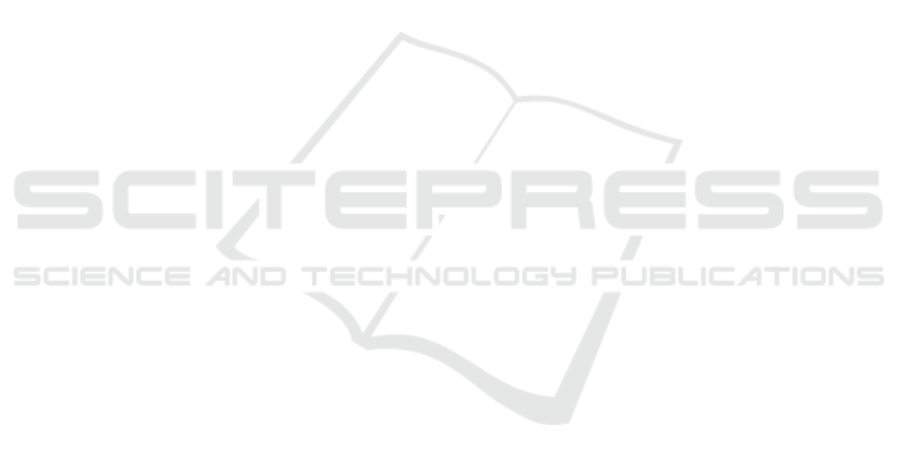
gions which exhibit a strong causal link between ex-
treme climate variations and conflict (predominantly
in Africa and South Asia), and 3. there exists a com-
mon time lag of the causality between the climate
variations and the conflict in many regions, which is
worth further study. In order to identify the spurious
causality in our results from pure data analysis per-
spective, we proposed to embed the knowledge from
social science into our initial assumptions about the
mechanisms within climate and conflict.
ACKNOWLEDGEMENTS
We acknowledge funding from the Alan Turing In-
stitute via the Defence and Securities Program, and
valuable advice and help given by the previous pro-
gram director Prof. Mark Briers.
REFERENCES
Buhaug, H. (2010). Climate not to blame for african civil
wars. In Proc. National Academy of Sciences (PNAS).
Burke, M. B., Miguel, E., Satyanath, S., Dykema, J. A.,
and Lobell, D. B. (2009). Warming increases the risk
of civil war in africa. In Proc. National Academy of
Sciences (PNAS).
Granger, C. W. (1969). nvestigating causal relations by
econometric models and cross-spectral methods. In
Econometrica: journal of the Econometric Society.
Guo, W., Gleditsch, K., and Wilson, A. (2018). Retool AI
to forecast and limit wars. In Nature.
Hlavackova-Schindler, K., Palus, M., Vejmelka, M., and
Bhattacharya, J. (2007). Causality detection based on
information-theoretic approaches in time series analy-
sis. In Physical Reports.
Homer-Dixon, T. (2010). Environment, scarcity, and vio-
lence. Princeton University Press.
Hsiang, S. M. and Burke, M. (2014). Climate, conflict, and
social stability: what does the evidence say? In Cli-
matic Change.
Hsiang, S. M., Meng, K. C., and Cane, M. A. (2011). Civil
conflicts are associated with the global climate. In
Nature.
Krakovska, A. (2017). Predictability improvement as a tool
to detect causality. In International Conference on
Measurement.
Krakovska, A. and Hanzely, F. (2016). Testing for causality
in reconstructed state spaces by an optimized mixed
prediction method. In Physical Review E.
Krakovska, A. and Jakubik, J. (2020). Implementation of
two causal methods based on predictions in recon-
structed state space. In Physical Review E.
Krakovska, A., Jakubik, J., Chvostekova, M., Coufal, D.,
Jajcay, N., and Palus, M. (2018). Comparison of six
methods for the detection of causality in a bivariate
time series. In Physical Review E.
Li, X. and Ouyang, W. (2010). Estimating coupling direc-
tion between neuronal populations with permutation
conditional mutual information. In NeuroImage.
Mach, K. J., Kraan, C. M., Adger, W. N., Buhaug, H.,
Burke, M., Fearon, J. D., Field, C., Maystadt, J.-F.,
and O’Loughlin, J. (2019). Climate as a risk factor for
armed conflict. In Nature.
Nes, E. H. V., Scheffer, M., Brovkin, V., Lenton, T. M., Ye,
H., Deyle, E., and Sugihara, G. (2015). Causal feed-
backs in climate change. In Nature Climate Change.
Salehyan, I. (2014). Climate change and conflict: Making
sense of disparate findings. In Political Geography.
Slettebak, R. T. (2012). Don?t blame the weather! climate-
related natural disasters and civil conflict. In Journal
of Peace Research.
Sugihara, G., May, R., Ye, H., h. Hsieh, C., Deyle, E., Fog-
arty, M., and Munch, S. (2012). Detecting causality in
complex ecosystems. In Science.
Sun, S. C., Jin, B., Wei, Z., and Guo, W. (2022). Revealing
the excitation causality between climate and political
violence via a neural forward-intensity poisson pro-
cess. In International Joint Congress on AI (IJCAI).
Tsonis, A., Deyle, E. R., Ye, H., and Sugihara, G. (2018).
Convergent cross mapping: theory and an example. In
Advances in nonlinear geosciences.
Wang, X., Piao, S., Ciais, P., Friedlingstein, P., Myneni,
R. B., Cox, P., Heimann, M., Miller, J., Peng, S., and
Wang, T. (2014). A two-fold increase of carbon cy-
cle sensitivity to tropical temperature variations. In
Nature.
Wang, Y., Yang, J., Chen, Y., P. De Maeyer, Z. L., and Duan,
W. (2018). Detecting the causal effect of soil moisture
on precipitation using convergent cross mapping. In
Nature Scientific reports.
Wen, D., Jia, P., Hsu, S.-H., Zhou, Y., Lan, X., Cui, D., Li,
G., Yin, S., and Wang, L. (2019). Estimating coupling
strength between multivariate neural series with mul-
tivariate permutation conditional mutual information.
In Neural Networks.
COMPLEXIS 2023 - 8th International Conference on Complexity, Future Information Systems and Risk
76