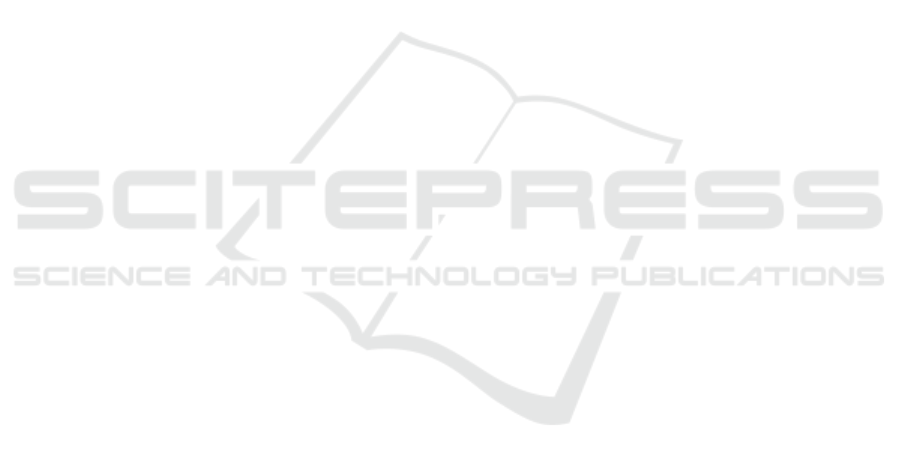
troller. For example, errors could be minimized, if the
laser power is reduced by the time of a warning, or at
least if an error occurs. This would likely lead to an
improved BP quality and thus should be investigated.
Additionally, machine learning should be used to in-
tegrate more parts of a complete digital twin, such as
geometry optimization and material planning. Sum-
marizing, the presented approach is a vital proof of
concept for the feasibility of moving AI into practice
without too many expenses and labour time.
ACKNOWLEDGEMENTS
All results presented refer to the TWIN project
(https://websites.fraunhofer.de/TWIN), funded by the
Federal Ministry of Education and Research, Ger-
many (02K18D052).
REFERENCES
Akbari, P., Ogoke, F., Kao, N.-Y., Meidani, K., Yeh, C.-Y.,
Lee, W., and Farimani, A. B. (2022). MeltpoolNet:
Melt pool characteristic prediction in metal additive
manufacturing using machine learning. Additive Man-
ufacturing, 55:102817.
Bauer, M., Augenstein, C., Sch
¨
afer, M., and Theile, O.
(2022). Artificial intelligence in laser powder bed fu-
sion procedures – neural networks for live-detection
and forecasting of printing failures. Procedia CIRP,
107:1367–1372. Leading manufacturing systems
transformation – Proceedings of the 55th CIRP Con-
ference on Manufacturing Systems 2022.
Bel-Hadj, Y. and Weijtjens, W. (2023). Anomaly detection
in vibration signals for structural health monitoring
of an offshore wind turbine. In Rizzo, P. and Mi-
lazzo, A., editors, European Workshop on Structural
Health Monitoring, volume 270 of Lecture Notes in
Civil Engineering, pages 348–358. Springer Interna-
tional Publishing, Cham.
Cai, S., Wang, Z., Wang, S., Perdikaris, P., and Karniadakis,
G. E. (2021). Physics-informed neural networks for
heat transfer problems. Journal of Heat Transfer,
143(6).
Deng, J., Dong, W., Socher, R., Li, L.-J., Li, K., and Fei-
Fei, L. (2009). Imagenet: a large-scale hierarchi-
cal image database. In 2009 IEEE conference on com-
puter vision and pattern recognition, pages 248–255.
IEEE.
Gaikwad, A., Giera, B., Guss, G. M., Forien, J.-B.,
Matthews, M. J., and Rao, P. (2020). Heterogeneous
sensing and scientific machine learning for quality as-
surance in laser powder bed fusion – a single-track
study. Additive Manufacturing, 36:101659.
Gaikwad, A., Williams, R. J., de Winton, H., Bevans, B. D.,
Smoqi, Z., Rao, P., and Hooper, P. A. (2022). Multi
phenomena melt pool sensor data fusion for enhanced
process monitoring of laser powder bed fusion addi-
tive manufacturing. Materials & Design, 221:110919.
He, K., Zhang, X., Ren, S., and Sun, J. (2016). Deep resid-
ual learning for image recognition. In 2016 IEEE Con-
ference on Computer Vision and Pattern Recognition
(CVPR). IEEE.
Howard, A. G., Zhu, M., Chen, B., Kalenichenko, D.,
Wang, W., Weyand, T., Andreetto, M., and Adam,
H. (2017). Mobilenets: Efficient convolutional neu-
ral networks for mobile vision applications.
Knaak, C., Masseling, L., Duong, E., Abels, P., and Gill-
ner, A. (2021). Improving build quality in laser pow-
der bed fusion using high dynamic range imaging and
model-based reinforcement learning. IEEE Access,
9:55214–55231.
Kunkel, M. H., Gebhardt, A., Mpofu, K., and Kallweit, S.
(2019). Quality assurance in metal powder bed fusion
via deep-learning-based image classification. Rapid
Prototyping Journal, 26(2):259–266.
Kwon, O., Kim, H. G., Ham, M. J., Kim, W., Kim, G.-H.,
Cho, J.-H., Kim, N. I., and Kim, K. (2018). A deep
neural network for classification of melt-pool images
in metal additive manufacturing. Journal of Intelligent
Manufacturing, 31(2):375–386.
Mukherjee, T., Wei, H. L., De, A., and DebRoy, T. (2018).
Heat and fluid flow in additive manufacturing—part
i: Modeling of powder bed fusion. Computational
Materials Science, 150:304–313.
Ngairangbam, V. S., Spannowsky, M., and Takeuchi, M.
(2022). Anomaly detection in high-energy physics
using a quantum autoencoder. Physical Review D,
105(9).
Praveena, B., Lokesh, N., Abdulrajak, B., Santhosh, N.,
Praveena, B. L., and Vignesh, R. (2022). A compre-
hensive review of emerging additive manufacturing
(3d printing technology): Methods, materials, appli-
cations, challenges, trends and future potential. Mate-
rials Today: Proceedings, 52:1309–1313.
Raissi, M., Perdikaris, P., and Karniadakis, G. E. (2019).
Physics-informed neural networks: A deep learn-
ing framework for solving forward and inverse prob-
lems involving nonlinear partial differential equations.
Journal of Computational Physics, 378:686–707.
Shen, B., Lu, J., Wang, Y., Chen, D., Han, J., Zhang, Y., and
Zhao, Z. (2022). Multimodal-based weld reinforce-
ment monitoring system for wire arc additive manu-
facturing. Journal of Materials Research and Tech-
nology, 20:561–571.
Tan, M. and Le, Q. (2019). EfficientNet: Rethinking model
scaling for convolutional neural networks. In Chaud-
huri, K. and Salakhutdinov, R., editors, Proceedings of
the 36th International Conference on Machine Learn-
ing, volume 97 of Proceedings of Machine Learning
Research, pages 6105–6114. PMLR.
Zhu, Q., Liu, Z., and Yan, J. (2021). Machine learn-
ing for metal additive manufacturing: predicting tem-
perature and melt pool fluid dynamics using physics-
informed neural networks. Computational Mechanics,
67(2):619–635.
ICEIS 2023 - 25th International Conference on Enterprise Information Systems
546