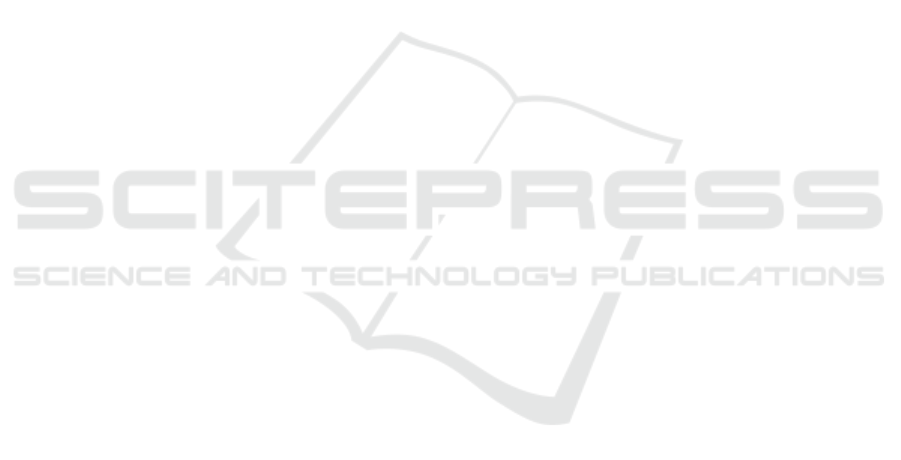
and select the most appropriate decision strategies.
These supports are necessary for making timely and
effective decisions.
This paper also intends to motivate further studies
in this context. One possible research direction is to
identify relevant decision-making contexts and ways
to elicit stakeholders’ perspectives on these contexts.
However, finding all the contexts and stakeholders re-
lated to an EA debt can be intricate, especially when
the EA landscape and management structure is large
and complex. Therefore, future research should de-
velop methods and tools to contextualize EA debts,
collect and organize relevant information from var-
ious sources, and bridge communication among the
relevant stakeholders. Following this, future research
should focus on developing ways to use the informa-
tion collected for meaningful assessments. Thus, we
recommend investigating the relationship between the
information pieces and existing debt-related key per-
formance indicators (KPI) and viewpoint-based ap-
proaches to assessing EA debt. Finally, future re-
search should develop practical ways to conclude the
prudence or recklessness of an EA debt based on the
assessments performed and to select appropriate con-
trol measures. Thus, we recommend exploring the
applicability of existing decision analysis approaches
and debt mitigation strategies to determine, e.g., when
to mitigate risks and reject the decision.
The second research direction is to evaluate the
proposed process in practice. As our evaluation re-
sult suggests, many aspects have yet to be considered
in the core design of the process, e.g., the financial
and project management aspects when evaluating the
prudence of EA debts. Thus, we recommend further
validation and development of the process to make it
more practical, concrete, and well-rounded for real-
world industrial scenarios through, e.g., performing a
series of workshops with various companies and ded-
icated experts. Such workshops can help identify the
unclear but needed aspects of the process.
The third research direction would be to consider
providing a tool to manage all the gathered informa-
tion in one place. Such a tool should classify the infor-
mation based on provided parameters and register the
relations between various information pieces, helping
to find needed information and make decisions.
REFERENCES
Becha, M., Dridi, O., Riabi, O., and Benmessaoud, Y.
(2020). Use of machine learning techniques in fi-
nancial forecasting. In 2020 International Multi-
Conference on: “Organization of Knowledge and Ad-
vanced Technologies” (OCTA).
Brenner, R. (2019). Balancing resources and load: Eleven
nontechnical phenomena that contribute to forma-
tion or persistence of technical debt. In 2019
IEEE/ACM International Conference on Technical
Debt (TechDebt).
Clair, K. (2016). Technical debt as an indicator of library
metadata quality. D-Lib Mag 22, 11/12.
Codabux, Z., Williams, B., Bradshaw, G., and Cantor, M.
(2017). An empirical assessment of technical debt
practices in industry. Journal of Software: Evolution
and Process 29, 10, page e1894.
Denton, M., Palmer, A., Masiello, R., and Skantze, P.
(2003). Managing market risk in energy. IEEE Trans-
actions on Power Systems 18, 2, pages 494–502.
Ernst, N., Bellomo, S., Ozkaya, I., Nord, R., and Gorton, I.
(2015). Measure it? manage it? ignore it? software
practitioners and technical debt. In Proceedings of the
2015 10th Joint Meeting on Foundations of Software
Engineering.
Fowler, M. (2009). Technical debt quadrant.
Hacks, S., Hofert, H., Salentin, J., Yeong, Y., and Lichter,
H. (2019). Towards the definition of enterprise
architecture debts. 2019 IEEE 23rd International
Enterprise Distributed Object Computing Workshop
(EDOCW).
Klinger, T., Tarr, P., Wagstrom, P., and Williams, C. (2011).
An enterprise perspective on technical debt. Proceed-
ing of the 2nd working on Managing technical debt -
MTD ’11.
Maqsood, A., Alexander, P., Lichter, H., and Tanachutiwat,
S. (2022). A viewpoints-based analysis of enterprise
architecture debt. In Wang, C.-C. and Nallanathan, A.,
editors, Proceedings of the 5th International Confer-
ence on Signal Processing and Information Communi-
cations, pages 133–154, Cham. Springer International
Publishing.
Matthes, F., Monahov, I., Schneider, A., and Schulz, C.
(2012). Eam kpi catalog v 1.0. Technical report, Tech-
nische Universit
¨
at M
¨
unchen, Munich, Germany.
Sas, D. and Avgeriou, P. (2019). Quality attribute trade-
offs in the embedded systems industry: an exploratory
case study. Software Quality Journal 28, 2, pages
505–534.
Silva, V., Jeronimo, H., and Travassos, G. (2018). Technical
debt management in brazilian software organizations.
In Proceedings of the 17th Brazilian Symposium on
Software Quality - SBQS.
Waltersdorfer, L., Rinker, F., Kathrein, L., and Biffl, S.
(2020). Experiences with technical debt and manage-
ment strategies in production systems engineering. In
Proceedings of the 3rd International Conference on
Technical Debt.
Wohlin, C. (2000). Experimentation in software engineer-
ing: An introduction / by Claes Wohlin ... [et al.], vol-
ume 6 of The Kluwer international series in software
engineering. Kluwer Academic, Boston and London.
Wolf, E. (2013). Stochastic simulation of optimal insurance
policies to manage supply chain risk. In 2013 Winter
Simulations Conference (WSC).
Zalewski, A. (2017). Risk appetite in architectural decision-
making. In 2017 IEEE International Conference on
Software Architecture Workshops (ICSAW).
ICEIS 2023 - 25th International Conference on Enterprise Information Systems
630