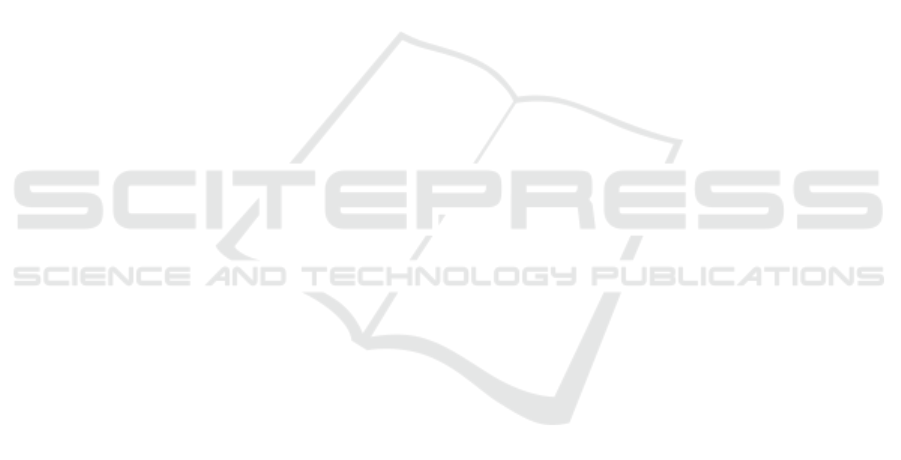
poral projection for multivariates time series. In In-
ternational Symposium on Visual Computing, pages
91–102. Springer.
Hartigan, J. (1975). Clustering Algorithms. John Wiley &
Sons, New York.
Hochheiser, H. and Shneiderman, B. (2004). Dynamic
query tools for time series data sets: Timebox widgets
for interactive exploration. Information Visualization,
3(1):1–18.
Inselberg, A. (1985). The plane with parallel coordinates.
The Visual Computer, 1(2):69–91.
Johansson, J. and Forsell, C. (2015). Evaluation of parallel
coordinates: Overview, categorization and guidelines
for future research. IEEE Transactions on Visualiza-
tion and Computer Graphics, 22:1–1.
Kanehisa, M., Furumichi, M., Sato, Y., Ishiguro-Watanabe,
M., and Tanabe, M. (2020). Kegg: Integrating viruses
and cellular organisms. Nucleic Acids Research, 49.
Kanehisa, M., Furumichi, M., Sato, Y., Kawashima, M., and
Ishiguro-Watanabe, M. (2022). KEGG for taxonomy-
based analysis of pathways and genomes. Nucleic
Acids Research. gkac963.
Keim, D. A. (2002). Information visualization and visual
data mining. IEEE Transactions on Visualization &
Computer Graphics, (1):1–8.
Krstajic, M., Bertini, E., and Keim, D. (2011). Cloudlines:
Compact display of event episodes in multiple time-
series. IEEE Transactions on Visualization and Com-
puter Graphics, 17(12):2432–2439.
Love, M. I., Huber, W., and Anders, S. (2014). Moderated
estimation of fold change and dispersion for rna-seq
data with deseq2. Genome Biology, 15(12):550.
Maza, E. (2016). In papyro comparison of tmm (edger),
rle (deseq2), and mrn normalization methods for a
simple two-conditions-without-replicates rna-seq ex-
perimental design. Frontiers in genetics, 7:164–164.
27695478[pmid].
McInnes, L., Healy, J., and Melville, J. (2018). Umap: Uni-
form manifold approximation and projection for di-
mension reduction.
M
¨
uller, W. and Schumann, H. (2004). Visualization meth-
ods for time-dependent data - an overview. volume 1,
pages 737 – 745 Vol.1.
Pham, C., Pham, V., and Dang, T. (2020). Genexplorer:
Visualizing and comparing gene expression levels via
differential charts. In Bebis, G., Yin, Z., Kim, E., Ben-
der, J., Subr, K., Kwon, B. C., Zhao, J., Kalkofen,
D., and Baciu, G., editors, Advances in Visual Com-
puting, pages 248–259, Cham. Springer International
Publishing.
Reddy, R. (2015). A comparison of methods: Normalizing
high-throughput rna sequencing data. bioRxiv.
Robinson, M. D., McCarthy, D. J., and Smyth, G. K.
(2009). edgeR: a Bioconductor package for differ-
ential expression analysis of digital gene expression
data. Bioinformatics, 26(1):139–140.
Shneiderman, B. (1997). Designing the User Interface:
Strategies for Effective Human-Computer Interaction.
Addison-Wesley Longman Publishing Co., Inc., USA,
3rd edition.
survey. A visual survey of visualization techniques for time-
oriented data.
Tang, M., Sun, J., Shimizu, K., and Kadota, K. (2015).
Evaluation of methods for differential expression
analysis on multi-group rna-seq count data. BMC
Bioinformatics, 16(1):360.
Uchida, Y. and Itoh, T. (2009). A visualization and level-
of-detail control technique for large scale time series
data. In 2009 13th International Conference Informa-
tion Visualisation, pages 80–85.
Van Der Maaten, L. and Hinton, G. (2008). Visualizing
Data using t-SNE. 9:2579–2605.
Wold, S., Esbensen, K., and Geladi, P. (1987). Principal
component analysis.
BIOINFORMATICS 2023 - 14th International Conference on Bioinformatics Models, Methods and Algorithms
308