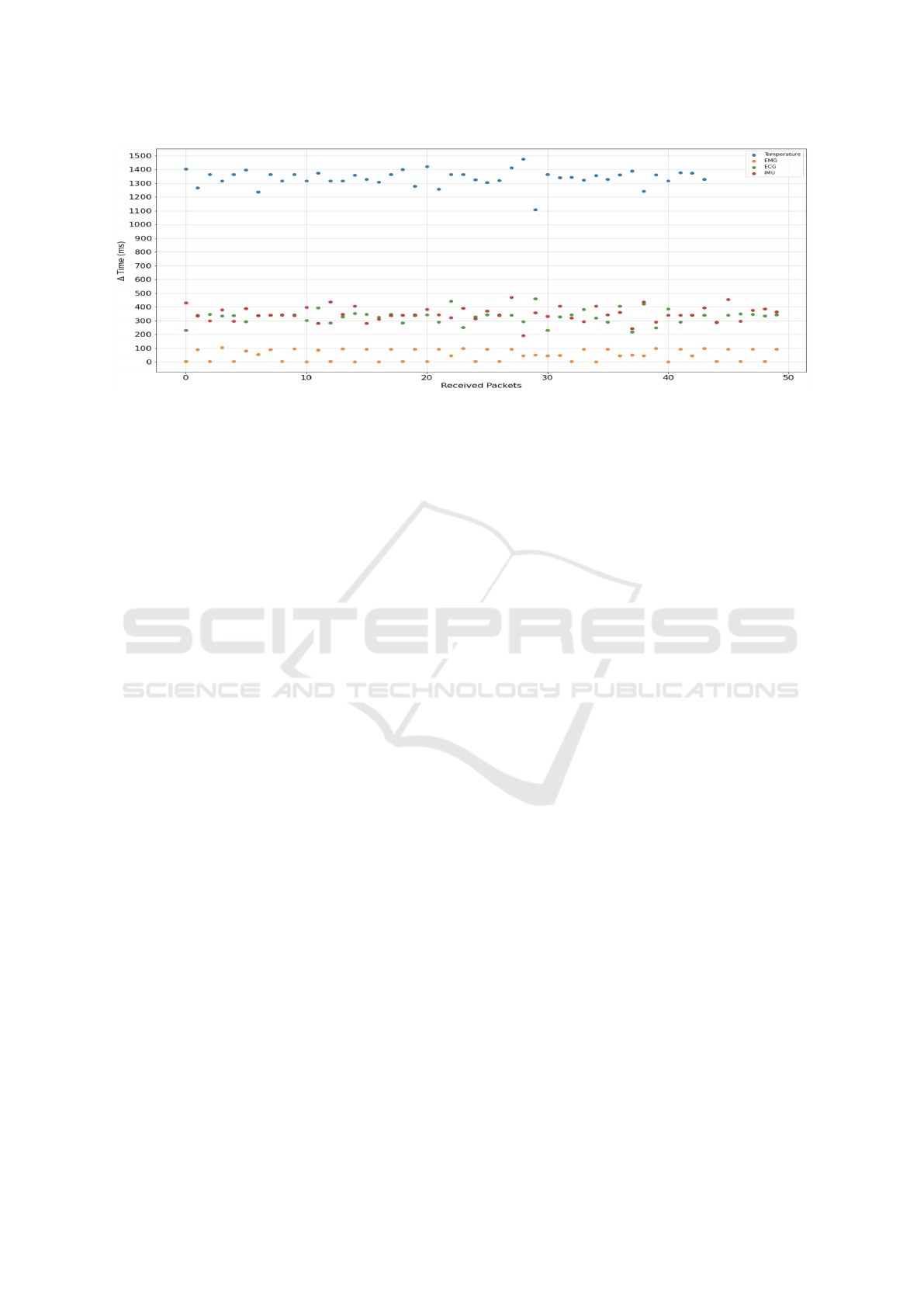
Figure 9: Received Data Packets ∆ Time.
REFERENCES
(2022). Ageing and health. https://www.who.int/news-
room/fact-sheets/detail/ageing-and-health. Accessed:
2022-05-12.
Ajdaraga, E. and Gusev, M. (2017). Analysis of sam-
pling frequency and resolution in ecg signals. In 2017
25th Telecommunication Forum (TELFOR), pages 1–
4. IEEE.
Ali, H., Naing, H. H., and Yaqub, R. (2021). An iot assisted
real-time high cmrr wireless ambulatory ecg monitor-
ing system with arrhythmia detection. Electronics,
10(16):1871.
Berkaya, S. K., Uysal, A. K., Gunal, E. S., Ergin, S., Gunal,
S., and Gulmezoglu, M. B. (2018). A survey on ecg
analysis. Biomedical Signal Processing and Control,
43:216–235.
Biometrics Ltd (2021). Datalog MWX8.
http://www.biometricsltd.com/datalog.htm. Ac-
cessed: 26.11.2021.
Bruce-Brand, R. A., Walls, R. J., Ong, J. C., Emerson, B. S.,
O’Byrne, J. M., and Moyna, N. M. (2012). Effects
of home-based resistance training and neuromuscular
electrical stimulation in knee osteoarthritis: a random-
ized controlled trial. BMC musculoskeletal disorders,
13(1):1–10.
Butt, F. S., La Blunda, L., Wagner, M. F., Sch
¨
afer, J.,
Medina-Bulo, I., and G
´
omez-Ullate, D. (2021). Fall
detection from electrocardiogram (ecg) signals and
classification by deep transfer learning. Information,
12(2):63.
Dolson, C. M., Harlow, E. R., Phelan, D. M., Gabbett, T. J.,
Gaal, B., McMellen, C., Geletka, B. J., Calcei, J. G.,
Voos, J. E., and Seshadri, D. R. (2022). Wearable sen-
sor technology to predict core body temperature: A
systematic review. Sensors, 22(19):7639.
Jeong, J.-W., Lee, W., and Kim, Y.-J. (2021). A real-time
wearable physiological monitoring system for home-
based healthcare applications. Sensors, 22(1):104.
Kwon, O., Jeong, J., Kim, H. B., Kwon, I. H., Park, S. Y.,
Kim, J. E., and Choi, Y. (2018). Electrocardiogram
sampling frequency range acceptable for heart rate
variability analysis. Healthcare informatics research,
24(3):198–206.
Lou, Z., Wang, L., Jiang, K., Wei, Z., and Shen, G. (2020).
Reviews of wearable healthcare systems: Materials,
devices and system integration. Materials Science and
Engineering: R: Reports, 140:100523.
Marin-Pardo, O., Laine, C. M., Rennie, M., Ito, K. L.,
Finley, J., and Liew, S.-L. (2020). A virtual reality
muscle–computer interface for neurorehabilitation in
chronic stroke: A pilot study. Sensors, 20(13):3754.
Melillo, P., Castaldo, R., Sannino, G., Orrico, A., De Pietro,
G., and Pecchia, L. (2015). Wearable technology and
ecg processing for fall risk assessment, prevention and
detection. In 2015 37th Annual International Confer-
ence of the IEEE Engineering in Medicine and Biol-
ogy Society (EMBC), pages 7740–7743. IEEE.
Mina, S. M. (2021). Chronobiological assessment. In Refer-
ence Module in Neuroscience and Biobehavioral Psy-
chology. Elsevier.
Muharrem Adak, Vecteezy (2022).
https://pt.vecteezy.com/foto/9213086-
3d-renderizacao-de-tronco-humano and
https://pt.vecteezy.com/foto/9211711-3d-
renderizacao-de-tronco-humano. Accessed:
25.10.2022.
Qian, Q., Hu, X., Lai, Q., Ng, S., Zheng, Y., and Poon, W.-
S. (2017). Early stroke rehabilitation of the upper limb
assisted with an electromyography-driven neuromus-
cular electrical stimulation-robotic arm. Frontiers in
Neurology, 8.
Queralta, J. P., Gia, T. N., Tenhunen, H., and Westerlund, T.
(2019). Edge-ai in lora-based health monitoring: Fall
detection system with fog computing and lstm recur-
rent neural networks. In 2019 42nd international con-
ference on telecommunications and signal processing
(TSP), pages 601–604. IEEE.
Rakugi, H., Sugimoto, K., Arai, H., Kozaki, K., Matsui, Y.,
Mizukami, K., Ohyagi, Y., Okochi, J., and Akishita,
M. (2022). Statement on falls in long-term care fa-
cilities by the japan geriatrics society and the japan
association of geriatric health services facilities.
Data Acquisition System for a Wearable-Based Fall Prevention
709