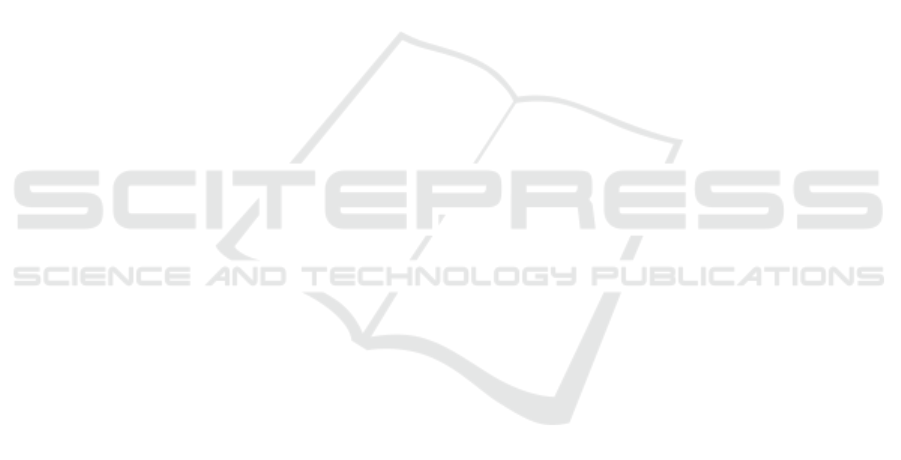
Das, S., Mullick, S. S., and Zelinka, I. (2022). On super-
vised class-imbalanced learning: An updated perspec-
tive and some key challenges. IEEE Transactions on
Artificial Intelligence, 3(6):973–993.
F
¨
urnkranz, J., H
¨
ullermeier, E., Loza Menc
´
ıa, E., and
Brinker, K. (2008). Multilabel classification via cali-
brated label ranking. Mach. Learn., 73(2):133–153.
Garc
´
ıa, S., Fern
´
andez, A., Luengo, J., and Herrera, F.
(2010). Advanced nonparametric tests for multi-
ple comparisons in the design of experiments in
computational intelligence and data mining: Exper-
imental analysis of power. Information sciences,
180(10):2044–2064.
Godbole, S. and Sarawagi, S. (2004). Discriminative meth-
ods for multi-labeled classification. In Proceedings
of the 8th Pacific-Asia Conference on Knowledge Dis-
covery and Data Mining, pages 22–30.
He, H. and Garcia, E. A. (2009). Learning from imbal-
anced data. IEEE Trans. on Knowl. and Data Eng.,
21(9):1263–1284.
Huang, J., Li, G., Huang, Q., and Wu, X. (2018). Joint fea-
ture selection and classification for multilabel learn-
ing. IEEE Transactions on Cybernetics, 48(3):876–
889.
Huang, J., Qin, F., Zheng, X., Cheng, Z., Yuan, Z., Zhang,
W., and Huang, Q. (2019). Improving multi-label
classification with missing labels by learning label-
specific features. Information Sciences, 492:124–146.
Joachims, T. (1998). Text categorization with support vec-
tor machines: Learning with many relevant features.
In European conference on machine learning, pages
137–142. Springer.
Li, F., Miao, D., and Pedrycz, W. (2017). Granular multi-
label feature selection based on mutual information.
Pattern Recognition, 67:410 – 423.
Li, T. and Ogihara, M. (2006). Toward intelligent music
information retrieval. Multimedia, IEEE Transactions
on, 8(3):564–574.
Li, X., Zhao, F., and Guo, Y. (2014). Multi-label image
classification with a probabilistic label enhancement
model. In Uncertainty in Artificial Intelligence.
Liu, B. and Tsoumakas, G. (2019). Synthetic oversampling
of multi-label data based on local label distribution. In
Joint European Conference on Machine Learning and
Knowledge Discovery in Databases, pages 180–193.
Springer.
Nam, J., Kim, J., Menc
´
ıa, E. L., Gurevych, I., and
F
¨
urnkranz, J. (2014). Large-scale multi-label
text classification—revisiting neural networks. In
Joint european conference on machine learning and
knowledge discovery in databases, pages 437–452.
Springer.
Nasierding, G., Tsoumakas, G., and Kouzani, A. Z. (2009).
Clustering based multi-label classification for image
annotation and retrieval. In Systems, Man and Cyber-
netics, 2009. SMC 2009. IEEE International Confer-
ence on, pages 4514–4519.
Pakrashi, A. and Namee, B. M. (2017). Stacked-MLkNN: A
stacking based improvement to multi-label k-nearest
neighbours. In LIDTA@PKDD/ECML.
Park, S. and F
¨
urnkranz, J. (2007). Efficient pairwise clas-
sification. In ECML 2007. LNCS (LNAI, pages 658–
665. Springer.
Pereira, R. M., Costa, Y. M., and Silla Jr, C. N. (2020a).
Mltl: A multi-label approach for the tomek link under-
sampling algorithm. Neurocomputing, 383:95–105.
Pereira, R. M., Costa, Y. M., and Silla Jr., C. N. (2020b).
MLTL: A multi-label approach for the tomek link
undersampling algorithm. Neurocomputing, 383:95–
105.
Petterson, J. and Caetano, T. S. (2010). Reverse multi-label
learning. In Advances in Neural Information Process-
ing Systems 23, pages 1912–1920. Curran Associates,
Inc.
Pillai, I., Fumera, G., and Roli, F. (2013). Threshold opti-
misation for multi-label classifiers. Pattern Recogn.,
46(7):2055–2065.
Qi, G.-J., Hua, X.-S., Rui, Y., Tang, J., Mei, T., and Zhang,
H.-J. (2007). Correlative multi-label video annotation.
In Proceedings of the 15th ACM International Con-
ference on Multimedia, MM ’07, pages 17–26, New
York, NY, USA. ACM.
Read, J., Martino, L., and Luengo, D. (2013). Effi-
cient monte carlo optimization for multi-label classi-
fier chains. pages 3457–3461.
Read, J., Pfahringer, B., Holmes, G., and Frank, E. (2011).
Classifier chains for multi-label classification. Ma-
chine learning, 85(3):333.
Sadhukhan, P. and Palit, S. (2019). Reverse-nearest neigh-
borhood based oversampling for imbalanced, multi-
label datasets. Pattern Recognition Letters, 125:813 –
820.
Su, H. and Rousu, J. (2015). Multilabel classification
through random graph ensembles. Machine Learning,
99(2).
Tahir, M. A., Kittler, J., and Yan, F. (2012). Inverse
random under sampling for class imbalance prob-
lem and its application to multi-label classification.
45(10):3738–3750.
Tanaka, E. A., Nozawa, S. R., Macedo, A. A., and
Baranauskas, J. A. (2015). A multi-label approach
using binary relevance and decision trees applied to
functional genomics. Journal of Biomedical Informat-
ics, 54:85–95.
Tsoumakas, G., Katakis, I., and Vlahavas, I. (2011). Ran-
dom k-labelsets for multilabel classification. IEEE
Transactions on Knowledge and Data Engineering,
23(7):1079–1089.
Xu, J. (2018). A weighted linear discriminant analysis
framework for multi-label feature extraction. Neuro-
computing, 275:107–120.
Xu, J., Liu, J., Yin, J., and Sun, C. (2016). A multi-
label feature extraction algorithm via maximizing fea-
ture variance and feature-label dependence simultane-
ously. Knowledge-Based Systems, 98:172–184.
Younes, Z., Abdallah, F., and Denœux, T. (2008). Multi-
label classification algorithm derived from k-nearest
neighbor rule with label dependencies. In 2008 16th
European Signal Processing Conference, pages 1–5.
IEEE.
Integrating Unsupervised Clustering and Label-Specific Oversampling to Tackle Imbalanced Multi-Label Data
497