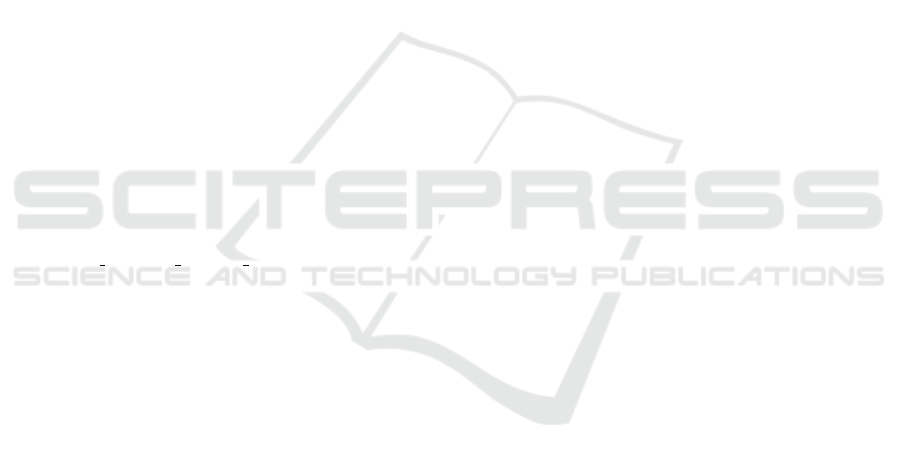
Ahmed, U., Lin, J. C.-W., and Srivastava, G. (2022). Hyper-
graph-based attention curriculum learning using a lex-
ical algorithm for mental health. Pattern Recognition
Letters, 157:135–143.
Alonso, V., Santos, J. V., Pinto, M., Ferreira, J., Lema, I.,
Lopes, F., and Freitas, A. (2020). Problems and bar-
riers during the process of clinical coding: a focus
group study of coders’ perceptions. Journal of medi-
cal systems, 44(3):1–8.
Biswas, B., Pham, T.-H., and Zhang, P. (2021). Transicd:
Transformer based code-wise attention model for ex-
plainable icd coding. In International Conference
on Artificial Intelligence in Medicine, pages 469–478.
Springer.
Campbell, S. and Giadresco, K. (2020). Computer-assisted
clinical coding: A narrative review of the literature
on its benefits, limitations, implementation and impact
on clinical coding professionals. Health Information
Management Journal, 49(1):5–18.
Catling, F., Spithourakis, G. P., and Riedel, S. (2018). To-
wards automated clinical coding. International jour-
nal of medical informatics, 120:50–61.
Chai, X. (2020). Diagnosis method of thyroid disease com-
bining knowledge graph and deep learning. IEEE Ac-
cess, 8:149787–149795.
Confalonieri, R., Coba, L., Wagner, B., and Besold, T. R.
(2021). A historical perspective of explainable arti-
ficial intelligence. Wiley Interdisciplinary Reviews:
Data Mining and Knowledge Discovery, 11(1):e1391.
Delangue, C. (2016). Hugging face – the ai community
building the future.
Desai, G. (2020). gauravkdesai/mids-w210-
medical
insurance payment assistant.
Devlin, J., Chang, M.-W., Lee, K., and Toutanova, K.
(2018). Bert: Pre-training of deep bidirectional trans-
formers for language understanding. arXiv preprint
arXiv:1810.04805.
Dong, H., Su
´
arez-Paniagua, V., Whiteley, W., and Wu,
H. (2021). Explainable automated coding of clinical
notes using hierarchical label-wise attention networks
and label embedding initialisation. Journal of biomed-
ical informatics, 116:103728.
Dranc
´
e, M. (2022). Neuro-symbolic xai: Application to
drug repurposing for rare diseases. In International
Conference on Database Systems for Advanced Ap-
plications, pages 539–543. Springer.
Futia, G. and Vetr
`
o, A. (2020). On the integration of
knowledge graphs into deep learning models for a
more comprehensible ai—three challenges for future
research. Information, 11(2):122.
Garcez, A. d. and Lamb, L. C. (2020). Neurosymbolic ai:
the 3rd wave. arXiv preprint arXiv:2012.05876.
Gaur, M., Faldu, K., and Sheth, A. (2021). Semantics of
the black-box: Can knowledge graphs help make deep
learning systems more interpretable and explainable?
IEEE Internet Computing, 25(1):51–59.
Gaur, M., Gunaratna, K., Bhatt, S., and Sheth, A. (2022).
Knowledge-infused learning: A sweet spot in neuro-
symbolic ai. IEEE Internet Computing, 26(4):5–11.
Graves, A., Fern
´
andez, S., and Schmidhuber, J. (2005).
Bidirectional lstm networks for improved phoneme
classification and recognition. In International con-
ference on artificial neural networks, pages 799–804.
Springer.
Gu, P., Yang, S., Li, Q., and Wang, J. (2021). Disease cor-
relation enhanced attention network for icd coding.
In 2021 IEEE International Conference on Bioinfor-
matics and Biomedicine (BIBM), pages 1325–1330.
IEEE.
Hitzler, P., Bianchi, F., Ebrahimi, M., and Sarker, M. K.
(2020). Neural-symbolic integration and the semantic
web. Semantic Web, 11(1):3–11.
Hochreiter, S. and Schmidhuber, J. (1997). Long short-term
memory. Neural computation, 9(8):1735–1780.
Hogan, A., Blomqvist, E., Cochez, M., d’Amato, C., Melo,
G. D., Gutierrez, C., Kirrane, S., Gayo, J. E. L.,
Navigli, R., Neumaier, S., et al. (2021). Knowledge
graphs. ACM Computing Surveys (CSUR), 54(4):1–
37.
Johnson, A. E., Pollard, T. J., Shen, L., Lehman, L.-w. H.,
Feng, M., Ghassemi, M., Moody, B., Szolovits, P.,
Anthony Celi, L., and Mark, R. G. (2016). Mimic-
iii, a freely accessible critical care database. Scientific
data, 3(1):1–9.
Johnson, S. L. and Linker, R. (2015). Understanding medi-
cal coding: A comprehensive guide. Cengage Learn-
ing.
Khalid, M., Khattak, H. A., Ahmad, A., and Bukhari, S.
A. C. (2022). Explainable prediction of medical codes
through automated knowledge graph curation frame-
work. In Proceedings of 2022 19th International
Bhurban Conference on Applied Sciences & Technol-
ogy (IBCAST) Islamabad, Pakistan, 16th-20th August,
2022, pages 1–6. IEEE.
Lu, H., Uddin, S., Hajati, F., Khushi, M., and Moni, M. A.
(2022). Predictive risk modelling in mental health
issues using machine learning on graphs. In Aus-
tralasian Computer Science Week 2022, pages 168–
175.
Malik, K. M., Krishnamurthy, M., Alobaidi, M., Hussain,
M., Alam, F., and Malik, G. (2020). Automated
domain-specific healthcare knowledge graph curation
framework: Subarachnoid hemorrhage as phenotype.
Expert Systems with Applications, 145:113120.
Moons, E., Khanna, A., Akkasi, A., and Moens, M.-F.
(2020). A comparison of deep learning methods for
icd coding of clinical records. Applied Sciences,
10(15):5262.
Mullenbach, J., Wiegreffe, S., Duke, J., Sun, J., and
Eisenstein, J. (2018). Explainable prediction of
medical codes from clinical text. arXiv preprint
arXiv:1802.05695.
Niu, Z., Zhong, G., and Yu, H. (2021). A review on the
attention mechanism of deep learning. Neurocomput-
ing, 452:48–62.
Noy, N. F., Shah, N. H., Whetzel, P. L., Dai, B., Dorf, M.,
Griffith, N., Jonquet, C., Rubin, D. L., Storey, M.-A.,
Chute, C. G., et al. (2009). Bioportal: ontologies and
CCH 2023 - Special Session on Machine Learning and Deep Learning for Preventive Healthcare and Clinical Decision Support
636