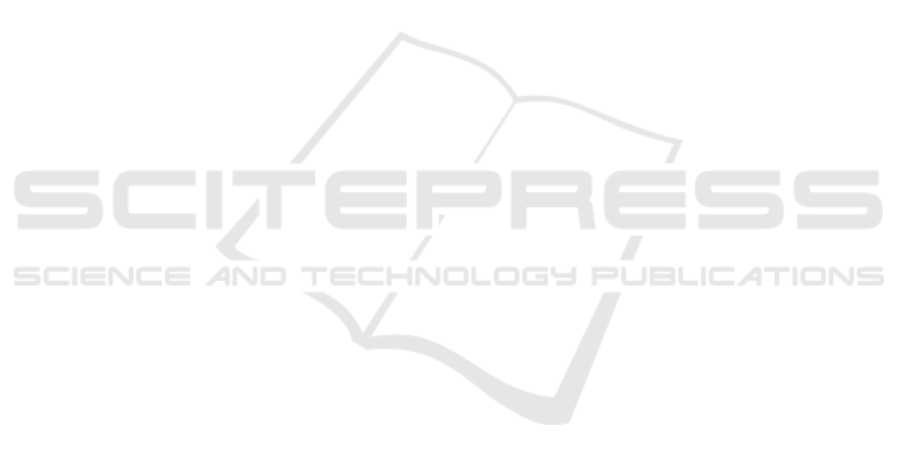
activity recognition. In Proceedings of the 15th Inter-
national Joint Conference on Biomedical Engineering
Systems and Technologies - Volume 4: BIOSIGNALS,
pages 40–49.
Hartmann, Y., Liu, H., and Schultz, T. (2020). Feature space
reduction for multimodal human activity recognition.
In Proceedings of the 13th International Joint Confer-
ence on Biomedical Engineering Systems and Tech-
nologies - Volume 4: BIOSIGNALS, pages 135–140.
INSTICC, SciTePress.
Hartmann, Y., Liu, H., and Schultz, T. (2021). Feature
space reduction for human activity recognition based
on multi-channel biosignals. In Proceedings of the
14th International Joint Conference on Biomedical
Engineering Systems and Technologies, pages 215–
222. INSTICC, SciTePress.
Hartmann, Y., Liu, H., and Schultz, T. (2022b). Interactive
and interpretable online human activity recognition.
In 2022 IEEE International Conference on Perva-
sive Computing and Communications Workshops and
other Affiliated Events (PerCom Workshops), pages
109–111. IEEE.
Hu, B., Rouse, E., and Hargrove, L. (2018). Benchmark
datasets for bilateral lower-limb neuromechanical sig-
nals from wearable sensors during unassisted locomo-
tion in able-bodied individuals. Frontiers in Robotics
and AI, 5:14.
Kuo, S. M., Lee, B. H., and Tian, W. (2013). Real-time digi-
tal signal processing: fundamentals, implementations
and applications. John Wiley & Sons.
Lara, O. D. and Labrador, M. A. (2012). A survey
on human activity recognition using wearable sen-
sors. IEEE communications surveys & tutorials,
15(3):1192–1209.
Liu, H. (2021). Biosignal Processing and Activity Model-
ing for Multimodal Human Activity Recognition. PhD
thesis, Universit
¨
at Bremen.
Liu, H., Gamboa, H., and Schultz, T. (2023). Sensor-
based human activity and behavior research: Where
advanced sensing and recognition technologies meet.
Sensors, 23(1):125.
Liu, H., Hartmann, Y., and Schultz, T. (2021a). CSL-
SHARE: A multimodal wearable sensor-based human
activity dataset. Frontiers in Computer Science, 3:90.
Liu, H., Hartmann, Y., and Schultz, T. (2021b). Mo-
tion Units: Generalized sequence modeling of hu-
man activities for sensor-based activity recognition. In
EUSIPCO 2021 — 29th European Signal Processing
Conference, pages 1506–1510. IEEE.
Liu, H., Hartmann, Y., and Schultz, T. (2022a). A practi-
cal wearable sensor-based human activity recognition
research pipeline. In Proceedings of the 15th Inter-
national Joint Conference on Biomedical Engineering
Systems and Technologies - Volume 5: HEALTHINF,
pages 847–856.
Liu, H., Jiang, K., Gamboa, H., Xue, T., and Schultz, T.
(2022b). Bell shape embodying zhongyong: The pitch
histogram of traditional chinese anhemitonic penta-
tonic folk songs. Applied Sciences, 12(16):8343.
Liu, H. and Schultz, T. (2018). ASK: A framework for data
acquisition and activity recognition. In Proceedings of
the 11th International Joint Conference on Biomedi-
cal Engineering Systems and Technologies - Volume 3:
BIOSIGNALS, pages 262–268. INSTICC, SciTePress.
Liu, H. and Schultz, T. (2019). A wearable real-time hu-
man activity recognition system using biosensors in-
tegrated into a knee bandage. In Proceedings of the
12th International Joint Conference on Biomedical
Engineering Systems and Technologies - Volume 1:
BIODEVICES, pages 47–55. INSTICC, SciTePress.
Liu, H. and Schultz, T. (2022). How long are various types
of daily activities? statistical analysis of a multimodal
wearable sensor-based human activity dataset. In Pro-
ceedings of the 15th International Joint Conference on
Biomedical Engineering Systems and Technologies -
Volume 5: HEALTHINF, pages 680–688.
Liu, H., Xue, T., and Schultz, T. (2022c). Merged pitch his-
tograms and pitch-duration histograms. In Proceed-
ings of the 19th International Conference on Signal
Processing and Multimedia Applications - SIGMAP,
pages 32–39. INSTICC, SciTePress.
Lukowicz, P., Ward, J. A., Junker, H., St
¨
ager, M., Tr
¨
oster,
G., Atrash, A., and Starner, T. (2004). Recognizing
workshop activity using body worn microphones and
accelerometers. In In Pervasive Computing, pages 18–
32.
Mekruksavanich, S., Jantawong, P., and Jitpattanakul, A.
(2022). A deep learning-based model for human ac-
tivity recognition using biosensors embedded into a
smart knee bandage. Procedia Computer Science,
214:621–627.
Micucci, D., Mobilio, M., and Napoletano, P. (2017).
UniMiB SHAR: A dataset for human activity recog-
nition using acceleration data from smartphones. Ap-
plied Sciences, 7(10):1101.
Naseeb, C. and Saeedi, B. A. (2020). Activity recogni-
tion for locomotion and transportation dataset using
deep learning. In Adjunct Proceedings of the 2020
ACM International Joint Conference on Pervasive and
Ubiquitous Computing and Proceedings of the 2020
ACM International Symposium on Wearable Comput-
ers, pages 329–334.
Palyaf
´
ari, R. (2015). Continuous activity recognition for an
intelligent knee orthosis; an out-of-lab study. Master’s
thesis, Karlsruher Institut f
¨
ur Technologie.
Rebelo, D., Amma, C., Gamboa, H., and Schultz, T. (2013).
Human activity recognition for an intelligent knee or-
thosis. In BIOSIGNALS 2013 - 6th International Con-
ference on Bio-inspired Systems and Signal Process-
ing, pages 368–371.
Rodrigues, J., Liu, H., Folgado, D., Belo, D., Schultz,
T., and Gamboa, H. (2022). Feature-based informa-
tion retrieval of multimodal biosignals with a self-
similarity matrix: Focus on automatic segmentation.
Biosensors, 12(12):1182.
Telaar, D., Wand, M., Gehrig, D., Putze, F., Amma, C.,
Heger, D., Vu, N. T., Erhardt, M., Schlippe, T.,
Janke, M., et al. (2014). Biokit—real-time decoder for
biosignal processing. In Fifteenth Annual Conference
of the International Speech Communication Associa-
tion.
Weiner, J., Diener, L., Stelter, S., Externest, E., K
¨
uhl, S.,
Herff, C., Putze, F., Schulze, T., Salous, M., Liu,
On a Real Real-Time Wearable Human Activity Recognition System
719