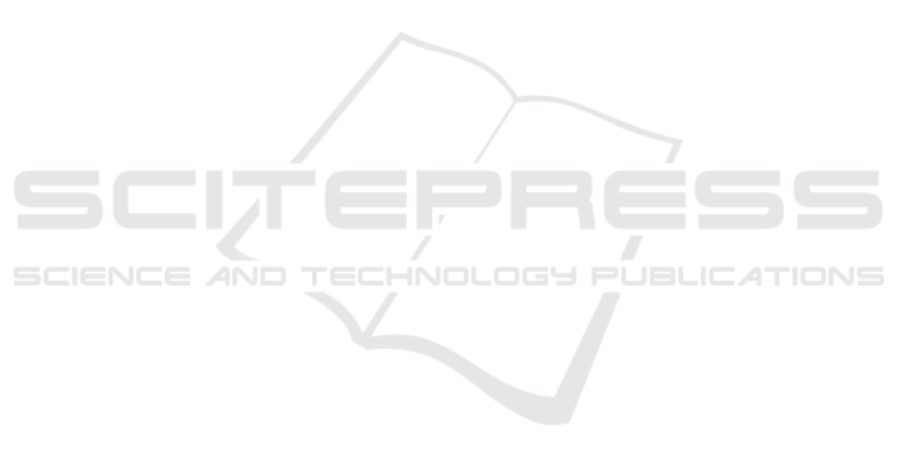
Cafarelli, D., Ciampi, L., Vadicamo, L., Gennaro, C.,
Berton, A., Paterni, M., Benvenuti, C., Passera, M.,
and Falchi, F. (2022). MOBDrone: A drone video
dataset for man OverBoard rescue. In Image Anal-
ysis and Processing – ICIAP 2022, pages 633–644.
Springer International Publishing.
Chen, Y., Li, W., Chen, X., and Gool, L. V. (2019). Learn-
ing semantic segmentation from synthetic data: A ge-
ometrically guided input-output adaptation approach.
In 2019 IEEE/CVF Conference on Computer Vision
and Pattern Recognition (CVPR). IEEE.
Cheng, M., Cai, K., and Li, M. (2021). RWF-2000: An
open large scale video database for violence detec-
tion. In 2020 25th International Conference on Pat-
tern Recognition (ICPR). IEEE.
Ciampi, L., Amato, G., Falchi, F., Gennaro, C., and Rabitti,
F. (2018). Counting vehicles with cameras. In Pro-
ceedings of the 26th Italian Symposium on Advanced
Database Systems, Castellaneta Marina (Taranto),
Italy, June 24-27, 2018, volume 2161 of CEUR Work-
shop Proceedings. CEUR-WS.org.
Ciampi, L., Foszner, P., Messina, N., Staniszewski, M.,
Gennaro, C., Falchi, F., Serao, G., Cogiel, M., Golba,
D., Szcz˛esna, A., and Amato, G. (2022a). Bus vio-
lence: An open benchmark for video violence detec-
tion on public transport. Sensors, 22(21):8345.
Ciampi, L., Gennaro, C., Carrara, F., Falchi, F., Vairo, C.,
and Amato, G. (2022b). Multi-camera vehicle count-
ing using edge-AI. Expert Systems with Applications,
207:117929.
Ciampi, L., Messina, N., Falchi, F., Gennaro, C., and Am-
ato, G. (2020a). Virtual to real adaptation of pedes-
trian detectors. Sensors, 20(18):5250.
Ciampi, L., Santiago, C., Costeira, J., Gennaro, C., and
Amato, G. (2021). Domain adaptation for traffic den-
sity estimation. In Proceedings of the 16th Interna-
tional Joint Conference on Computer Vision, Imag-
ing and Computer Graphics Theory and Applications.
SCITEPRESS - Science and Technology Publications.
Ciampi, L., Santiago, C., Costeira, J. P., Gennaro, C., and
Amato, G. (2020b). Unsupervised vehicle counting
via multiple camera domain adaptation. In Proceed-
ings of the First International Workshop on New Foun-
dations for Human-Centered AI (NeHuAI) co-located
with 24th European Conference on Artificial Intelli-
gence (ECAI 2020), Santiago de Compostella, Spain,
September 4, 2020, volume 2659 of CEUR Workshop
Proceedings, pages 82–85. CEUR-WS.org.
Csurka, G. (2017). Domain adaptation for visual
applications: A comprehensive survey. CoRR,
abs/1702.05374.
Deng, J., Dong, W., Socher, R., Li, L.-J., Li, K., and Fei-Fei,
L. (2009). ImageNet: A large-scale hierarchical im-
age database. In 2009 IEEE Conference on Computer
Vision and Pattern Recognition. IEEE.
Feichtenhofer, C., Fan, H., Malik, J., and He, K. (2019).
SlowFast networks for video recognition. In 2019
IEEE/CVF International Conference on Computer Vi-
sion (ICCV). IEEE.
Feichtenhofer, C., Pinz, A., and Wildes, R. P. (2016). Spa-
tiotemporal residual networks for video action recog-
nition. In Advances in Neural Information Processing
Systems 29: Annual Conference on Neural Informa-
tion Processing Systems 2016, December 5-10, 2016,
Barcelona, Spain, pages 3468–3476.
Ganin, Y., Ustinova, E., Ajakan, H., Germain, P.,
Larochelle, H., Laviolette, F., Marchand, M., and
Lempitsky, V. S. (2016). Domain-adversarial training
of neural networks. J. Mach. Learn. Res., 17:59:1–
59:35.
Hanson, A., PNVR, K., Krishnagopal, S., and Davis, L.
(2019). Bidirectional convolutional LSTM for the de-
tection of violence in videos. In Lecture Notes in Com-
puter Science, pages 280–295. Springer International
Publishing.
He, K., Zhang, X., Ren, S., and Sun, J. (2016). Deep resid-
ual learning for image recognition. In 2016 IEEE Con-
ference on Computer Vision and Pattern Recognition
(CVPR). IEEE.
Hong, W., Wang, Z., Yang, M., and Yuan, J. (2018). Con-
ditional generative adversarial network for structured
domain adaptation. In 2018 IEEE/CVF Conference on
Computer Vision and Pattern Recognition. IEEE.
Huo, X., Xie, L., Hu, H., Zhou, W., Li, H., and Tian, Q.
(2022). Domain-agnostic prior for transfer seman-
tic segmentation. In 2022 IEEE/CVF Conference on
Computer Vision and Pattern Recognition (CVPR).
IEEE.
Jin, Y., Wang, X., Long, M., and Wang, J. (2020). Mini-
mum class confusion for versatile domain adaptation.
In Computer Vision – ECCV 2020, pages 464–480.
Springer International Publishing.
Lin, T.-Y., Maire, M., Belongie, S., Hays, J., Perona, P., Ra-
manan, D., Dollár, P., and Zitnick, C. L. (2014). Mi-
crosoft COCO: Common objects in context. In Com-
puter Vision – ECCV 2014, pages 740–755. Springer
International Publishing.
Liu, Z., Lin, Y., Cao, Y., Hu, H., Wei, Y., Zhang, Z., Lin,
S., and Guo, B. (2021). Swin transformer: Hierarchi-
cal vision transformer using shifted windows. In Pro-
ceedings of the IEEE/CVF International Conference
on Computer Vision, pages 10012–10022.
Liu, Z., Ning, J., Cao, Y., Wei, Y., Zhang, Z., Lin, S., and
Hu, H. (2022). Video swin transformer. In Proceed-
ings of the IEEE/CVF Conference on Computer Vision
and Pattern Recognition, pages 3202–3211.
Padamwar, B. (2020). Violence detection in surveillance
video using computer vision techniques. International
Journal for Research in Applied Science and Engi-
neering Technology, 8(8):533–536.
Pan, S. J. and Yang, Q. (2010). A survey on transfer learn-
ing. IEEE Transactions on Knowledge and Data En-
gineering, 22(10):1345–1359.
P˛eszor, D., Staniszewski, M., and Wojciechowska, M.
(2016). Facial reconstruction on the basis of video
surveillance system for the purpose of suspect identi-
fication. In Intelligent Information and Database Sys-
tems, pages 467–476. Springer Berlin Heidelberg.
Shi, X., Chen, Z., Wang, H., Yeung, D., Wong, W., and
Woo, W. (2015). Convolutional LSTM network: A
Unsupervised Domain Adaptation for Video Violence Detection in the Wild
45