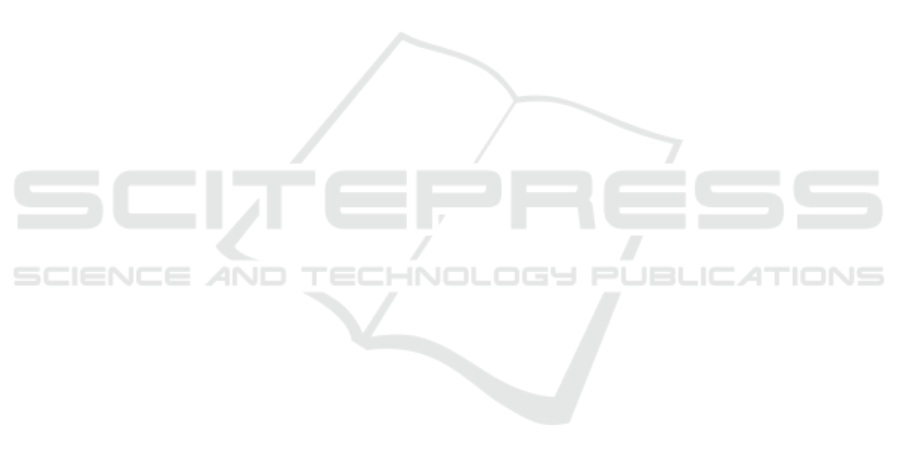
near future, with both areas positively feeding each
other in terms of research questions and tasks, and
also solutions. A strong common mathematical back-
ground also unites researchers in the two fields, mak-
ing it easy to exchange research questions, ideas, and
results. We also see several high-potential research di-
rections at the crossroads of ML and DR: using dense
maps to explore and improve classifiers and regres-
sors, effectively mapping the whole high-dimensional
space to an image; using ML to create highly cus-
tomized, high-quality projections for both static and
dynamic data; and developing inverse projections to
meet all the standards that current direct projection
techniques have. Such developments, jointly enabled
by DR and ML researchers, will have impact far be-
yond these two fields.
REFERENCES
Amorim, E., Brazil, E., Daniels, J., Joia, P., Nonato, L.,
and Sousa, M. (2012). iLAMP: Exploring high-
dimensional spacing through backward multidimen-
sional projection. In Proc. IEEE VAST.
Amorim, E., Brazil, E., Mena-Chalco, J., Velho, L., Nonato,
L. G., Samavati, F., and Sousa, M. (2015). Facing the
high-dimensions: Inverse projection with radial basis
functions. Computers & Graphics, 48:35–47.
Appleby, G., Espadoto, M., Chen, R., Goree, S., Telea, A.,
Anderson, E., and Chang, R. (2022). HyperNP: Inter-
active visual exploration of multidimensional projec-
tion hyperparameters. CGF, 41(3):169–181.
Azodi, C., Tang, J., and Shiu, S. (2020). Opening the black
box: Interpretable machine learning for geneticists.
Trends Genet, 36(6):442–455.
Benato, B., Gomes, J., Telea, A., and Falc
˜
ao, A. (2020).
Semi-automatic data annotation guided by feature
space projection. Pattern Recognition, 109:107612.
Benato, B., Telea, A., and Falc
˜
ao, A. (2018). Semi-
supervised learning with interactive label propagation
guided by feature space projections. In Proc. SIB-
GRAPI, pages 392–399.
Botchkarev, A. (2019). Performance metrics (error mea-
sures) in machine learning regression, forecasting and
prognostics: Properties and typology. Interdisci-
plinary J. of Information, Knowledge, and Manage-
ment, 14:45–79.
Bredius, C., Tian, Z., and Telea, A. (2022). Visual explo-
ration of neural network projection stability. In Proc.
MLVis.
Duarte, F., Sikanski, F., Fatore, F., Fadel, S., and
Paulovich, F. V. (2014). Nmap: A novel neighborhood
preservation space-filling algorithm. IEEE TVCG,
20(12):2063–2071.
Espadoto, M., Appleby, G., A. Suh, D. C., Li, M.,
Scheidegger, C., Anderson, E., Chang, R.,
and Telea, A. (2021a). UnProjection: Lever-
aging inverse-projections for visual analyt-
ics of high-dimensional data. IEEE TVCG.
DOI:10.1109/TVCG.2021.3125576.
Espadoto, M., Hirata, N., and Telea, A. (2020). Deep learn-
ing multidimensional projections. Information Visual-
ization, 9(3):247–269.
Espadoto, M., Hirata, N., and Telea, A. (2021b). Self-
supervised dimensionality reduction with neural net-
works and pseudo-labeling. In Proc. IVAPP.
Espadoto, M., Martins, R., Kerren, A., Hirata, N., and
Telea, A. (2019a). Toward a quantitative survey
of dimension reduction techniques. IEEE TVCG,
27(3):2153–2173.
Espadoto, M., Rodrigues, F. C. M., Hirata, N., and Telea,
A. (2021c). OptMap: Using dense maps for visualiz-
ing multidimensional optimization problems. In Proc.
IVAPP.
Espadoto, M., Rodrigues, F. C. M., Hirata, N. S. T., Jr,
R. H., and Telea, A. (2019b). Deep learning inverse
multidimensional projections. In Proc. EuroVA.
Garcia, R., Telea, A., da Silva, B., Torresen, J., and Comba,
J. (2018). A task-and-technique centered survey on
visual analytics for deep learning model engineering.
Computers and Graphics, 77:30–49.
Geurts, P., Ernst, D., and Wehenkel, L. (2006). Extremely
randomized trees. Machine learning, 63(1):3–42.
Guyon, I., S, S. G., and Ben-Hur, A. (2004). Result anal-
ysis of the NIPS 2003 feature selection challenge. In
Advances in Neural Information Processing Systems,
page 545?552.
Hinton, G. E. and Salakhutdinov, R. R. (2006). Reducing
the dimensionality of data with neural networks. Sci-
ence, 313(5786):504–507.
Jiang, T., Gradus, J., and Rosellini, A. (2020). Supervised
machine learning: A brief primer. Behavior Therapy,
51(5):675–687.
Kim, Y., Espadoto, M., Trager, S., Roerdink, J., and Telea,
A. (2022). SDR-NNP: Sharpened dimensionality re-
duction with neural networks. In Proc. IVAPP.
Lespinats, S. and Aupetit, M. (2011). CheckViz: Sanity
check and topological clues for linear and nonlinear
mappings. CGF, 30(1):113–125.
Luan, F., Paris, S., Shechtman, E., and Bala, K. (2017).
Deep photo style transfer. In Proc. IEEE CVPR.
McInnes, L., Healy, J., and Melville, J. (2018). UMAP:
Uniform manifold approximation and projection for
dimension reduction. arXiv:1802.03426v2 [stat.ML].
Minghim, R., Paulovich, F. V., and Lopes, A. A. (2006).
Content-based text mapping using multi-dimensional
projections for exploration of document collections.
In Proc. SPIE. Intl. Society for Optics and Photonics.
Modrakowski, T., Espadoto, M., Falcao, A., Hirata, N., and
Telea, A. (2021). Improving deep learning projections
by neighborhood analysis. In Communication in Com-
puter and Information. Springer.
Nonato, L. and Aupetit, M. (2018). Multidimensional
projection for visual analytics: Linking techniques
with distortions, tasks, and layout enrichment. IEEE
TVCG.
Beyond the Third Dimension: How Multidimensional Projections and Machine Learning Can Help Each Other
15