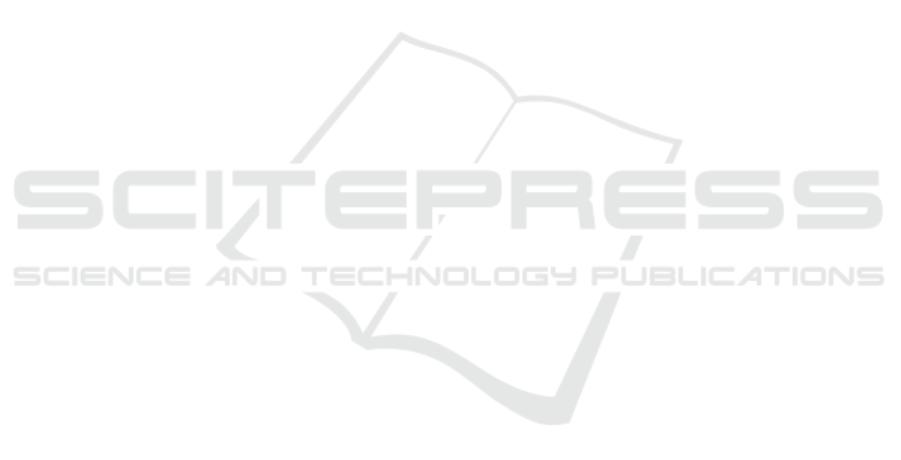
possess the ability to add structural and interpersonal
meaning. In our analysis, we used hashtags to distin-
guish between alternative narratives and mainstream
media narratives. For this task, we deployed two an-
notators who manually examined the list of hashtags
and labelled them as either “mainstream” or “alter-
native”. The annotators were guided by the list of
mainstream narratives that resulted from the news ar-
ticle inspection described above. For some hashtags,
the annotators inspected a random sample of tweets
containing the corresponding hashtags to decide on
classification of that label. This procedure resulted
in a substantial inter-rater agreement (Cohen Kappa
79.35%).
After the labels were revised and any discrepan-
cies between the two annotators resolved, the propor-
tion of alternative narratives in the data-set was 2.2%
for the Las Vegas shooting, 4.6% for YouTube HQ
shooting, 0.9% for El Paso Walmart shooting, and
0.1% for the Santa Fe school shooting.
Semantic and User Analysis. For our semantic anal-
ysis, we used the UDPipe R package (Straka and
Strakov
´
a, 2017) to carry out Part-of-Speech-Tagging
(POS), word frequency, and a co-occurrence analysis.
Furthermore, we created a hashtag co-occurrence net-
work and applied the Louvain-clustering algorithm to
obtain themes in the hashtags of the four events.
5 RESULTS
Semantic Analysis. First, we investigated the corre-
lation between proportional word frequencies among
alternative and mainstream narrative tweets. Pear-
son’s product-moment correlation sample estimate is
between 0.2347 for Santa Fe and 0.3051 for El Paso
which indicates a low correlation of word proportion
between the two narrative subsets. The proportion
of original messages (excluding retweets) among all
messages accounts to 17.0415% for alternative and
18.4892% for mainstream messages. We then car-
ried out a temporal analysis of alternative vs. main-
stream narratives in order to identify differences in
their prevalence. Figure 1 depicts the temporal (pro-
portional) prevalence of alternative and mainstream
narratives. The analysis indicated a time-lag in preva-
lence of alternative narratives which is intuitive as al-
ternative narratives do not include news updates dur-
ing the event. Across data-sets, we found peaks of
alternative tweets on certain days after an event. No
obvious connection to real-world incidents could be
identified for the peaks in alternative narratives.
As described above, we applied part-of-speech
tagging to investigate structural differences. Figure
2 depicts the proportional difference in prevalence
of universal part of speech categories between al-
ternative and mainstream narrative. For example,
in alternative narratives, the proportion of verbs is
1.59% higher than in mainstream narratives. More-
over, mainstream narratives include more pronouns,
prepositions (such as in, to, during) and adjectives
compared to alternative narratives. Interestingly, the
proportion of undefined tokens “X” was higher for al-
ternative narratives. The most frequent words in the
“X” category were: qanon, falseflag, george, good-
man, jason, webb, maga and wwg1wga (for our anal-
ysis, we excluded stop words such as lasvegasshoot-
ing).
To investigate the use of entities in alternative and
mainstream narratives, we analyzed the top 15 nouns
of each corpus and aggregated them in Figure 3. As
expected, the top alternative nouns were populated
by words associated with conspiracy theories, such
as ”wwg1wgaworldwide”, which stands for ”where
we go 1, we go all worldwide” and is used by Qanon
followers. “Fakenews”, “fakenewsmedia” and “msm”
(mainstream media) on the other hand accused main-
stream media of spreading misinformation to support
a hidden agenda. We also identified attempts to de-
clare the event as a “falseflag” attack carried out by
the government itself to cover-up for other actions to-
wards alleged secret goals.
Mainstream word analysis included references to
other shootings, labels such as “domestic terrorism”
and entities directly associated with the events (e.g.,
“walmart”, “victims” or “shootings”).
Furthermore, we carried out a co-occurrence anal-
ysis for each narrative and data-set. Co-occurrences
cover combinations of nouns and adjectives. Fig-
ure 4 depicts the 30 most prevalent combinations per
tweet. The number of items in each Figure varies, as
some terms might be included in multiple prevalent
combinations (e.g., for mainstream narratives for San
Bruno: gun - woman, gun - state).
When comparing word networks of alternative
narratives with mainstream narratives, positive words
that can be found in mainstream narratives (e.g.,
“prayer”, “thought”, “love”) cannot be found in the
word networks resulting from alternative narratives.
Also, across all events, we found alternative narra-
tives to be connected with conspiracy theories, such
as Qanon. Prevalent word combinations were, for ex-
ample, “deep state”, “qanon”, “george soros”. Inter-
estingly, alternative narratives often included names
of well-known individuals who were not directly con-
nected to an event itself (e.g., George Soros, Jason
Webb). This could not be observed for mainstream
narratives. We often found negative references to
Mainstream and Alternative Narratives in the Wake of Gun Shootings
21