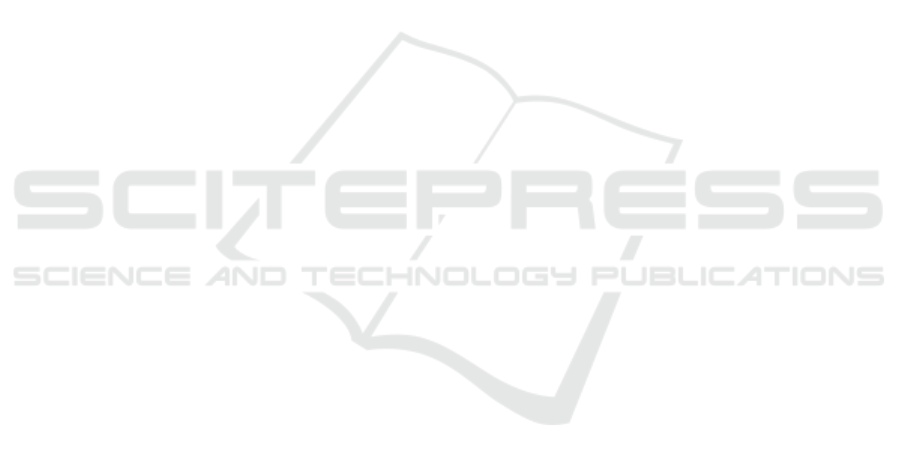
classifier into a two level grade classification scheme.
Computer Methods and Programs in Biomedicine,
90(3):251–261.
Gurcan, M. N., Boucheron, L. E., Can, A., Madabhushi, A.,
Rajpoot, N. M., and Yener, B. (2009). Histopatho-
logical Image Analysis: A Review. IEEE Reviews in
Biomedical Engineering, 2:147–171.
Irshad, H., Jalali, S., Roux, L., Racoceanu, D., Naour, G.,
Hwee, L., and Capron, F. (2013). Automated mito-
sis detection using texture, SIFT features and HMAX
biologically inspired approach. Journal of Pathology
Informatics, 4(2):12.
Irshad, H., Veillard, A., Roux, L., and Racoceanu, D.
(2014). Methods for nuclei detection, segmentation,
and classification in digital histopathology: A review-
current status and future potential. IEEE Reviews in
Biomedical Engineering, 7:97–114.
Komura, D. and Ishikawa, S. (2018). Machine Learn-
ing Methods for Histopathological Image Analysis.
Computational and Structural Biotechnology Journal,
16:34–42.
Kostopoulos, S., Glotsos, D., Cavouras, D., Daskalakis,
A., Kalatzis, I., Georgiadis, P., Bougioukos, P., Rava-
zoula, P., and Nikiforidis, G. ANALYTICAL AND
QUANTITATIVE CYTOLOGY AND HISTOLOGY
® ARTICLES Computer-Based Association of the
Texture of Expressed Estrogen Receptor Nuclei with
Histologic Grade Using Immunohistochemically-
Stained Breast Carcinomas. Technical report.
Kumar, M. D., Babaie, M., Zhu, S., Kalra, S., and Tizhoosh,
H. R. (2018). A comparative study of CNN, BoVW
and LBP for classification of histopathological im-
ages. 2017 IEEE Symposium Series on Computational
Intelligence, SSCI 2017 - Proceedings, 2018-Janua:1–
7.
Leutenegger, S., Chli, M., and Siegwart, R. Y. (2011).
BRISK: Binary Robust invariant scalable keypoints.
Proceedings of the IEEE International Conference on
Computer Vision, pages 2548–2555.
Li, C., Chen, H., Li, X., Xu, N., Hu, Z., Xue, D., Qi, S.,
Ma, H., Zhang, L., and Sun, H. (2020). A review for
cervical histopathology image analysis using machine
vision approaches, volume 53.
Li, C., Chen, H., Xue, D., Hu, Z., Zhang, L., He, L., Xu, N.,
Qi, S., Ma, H., and Sun, H. (2019a). Weakly Super-
vised Cervical Histopathological Image Classification
Using Multilayer Hidden Conditional Random Fields.
In Advances in Intelligent Systems and Computing,
volume 1011, pages 209–221. Springer Verlag.
Li, C., Chen, H., Zhang, L., Xu, N., Xue, D., Hu, Z., Ma,
H., and Sun, H. (2019b). Cervical Histopathology
Image Classification Using Multilayer Hidden Condi-
tional Random Fields and Weakly Supervised Learn-
ing. IEEE Access, 7:90378–90397.
Litjens, G., Bandi, P., Bejnordi, B. E., Geessink, O., Balken-
hol, M., Bult, P., Halilovic, A., Hermsen, M., van de
Loo, R., Vogels, R., Manson, Q. F., Stathonikos, N.,
Baidoshvili, A., van Diest, P., Wauters, C., van Dijk,
M., and van der Laak, J. (2018). 1399 H&E-stained
sentinel lymph node sections of breast cancer patients:
The CAMELYON dataset. GigaScience, 7(6):1–8.
Lowe, D. G. (1999). Object recognition from local scale-
invariant features. In Proceedings of the IEEE Inter-
national Conference on Computer Vision, volume 2,
pages 1150–1157. IEEE.
Ninos, K., Kostopoulos, S., Kalatzis, I., Sidiropoulos,
K., Ravazoula, P., Sakellaropoulos, G., Panayiotakis,
G., Economou, G., and Cavouras, D. (2016). Mi-
croscopy image analysis of p63 immunohistochemi-
cally stained laryngeal cancer lesions for predicting
patient 5-year survival. European Archives of Oto-
Rhino-Laryngology, 273(1):159–168.
Ouddai, G., Hamdi, I., and Ben Ghezala, H. (2023). Color-
SIFT Features for Histopathological Image Analysis.
In Lee, R., editor, Software Engineering, Artificial In-
telligence, Networking and Parallel/Distributed Com-
puting. SNPD 2022. Studies in Computational Intelli-
gence. (in press). Springer cham.
¨
Ozt
¨
urk,
˚
A and Akdemir, B. (2018). Application
of Feature Extraction and Classification Methods
for Histopathological Image using GLCM, LBP,
LBGLCM, GLRLM and SFTA. In Procedia Com-
puter Science, volume 132, pages 40–46. Elsevier
B.V.
Popovici, V., Budinsk
´
a, E.,
ˇ
C
´
apkov
´
a, L., Schwarz, D.,
Du
ˇ
sek, L., Feit, J., and Jaggi, R. (2016). Joint analysis
of histopathology image features and gene expression
in breast cancer. BMC Bioinformatics, 17(1):1–9.
Rosten, E., Porter, R., and Drummond, T. (2010). Faster
and better: A machine learning approach to corner de-
tection. IEEE Transactions on Pattern Analysis and
Machine Intelligence, 32(1):105–119.
Sanchez-Morillo, D., Gonz
´
alez, J., Garc
´
ıa-Rojo, M., and
Ortega, J. (2018). Classification of Breast Cancer
Histopathological Images Using KAZE Features. Lec-
ture Notes in Computer Science (including subseries
Lecture Notes in Artificial Intelligence and Lecture
Notes in Bioinformatics), 10814 LNBI:276–286.
Shafiei, S., Babaie, M., Kalra, S., and Tizhoosh, H. R.
(2021). Colored Kimia Path24 Dataset: Configura-
tions and Benchmarks with Deep Embeddings.
Simon, O., Yacoub, R., Jain, S., Tomaszewski, J. E., and
Sarder, P. (2018). Multi-radial LBP Features as a Tool
for Rapid Glomerular Detection and Assessment in
Whole Slide Histopathology Images. Scientific Re-
ports, 8(1):1–11.
Spanhol, F. A., Oliveira, L. S., Petitjean, C., and Heutte,
L. (2016). A Dataset for Breast Cancer Histopatho-
logical Image Classification. IEEE Transactions on
Biomedical Engineering, 63(7):1455–1462.
Tola, E., Lepetit, V., and Fua, P. (2010). DAISY: An effi-
cient dense descriptor applied to wide-baseline stereo.
IEEE Transactions on Pattern Analysis and Machine
Intelligence, 32(5):815–830.
Wang, C. W., Chang, C. C., Khalil, M. A., Lin, Y. J., Liou,
Y. A., Hsu, P. C., Lee, Y. C., Wang, C. H., and Chao,
T. K. (2022). Histopathological whole slide image
dataset for classification of treatment effectiveness to
ovarian cancer. Scientific Data, 9(1):1–5.
ICPRAM 2023 - 12th International Conference on Pattern Recognition Applications and Methods
970