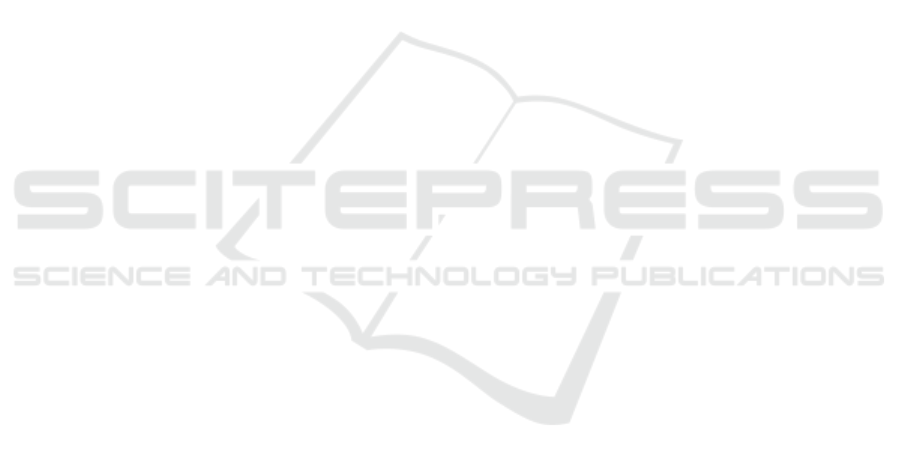
REFERENCES
Barron, J. T. and Malik, J. (2014). Shape, illumination, and
reflectance from shading. IEEE Trans. Pattern Anal.
Mach. Intell., 37:1670–1687.
Barrow, H., Tenenbaum, J., Hanson, A., and Riseman, E.
(1978). Recovering intrinsic scene characteristics.
Comput. Vision Syst., 2:2.
Bell, S., Bala, K., and Snavely, N. (2014). Intrinsic images
in the wild. ACM Trans. Graph., 33:1–12.
Bonneel, N., Kovacs, B., Paris, S., and Bala, K. (2017).
Intrinsic decompositions for image editing. Com put.
Graph. Forum, 36:593–609.
Chen, Q. and Koltun, V. (2013). A simple model for intrinsic
image decomposition with depth cues. In ICCV, pages
241–248, Sydney, NSW, Australia. IEEE.
Ding, Y. (2018). Image quality assessment based on human
visual system properties. Vis. Qual. Assessment Natu-
ral Med. Image, 37:63–106.
Ebner, M. (2007). Color Constancy, 1st ed. Wiley Publish-
ing, ISBN: 0470058299.
Ebner, M., Tischler, G., and Albert, J. (2007). Integrating
color constancy into JPEG2000. IEEE Trans. Image
Process., 16:2697–2706.
Gao, X., Lu, W., Tao, D., and Li, X. (2010). Image quality
assessment and human visual system. In Proc. Vis.
Commun. Image Process., pages 316–325, Huang-
shan, China. SPIE.
Garces, E., Rodriguez-Pardo, C., Casas, D., and Lopez-
Moreno, J. (2022). A survey on intrinsic images: Delv-
ing deep into lambert and beyond. Int. J. Comput. Vi-
sion, 130:836–868.
Gonzalez, R. C. and Woods, R. E. (2018). Digital Image
Processing, 3rd ed. Pearson Prentice Hall.
Grosse, R., Johnson, M. K., Adelson, E. H., and Freeman,
W. T. (2009). Ground truth dataset and baseline eval-
uations for intrinsic image algorithms. In ICCV, pages
2335–2342, Kyoto, Japan. IEEE.
Land, E. H. (1964). The retinex. Amer. Scientist, 52:247–
264.
Lettry, L., Vanhoey, K., and Van Gool, L. (2018). Unsuper-
vised deep single-image intrinsic decomposition us-
ing illumination-varying image sequences. Comput.
Graph. Forum, 37:409–419.
Li, Z., Yu, T.-W., Sang, S., Wang, S., Song, M., Liu, Y., Yeh,
Y.-Y., Zhu, R., Gundavarapu, N., Shi, J., Bi, S., Yu, H.-
X., Xu, Z., Sunkavalli, K., Hasan, M., Ramamoorthi,
R., and Chandraker, M. (2021). Openrooms: An open
framework for photorealistic indoor scene datasets. In
CVPR, pages 7190–7199, Nashville, TN, USA. IEEE.
Luo, M. R., Cui, G., and Rigg, B. (2001). The devel-
opment of the CIE 2000 colour-difference formula:
CIEDE2000. Color Res. Appl., 26:340–350.
Narihira, T., Maire, M., and Yu, S. X. (2015). Direct in-
trinsics: Learning albedo-shading decomposition by
convolutional regression. In ICCV, pages 2992–2992,
Santiago, Chile. IEEE.
Ren, X., Yang, W., Cheng, W.-H., and Liu, J. (2020). LR3M:
Robust low-light enhancement via low-rank regular-
ized retinex model. IEEE Trans. Image Process.,
29:5862–5876.
Roberts, M., Ramapuram, J., Ranjan, A., Kumar, A.,
Bautista, M. A., Paczan, N., Webb, R., and Susskind,
J. M. (2021). Hypersim: A photorealistic synthetic
dataset for holistic indoor scene understanding. In
ICCV, pages 10912–10922, Montreal, QC, Canada.
IEEE.
Sharma, G., Wu, W., and Dalal, E. N. (2005). The
CIEDE2000 color-difference formula: Implementa-
tion notes, supplementary test data, and mathematical
observations. Color Res. Appl., 30:21–30.
Sheikh, H. R. and Bovik, A. C. (2006). Image informa-
tion and visual quality. IEEE Trans. Image Process.,
15:430–444.
Shen, J., Yang, X., Jia, Y., and Li, X. (2011). Intrinsic im-
ages using optimization. In CVPR, pages 3481–3487,
Colorado Springs, CO, USA. IEEE.
Shi, J., Dong, Y., Su, H., and Yu, S. X. (2017). Learning
non-lambertian object intrinsics across shapenet cat-
egories. In CVPR, pages 1685–1694, Honolulu, HI,
USA. IEEE.
Ulucan, D., Ulucan, O., and Ebner, M. (2022a). IID-
NORD: A comprehensive intrinsic image decompo-
sition dataset. In ICIP, pages 2831–2835, Bordeaux,
France. IEEE.
Ulucan, O., Ulucan, D., and Ebner, M. (2022b). BIO-CC:
Biologically inspired color constancy. In BMVC, Lon-
don, UK. BMVA Press.
Ulucan, O., Ulucan, D., and Ebner, M. (2022c). Color con-
stancy beyond standard illuminants. In ICIP, pages
2826–2830, Bordeaux, France. IEEE.
Wang, Z., Bovik, A. C., Sheikh, H. R., and Simoncelli, E. P.
(2004). Image quality assessment: from error visibil-
ity to structural similarity. IEEE Trans. Image Pro-
cess., 13:600–612.
Wang, Z., Simoncelli, E. P., and Bovik, A. C. (2003). Mul-
tiscale structural similarity for image quality assess-
ment. In Proc. Asilomar Conf. Signals Syst. Comput.,
pages 1398–1402, Pacific Grove, CA, USA. IEEE.
Wimmer, M., Scherzer, D., and Purgathofer, W. (2004).
Light space perspective shadow maps. Rendering
Techn., 2004:143–151.
Zeki, S. (1993). A Vision of the Brain. Blackwell Science,
ISBN: 0632030545.
Zhang, L., Zhang, L., Mou, X., and Zhang, D. (2011).
FSIM: A feature similarity index for image quality
assessment. IEEE Trans. Image Process., 20:2378–
2386.
Zhao, Q., Tan, P., Dai, Q., Shen, L., Wu, E., and Lin, S.
(2012). A closed-form solution to retinex with non-
local texture constraints. IEEE Trans. Pattern Anal.
Mach. Intell., 34:1437–1444.
Zhu, W.-H., Sun, W., Min, X.-K., Zhai, G.-T., and Yang, X.-
K. (2021). Structured computational modeling of hu-
man visual system for no-reference image quality as-
sessment. Int. J. Automat. Comput., 18:204–218.
IMPROVE 2023 - 3rd International Conference on Image Processing and Vision Engineering
64