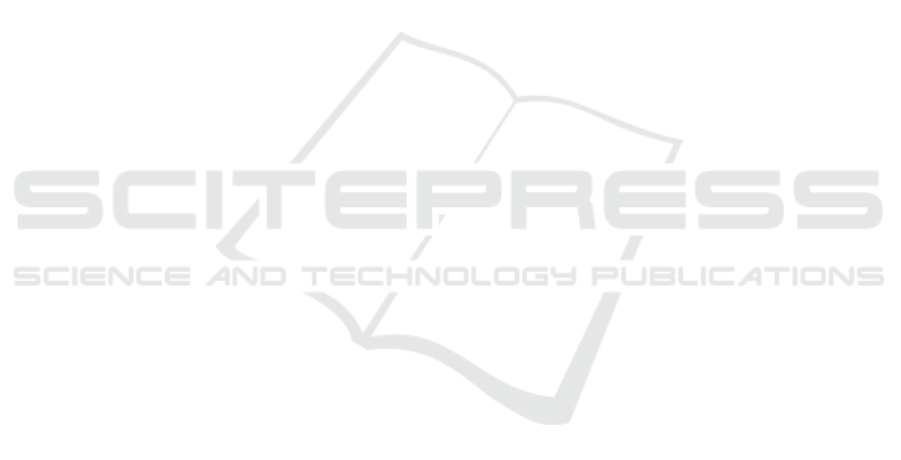
tree as there are accurate angles and distances be-
tween nodes in 3D.
ACKNOWLEDGEMENTS
This research received funding from the Flemish Gov-
ernment (AI Research Program).
REFERENCES
Adeli, V., Adeli, E., Reid, I., Niebles, J. C., and Rezatofighi,
H. (2020). Socially and contextually aware human
motion and pose forecasting. IEEE Robotics and Au-
tomation Letters, 5(4):6033–6040.
Ben-Shabat, Y., Yu, X., Saleh, F., Campbell, D., Rodriguez-
Opazo, C., Li, H., and Gould, S. (2020). The ikea
asm dataset: Understanding people assembling furni-
ture through actions, objects and pose.
Butepage, J., Black, M. J., Kragic, D., and Kjellstrom, H.
(2017). Deep representation learning for human mo-
tion prediction and classification. In Proceedings of
the IEEE conference on computer vision and pattern
recognition, pages 6158–6166.
Chen, D., Lin, Y., Li, W., Li, P., Zhou, J., and Sun, X.
(2020). Measuring and relieving the over-smoothing
problem for graph neural networks from the topolog-
ical view. In Proceedings of the AAAI Conference on
Artificial Intelligence, volume 34, pages 3438–3445.
Chiu, H.-k., Adeli, E., Wang, B., Huang, D.-A., and
Niebles, J. C. (2019). Action-agnostic human pose
forecasting. In 2019 IEEE Winter Conference on Ap-
plications of Computer Vision (WACV), pages 1423–
1432. IEEE.
Diller, C., Funkhouser, T., and Dai, A. (2022). Forecast-
ing actions and characteristic 3d poses. arXiv preprint
arXiv:2211.14309.
Ghoddoosian, R., Dwivedi, I., Agarwal, N., Choi, C., and
Dariush, B. (2022). Weakly-supervised online action
segmentation in multi-view instructional videos. In
Proceedings of the IEEE/CVF Conference on Com-
puter Vision and Pattern Recognition, pages 13780–
13790.
Gopalakrishnan, A., Mali, A., Kifer, D., Giles, L., and Oror-
bia, A. G. (2019). A neural temporal model for human
motion prediction. In Proceedings of the IEEE/CVF
Conference on Computer Vision and Pattern Recogni-
tion (CVPR).
Haresh, S., Kumar, S., Coskun, H., Syed, S. N., Konin, A.,
Zia, Z., and Tran, Q.-H. (2021). Learning by aligning
videos in time. In Proceedings of the IEEE/CVF Con-
ference on Computer Vision and Pattern Recognition,
pages 5548–5558.
He, K., Gkioxari, G., Dollar, P., and Girshick, R. (2017).
Mask r-cnn. In Proceedings of the IEEE International
Conference on Computer Vision (ICCV).
Hu, J., Fan, Z., Liao, J., and Liu, L. (2019). Predict-
ing long-term skeletal motions by a spatio-temporal
hierarchical recurrent network. arXiv preprint
arXiv:1911.02404.
Justusson, B. (1981). Median filtering: Statistical proper-
ties. Two-Dimensional Digital Signal Prcessing II,
pages 161–196.
Kwon, T., Tekin, B., Tang, S., and Pollefeys, M. (2022).
Context-aware sequence alignment using 4d skeletal
augmentation. In Proceedings of the IEEE/CVF Con-
ference on Computer Vision and Pattern Recognition
(CVPR), pages 8172–8182.
Li, Q., Chalvatzaki, G., Peters, J., and Wang, Y. (2021). Di-
rected acyclic graph neural network for human motion
prediction. In 2021 IEEE International Conference on
Robotics and Automation (ICRA), pages 3197–3204.
IEEE.
Liu, Z., Su, P., Wu, S., Shen, X., Chen, H., Hao, Y., and
Wang, M. (2021). Motion prediction using trajectory
cues. In Proceedings of the IEEE/CVF International
Conference on Computer Vision, pages 13299–13308.
Luo, W., Yang, B., and Urtasun, R. (2018). Fast and furi-
ous: Real time end-to-end 3d detection, tracking and
motion forecasting with a single convolutional net. In
Proceedings of the IEEE conference on Computer Vi-
sion and Pattern Recognition, pages 3569–3577.
Mart
´
ınez-Gonz
´
alez, A., Villamizar, M., and Odobez, J.-M.
(2021). Pose transformers (potr): Human motion pre-
diction with non-autoregressive transformers. In Pro-
ceedings of the IEEE/CVF International Conference
on Computer Vision, pages 2276–2284.
Mohamed, A., Chen, H., Wang, Z., and Claudel, C.
(2021). Skeleton-graph: Long-term 3d motion predic-
tion from 2d observations using deep spatio-temporal
graph cnns. arXiv preprint arXiv:2109.10257.
Nussbaumer, H. J. (1981). The fast fourier transform. In
Fast Fourier Transform and Convolution Algorithms,
pages 80–111. Springer.
Ragusa, F., Furnari, A., and Farinella, G. M. (2022). Mec-
cano: A multimodal egocentric dataset for humans be-
havior understanding in the industrial-like domain.
Sofianos, T., Sampieri, A., Franco, L., and Galasso, F.
(2021). Space-time-separable graph convolutional
network for pose forecasting. CoRR, abs/2110.04573.
Vendrow, E., Kumar, S., Adeli, E., and Rezatofighi, H.
(2022). Somoformer: Multi-person pose forecasting
with transformers. arXiv preprint arXiv:2208.14023.
Yuan, Y. and Kitani, K. (2020). Dlow: Diversifying latent
flows for diverse human motion prediction. In Euro-
pean Conference on Computer Vision, pages 346–364.
Springer.
Zhang, J., Liu, H., Chang, Q., Wang, L., and Gao, R. X.
(2020). Recurrent neural network for motion trajec-
tory prediction in human-robot collaborative assem-
bly. CIRP annals, 69(1):9–12.
Zhao, Z., Liu, Y., and Ma, L. (2022). Compositional action
recognition with multi-view feature fusion. Plos one,
17(4):e0266259.
Zheng, Y., Yang, Y., Mo, K., Li, J., Yu, T., Liu, Y.,
Liu, C. K., and Guibas, L. J. (2022). Gimo: Gaze-
informed human motion prediction in context. In Eu-
ropean Conference on Computer Vision, pages 676–
694. Springer.
VISAPP 2023 - 18th International Conference on Computer Vision Theory and Applications
914