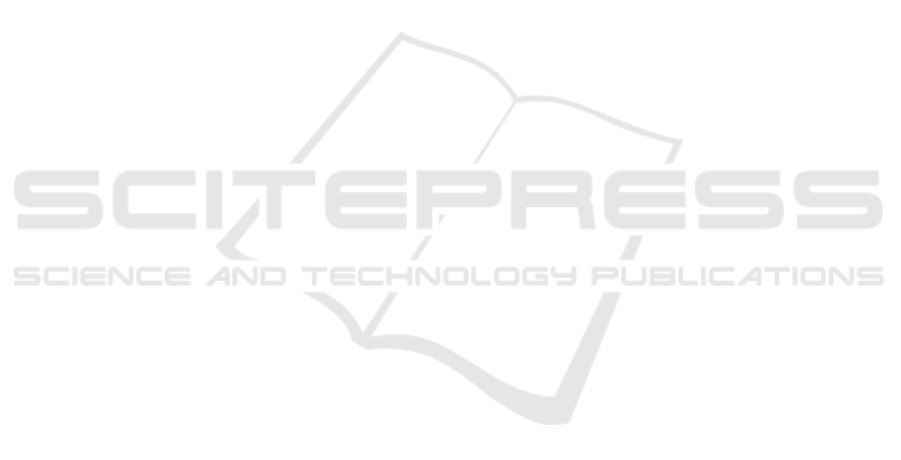
can significantly improve the DiMP tracker’s robust-
ness against illumination variation, background clut-
ters, camera motion, and occlusion. Extensive test-
ing on the VOT2018, UAV123, OTB100, and LaSOT
benchmarks demonstrate that our technique provides
state-of-the-art outcomes. In future work, we will ex-
tend our network by using the new fusions module
and adding a mask branch prediction to boost the per-
formance of trackers and address the challenges of
fast motion, scale variation, and similar objects.
ACKNOWLEDGEMENTS
This research has been done under the research
project TXTCN.22.02 of Vietnam National Univer-
sity, Hanoi.
REFERENCES
Bhat, G., Danelljan, M., Gool, L. V., and Timofte, R.
(2019). Learning discriminative model prediction for
tracking. In ICCV.
Bhat, G., Danelljan, M., Gool, L. V., and Timofte, R.
(2020). Know your surroundings: Exploiting scene
information for object tracking. In ECCV.
Chen, X., Yan, B., Zhu, J., Wang, D., Yang, X., and Lu, H.
(2021). Transformer tracking. In CVPR.
Chen, Z., Zhong, B., Li, G., Zhang, S., and Ji, R. (2020).
Siamese box adaptive network for visual tracking. In
CVPR.
Cheng, S., Zhong, B., Li, G., Liu, X., Tang, Z., Li, X., and
Wang, J. (2021). Learning to filter: Siamese relation
network for robust tracking. In CVPR.
Cui, Y., Jiang, C., Wang, L., and Wu, G. (2022). Mixformer:
End-to-end tracking with iterative mixed attention. In
CVPR.
Fan, H., Lin, L., Yang, F., Chu, P., Deng, G., Yu, S., Bai,
H., Xu, Y., Liao, C., and Ling, H. (2019). Lasot: A
high-quality benchmark for large-scale single object
tracking. In CVPR.
Guo, D., Shao, Y., Cui, Y., Wang, Z., Zhang, L., and Shen,
C. (2021). Graph attention tracking. In CVPR.
Guo, D., Wang, J., Cui, Y., Wang, Z., and Chen, S. (2020).
Siamcar: Siamese fully convolutional classification
and regression for visual tracking. In CVPR.
He, K., Zhang, X., Ren, S., and Sun, J. (2016). Deep resid-
ual learning for image recognition. In CVPR.
Huang, L., Zhao, X., and Huang, K. (2019). Got-10k:
A large high-diversity benchmark for generic object
tracking in the wild. In TPAML, volume 43. IEEE.
Jiang, B., Luo, R., Mao, J., Xiao, T., and Jiang, Y. (2018).
Acquisition of localization confidence for accurate ob-
ject detection. In ECCV.
Kingma Diederik, P. and Adam, J. B. (2014). A
method for stochastic optimization. arXiv preprint
arXiv:1412.6980.
Kristan, M., Leonardis, A., Matas, J., Felsberg, M.,
Pflugfelder, R., ˇCehovin Zajc, L., Vojir, T., Bhat, G.,
Lukezic, A., Eldesokey, A., et al. (2018). The sixth
visual object tracking vot2018 challenge results. In
ECCV Workshops.
Li, B., Wu, W., Wang, Q., Zhang, F., Xing, J., and Yan,
J. (2019). Siamrpn++: Evolution of siamese visual
tracking with very deep networks. In CVPR.
Lin, T.-Y., Maire, M., Belongie, S., Hays, J., Perona, P., Ra-
manan, D., Doll
´
ar, P., and Zitnick, C. L. (2014). Mi-
crosoft coco: Common objects in context. In ECCV.
Luvizon, D. C., Tabia, H., and Picard, D. (2019). Human
pose regression by combining indirect part detection
and contextual information. In Computers & Graph-
ics, volume 85. Elsevier.
Mueller, M., Smith, N., and Ghanem, B. (2016). A bench-
mark and simulator for uav tracking. In ECCV.
Russakovsky, O., Deng, J., Su, H., Krause, J., Satheesh, S.,
Ma, S., Huang, Z., Karpathy, A., Khosla, A., Bern-
stein, M., et al. (2015). Imagenet large scale visual
recognition challenge. In IJCV, volume 115. Springer.
Schlag, I., Irie, K., and Schmidhuber, J. (2021). Linear
transformers are secretly fast weight programmers. In
ICML. PMLR.
Tian, Z., Shen, C., Chen, H., and He, T. (2019). Fcos: Fully
convolutional one-stage object detection. In ICCV.
Vaswani, A., Shazeer, N., Parmar, N., Uszkoreit, J., Jones,
L., Gomez, A. N., Kaiser, Ł., and Polosukhin, I.
(2017). Attention is all you need. In NIPS, volume 30.
Wang, N., Zhou, W., Wang, J., and Li, H. (2021). Trans-
former meets tracker: Exploiting temporal context for
robust visual tracking. In CVPR.
Wu, Y., Lim, J., and Yang, M.-H. (2015). Object tracking
benchmark. In TPAMI, volume 37.
Yan, B., Zhang, X., Wang, D., Lu, H., and Yang, X. (2021).
Alpha-refine: Boosting tracking performance by pre-
cise bounding box estimation. In CVPR.
Yu, J., Jiang, Y., Wang, Z., Cao, Z., and Huang, T. (2016).
Unitbox: An advanced object detection network. In
24th ACM international conference on Multimedia.
Yu, Y., Xiong, Y., Huang, W., and Scott, M. R. (2020). De-
formable siamese attention networks for visual object
tracking. In CVPR.
Zhang, Z., Liu, Y., Wang, X., Li, B., and Hu, W. (2021).
Learn to match: Automatic matching network design
for visual tracking. In ICCV.
Zhang, Z., Peng, H., Fu, J., Li, B., and Hu, W. (2020).
Ocean: Object-aware anchor-free tracking. In ECCV.
Zhao, M., Okada, K., and Inaba, M. (2021). Trtr:
Visual tracking with transformer. arXiv preprint
arXiv:2105.03817.
ICPRAM 2023 - 12th International Conference on Pattern Recognition Applications and Methods
614