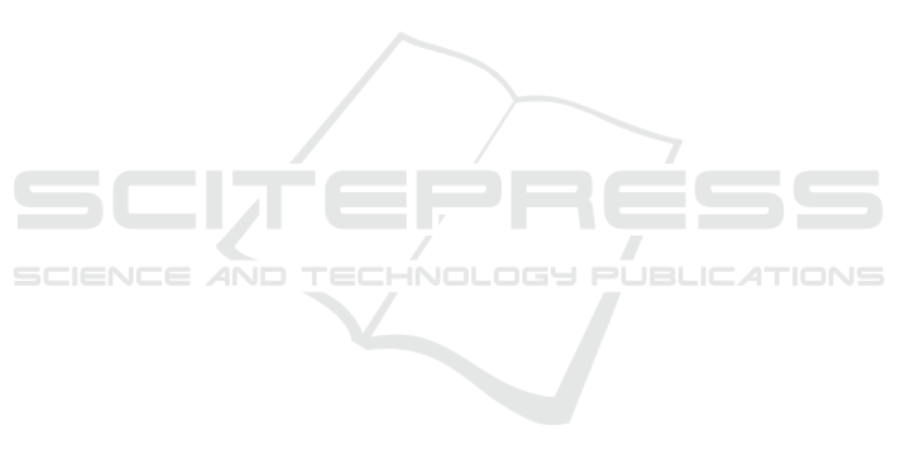
valid since they abide with the literature. Future work
will focus on providing weights to the features that
are manipulated so as for the method to provide rec-
ommendations ranked based on how easy these can be
implemented. For instance salary increase by 30 per-
cent might be more difficult to realise than increase in
company’s environmental conditions. In addition the
method will be extended to eliminate automatically
infeasible solutions by enabling the user to provide
range of feasible feature values. Future work will also
focus on comparing the results of our method with
similar work under different scenarios.
ACKNOWLEDGEMENTS
We gratefully acknowledge funding from the VW-
Foundation for the project IMPACT funded in the
frame of the funding line AI and its Implications for
Future Society.
REFERENCES
Artelt, A., Vaquet, V., Velioglu, R., Hinder, F., Brinkrolf,
J., Schilling, M., and Hammer, B. (2021). Evalu-
ating robustness of counterfactual explanations. In
2021 IEEE Symposium Series on Computational In-
telligence (SSCI), pages 01–09. IEEE.
Boyd, S., Boyd, S. P., and Vandenberghe, L. (2004). Convex
optimization. Cambridge university press.
Gao, J., Wang, X., Wang, Y., Yan, Y., and Xie, X. (2021).
Learning groupwise explanations for black-box mod-
els. In IJCAI, pages 2396–2402.
Guidotti, R., Monreale, A., Ruggieri, S., Turini, F., Gian-
notti, F., and Pedreschi, D. (2018). A survey of meth-
ods for explaining black box models. ACM Comput.
Surv., 51(5).
Haldorai, K., Kim, W. G., Pillai, S. G., Park, T. E., and
Balasubramanian, K. (2019). Factors affecting ho-
tel employees’ attrition and turnover: Application of
pull-push-mooring framework. International Journal
of Hospitality Management, 83:46–55.
Hancox-Li, L. (2020). Robustness in machine learning
explanations: does it matter? In Proceedings of
the 2020 conference on fairness, accountability, and
transparency, pages 640–647.
IBM (2020). Ibm hr analytics employee.
https://www.kaggle.com/pavansubhasht/ibm-hr-
analytics-attrition-dataset.
Kanamori, K., Takagi, T., Kobayashi, K., and Ike, Y.
(2022). Counterfactual explanation trees: Transparent
and consistent actionable recourse with decision trees.
In International Conference on Artificial Intelligence
and Statistics, pages 1846–1870. PMLR.
Kang, I. G., Croft, B., and Bichelmeyer, B. A. (2021). Pre-
dictors of turnover intention in us federal government
workforce: Machine learning evidence that perceived
comprehensive hr practices predict turnover intention.
Public Personnel Management, 50(4):538–558.
Le, H., Lee, J., Nielsen, I., and Nguyen, T. L. A. (2022).
Turnover intentions: the roles of job satisfaction and
family support. Personnel Review, (ahead-of-print).
Linardatos, P., Papastefanopoulos, V., and Kotsiantis, S.
(2020). Explainable ai: A review of machine learn-
ing interpretability methods. Entropy, 23(1):18.
Lundberg, S. M. and Lee, S.-I. (2017). A unified approach
to interpreting model predictions. Advances in neural
information processing systems, 30.
Mishra, S., Dutta, S., Long, J., and Magazzeni, D.
(2021). A survey on the robustness of feature impor-
tance and counterfactual explanations. arXiv preprint
arXiv:2111.00358.
Molnar, C. (2020). Interpretable machine learning. Lulu.
com.
Rawal, K. and Lakkaraju, H. (2020). Beyond individualized
recourse: Interpretable and interactive summaries of
actionable recourses. Advances in Neural Information
Processing Systems, 33:12187–12198.
Ribeiro, M. T., Singh, S., and Guestrin, C. (2016). ” why
should i trust you?” explaining the predictions of any
classifier. In Proceedings of the 22nd ACM SIGKDD
international conference on knowledge discovery and
data mining, pages 1135–1144.
Slack, D., Hilgard, A., Lakkaraju, H., and Singh, S. (2021).
Counterfactual explanations can be manipulated. Ad-
vances in Neural Information Processing Systems,
34:62–75.
Verma, S., Dickerson, J., and Hines, K. (2020). Counter-
factual explanations for machine learning: A review.
arXiv preprint arXiv:2010.10596.
Wachter, S., Mittelstadt, B., and Russell, C. (2017). Coun-
terfactual explanations without opening the black box:
Automated decisions and the gdpr. Harv. JL & Tech.,
31:841.
Zhao, Y., Hryniewicki, M. K., Cheng, F., Fu, B., and Zhu,
X. (2018). Employee turnover prediction with ma-
chine learning: A reliable approach. In Proceedings
of SAI intelligent systems conference, pages 737–758.
Springer.
ICEIS 2023 - 25th International Conference on Enterprise Information Systems
538