lightweight feature attention net-
work for abnormal cell segmentation in cervical cy-
tology images. Computers in Biology and Medicine,
145:105500.
Shape-based Features Investigation for Preneoplastic Lesions on Cervical Cancer Diagnosis
513