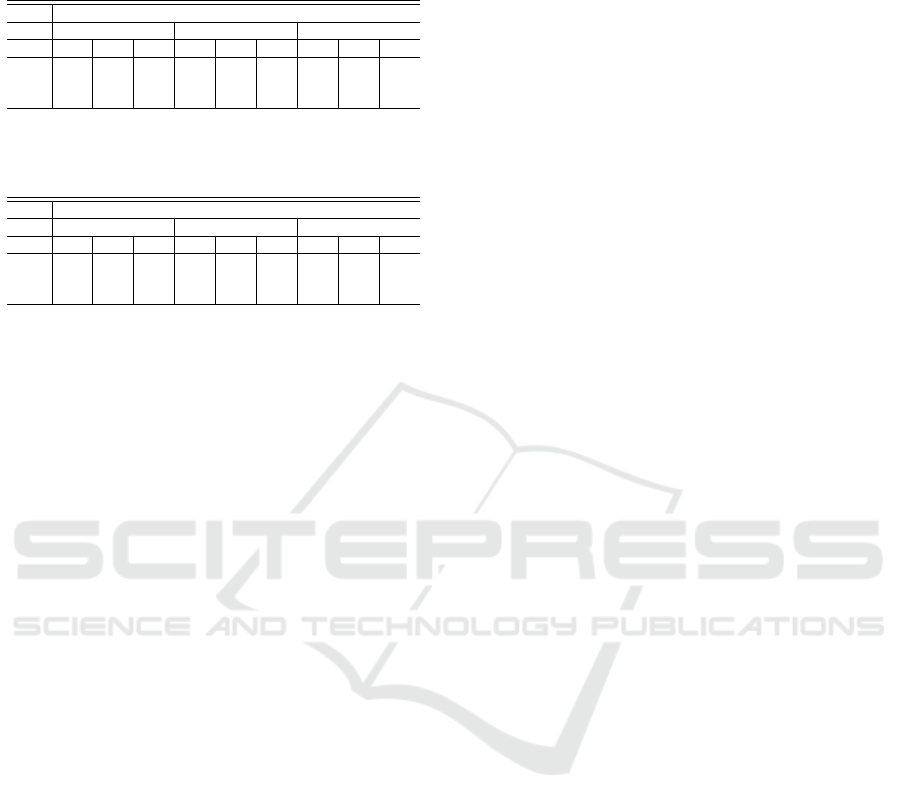
Table 10: Disaggregation risk of the common appliances in
the two synthetic datasets for Scenario 3. A = Original, B =
MDAV, C = DFTMicroagg. K = 40 and Coeff = 80.
DR - Synthetic REFIT and AMPd
ATE MPT VST
Load A B C A B C A B C
CWE 0.06 0.09 0.02 0.00 0.07 0.00 0.07 0.10 0.03
DWE 0.21 0.11 0.10 0.07 0.03 0.01 0.15 0.06 0.05
FRE 0.00 0.00 0.00 0.00 0.00 0.00 0.00 0.00 0.00
Table 11: Disaggregation risk of the common appliances in
the two synthetic datasets for Scenario 3. A = Original, B =
MDAV, C = DFTMicroagg. K = 50 and Coeff = 80.
DR - Synthetic REFIT and AMPd
ATE MPT VST
Load A B C A B C A B C
CWE 0.06 0.02 0.00 0.00 0.00 0.00 0.07 0.02 0.00
DWE 0.21 0.07 0.02 0.07 0.01 0.00 0.15 0.05 0.00
FRE 0.00 0.00 0.00 0.00 0.00 0.00 0.00 0.00 0.00
6 CONCLUSIONS
In this paper, we assessed the privacy levels of syn-
thetic households aggregate smart grid data. We in-
vestigated the performance of Seq2Seq energy dis-
aggregation algorithm and three activation extraction
methods. The findings revealed that the disclosure
risk associated with a significant number of appli-
ances in the synthetic aggregate data is high. There-
after, we proposed two privacy preserving approaches
to lower this disclosure risk. The results show that the
two privacy protection methods produced promising
results for privacy protection of individual household
lifestyles. In future, we would like to investigate the
privacy leakage at the top level of the grid hierarchy.
This will enable us to have a better understanding of
the privacy protection offer by the proposed mecha-
nisms at different levels of smart grid hierarchy.
ACKNOWLEDGEMENTS
This work was partially supported by the Wallen-
berg AI, Autonomous Systems and Software Program
(WASP) funded by the Knut and Alice Wallenberg
Foundation. The first author is supported by the
Kempe foundation.
REFERENCES
Adewole, K. S. and Torra, V. (2022a). Dftmicroagg: a dual-
level anonymization algorithm for smart grid data. In-
ternational Journal of Information Security, pages 1–
23.
Adewole, K. S. and Torra, V. (2022b). Privacy is-
sues in smart grid data: From energy disaggregation
to disclosure risk. In International Conference on
Database and Expert Systems Applications, pages 71–
84. Springer.
Batra, N., Kukunuri, R., Pandey, A., Malakar, R., Kumar,
R., Krystalakos, O., Zhong, M., Meira, P., and Par-
son, O. (2019). Towards reproducible state-of-the-
art energy disaggregation. In Proceedings of the 6th
ACM international conference on systems for energy-
efficient buildings, cities, and transportation, pages
193–202. ACM.
BBCNews (2017). Ukraine power cut ’was cyber-attack’.
https://www.bbc.com/news/technology-38573074.
Accessed: 18th May 2021.
Domingo-Ferrer, J. and Torra, V. (2005). Ordinal, continu-
ous and heterogeneous k-anonymity through microag-
gregation. Data Mining and Knowledge Discovery,
11(2):195–212.
El Kababji, S. and Srikantha, P. (2020). A data-driven
approach for generating synthetic load patterns and
usage habits. IEEE Transactions on Smart Grid,
11(6):4984–4995.
Fekri, M. N., Ghosh, A. M., and Grolinger, K. (2019).
Generating energy data for machine learning with re-
current generative adversarial networks. Energies,
13(1):130.
Hart, G. W., Kern Jr, E. C., and Schweppe, F. C. (1989).
Non-intrusive appliance monitor apparatus. US Patent
4,858,141.
Kelly, J. and Knottenbelt, W. (2015). Neural nilm: Deep
neural networks applied to energy disaggregation. In
Proceedings of the 2nd ACM international conference
on embedded systems for energy-efficient built envi-
ronments, pages 55–64. ACM.
Lopez, J. M. G., Pouresmaeil, E., Canizares, C. A., Bhat-
tacharya, K., Mosaddegh, A., and Solanki, B. V.
(2018). Smart residential load simulator for energy
management in smart grids. IEEE Transactions on In-
dustrial Electronics, 66(2):1443–1452.
Precioso, D. and Gomez-Ullate, D. (2020). Nilm as a re-
gression versus classification problem: the importance
of thresholding. arXiv preprint arXiv:2010.16050.
Samarati, P. (2001). Protecting respondents identities in mi-
crodata release. IEEE transactions on Knowledge and
Data Engineering, 13(6):1010–1027.
Sangogboye, F. C., Jia, R., Hong, T., Spanos, C., and
Kjærgaard, M. B. (2018). A framework for privacy-
preserving data publishing with enhanced utility for
cyber-physical systems. ACM Transactions on Sensor
Networks (TOSN), 14(3-4):1–22.
Soykan, E. U., Bilgin, Z., Ersoy, M. A., and Tomur, E.
(2019). Differentially private deep learning for load
forecasting on smart grid. In 2019 IEEE Globecom
Workshops (GC Wkshps), pages 1–6. IEEE.
SECRYPT 2023 - 20th International Conference on Security and Cryptography
286