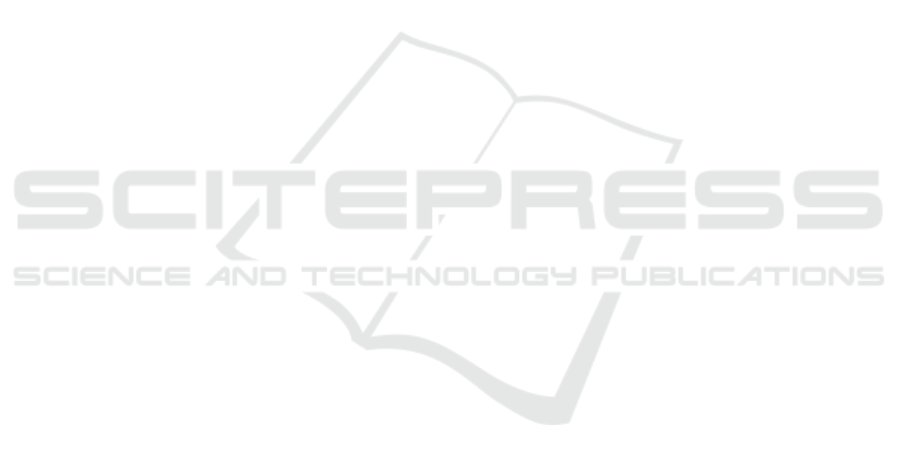
Chincarini, A., Peira, E., Morbelli, S., Pardini, M., Bauck-
neht, M., Arbizu, J., Castelo-Branco, M., B
¨
using,
K., De Mendonc¸a, A., Didic, M., et al. (2019).
Semi-quantification and grading of amyloid pet: A
project of the european alzheimer’s disease consor-
tium (eadc). NeuroImage: Clinical, 23:101846.
Colombi, D., Villani, G. D., Maffi, G., Risoli, C., Bodini,
F. C., Petrini, M., Morelli, N., Anselmi, P., Milanese,
G., Silva, M., et al. (2020). Qualitative and quan-
titative chest ct parameters as predictors of specific
mortality in covid-19 patients. Emergency radiology,
27(6):701–710.
Faggioni, L., Coppola, F., Ferrari, R., Neri, E., and Regge,
D. (2017). Usage of structured reporting in radiologi-
cal practice: results from an italian online survey. Eu-
ropean radiology, 27(5):1934–1943.
Fervers, P., Fervers, F., Jaiswal, A., Rinneburger, M.,
Weisthoff, M., Pollmann-Schweckhorst, P., Kottlors,
J., Carolus, H., Lennartz, S., Maintz, D., et al.
(2022). Assessment of covid-19 lung involvement on
computed tomography by deep-learning-, threshold-
, and human reader-based approaches—an interna-
tional, multi-center comparative study. Quantitative
Imaging in Medicine and Surgery, 12(11):5156–5170.
Hansell, D. M., Bankier, A. A., MacMahon, H., McLoud,
T. C., Muller, N. L., Remy, J., et al. (2008). Fleis-
chner society: glossary of terms for thoracic imaging.
Radiology, 246(3):697.
Kollias, D., Arsenos, A., and Kollias, S. (2022). Ai-mia:
Covid-19 detection & severity analysis through medi-
cal imaging. arXiv preprint arXiv:2206.04732.
Lizzi, F., Agosti, A., Brero, F., Cabini, R. F., Fantacci,
M. E., Figini, S., Lascialfari, A., Laruina, F., Oliva,
P., Piffer, S., et al. (2022). Quantification of pul-
monary involvement in covid-19 pneumonia by means
of a cascade of two u-nets: training and assessment
on multiple datasets using different annotation crite-
ria. International journal of computer assisted radiol-
ogy and surgery, 17(2):229–237.
Lyu, P., Liu, X., Zhang, R., Shi, L., and Gao, J. (2020).
The performance of chest ct in evaluating the clinical
severity of covid-19 pneumonia: identifying critical
cases based on ct characteristics. Investigative radiol-
ogy, 55(7):412–421.
Mergen, V., Kobe, A., Bl
¨
uthgen, C., Euler, A., Flohr,
T., Frauenfelder, T., Alkadhi, H., and Eberhard, M.
(2020). Deep learning for automatic quantification of
lung abnormalities in covid-19 patients: First experi-
ence and correlation with clinical parameters. Euro-
pean Journal of Radiology Open, 7:100272.
Neri, E., Coppola, F., Larici, A., Sverzellati, N., Mazzei,
M., Sacco, P., Dalpiaz, G., Feragalli, B., Miele, V., and
Grassi, R. (2020). Structured reporting of chest ct in
covid-19 pneumonia: a consensus proposal. Insights
into Imaging, 11(1):1–9.
of Radiology (ESR) communications@ myesr. org, E. S.
(2018). Esr paper on structured reporting in radiology.
Insights into Imaging, 9:1–7.
¨
Ozer, H., Kılınc¸er, A., Uysal, E., Yormaz, B., Cebeci,
H., Durmaz, M. S., and Koplay, M. (2021). Diag-
nostic performance of radiological society of north
america structured reporting language for chest com-
puted tomography findings in patients with covid-19.
Japanese journal of radiology, 39(9):877–888.
Rizzetto, F., Perillo, N., Artioli, D., Travaglini, F., Cuccia,
A., Zannoni, S., Tombini, V., Di Domenico, S. L.,
Albertini, V., Bergamaschi, M., et al. (2021). Cor-
relation between lung ultrasound and chest ct patterns
with estimation of pulmonary burden in covid-19 pa-
tients. European Journal of Radiology, 138:109650.
Rocha, D. M., Brasil, L. M., Lamas, J. M., Luz, G. V., and
Bacelar, S. S. (2020). Evidence of the benefits, advan-
tages and potentialities of the structured radiological
report: An integrative review. Artificial intelligence in
medicine, 102:101770.
Rubin, G. D., Ryerson, C. J., Haramati, L. B., Sverzellati,
N., Kanne, J. P., Raoof, S., Schluger, N. W., Volpi, A.,
Yim, J.-J., Martin, I. B., et al. (2020). The role of chest
imaging in patient management during the covid-19
pandemic: a multinational consensus statement from
the fleischner society. Radiology, 296(1):172–180.
Salvatore, C., Roberta, F., Angela, d. L., Cesare, P., Alfredo,
C., Giuliano, G., Giulio, L., Giuliana, G., Maria,
R. G., Paola, B. M., et al. (2021). Clinical and labo-
ratory data, radiological structured report findings and
quantitative evaluation of lung involvement on base-
line chest ct in covid-19 patients to predict prognosis.
La radiologia medica, 126(1):29–39.
Zhao, C., Xu, Y., He, Z., Tang, J., Zhang, Y., Han, J.,
Shi, Y., and Zhou, W. (2021). Lung segmentation
and automatic detection of covid-19 using radiomic
features from chest ct images. Pattern Recognition,
119:108071.
CCH 2023 - Special Session on Machine Learning and Deep Learning for Preventive Healthcare and Clinical Decision Support
670