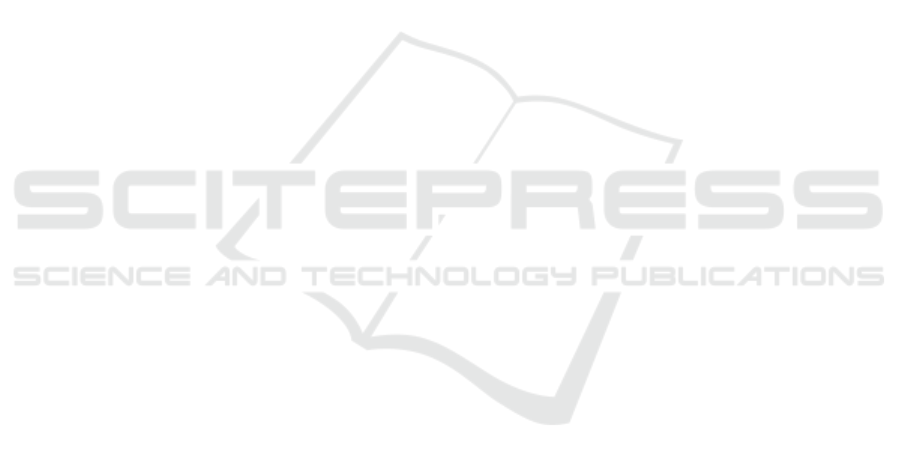
Han, J., Kamber, M., and Pei, J. (2012). Data mining con-
cepts and techniques, third edition. Morgan Kauf-
mann Publishers, 3rd edition.
Holzinger, A. (2018). From machine learning to explainable
ai. In Proc. of 2018 World Symp. on Digital Intell.
Syst. Mach., pages 55–66.
Kim, J., Comuzzi, M., Dumas, M., Maggi, F. M., and Teine-
maa, I. (2022). Encoding resource experience for
predictive process monitoring. Decis. Support Syst,
153:113669.
Maggi, F. M., Francescomarino, C. D., Dumas, M., and
Ghidini, C. (2014). Predictive monitoring of busi-
ness processes. In Int. Conf. Adv. Inf. Syst. Eng., pages
457–472.
Maisenbacher, M. and Weidlich, M. (2017). Handling con-
cept drift in predictive process monitoring. In Proc. of
2017 IEEE Int. Conf. Serv. Comput., pages 1–8.
M
´
arquez-Chamorro, A. E., Resinas, M., and Ruiz-Cort
´
es,
A. (2017). Predictive monitoring of business pro-
cesses: A survey. IEEE Trans. Serv. Comput.,
11(6):962–977.
Mehdiyev, N., Evermann, J., and Fettke, P. (2020). A novel
business process prediction model using a deep learn-
ing method. Bus. & Inf. Syst. Eng., 62(2):143–157.
Mehdiyev, N. and Fettke, P. (2021). Explainable artificial
intelligence for process mining: A general overview
and application of a novel local explanation approach
for predictive process monitoring. In Proc. of Inter-
pretable Artif. Intell.: A Perspective of Granular Com-
put., pages 1–28.
Polato, M., Sperduti, A., Burattin, A., and de Leoni, M.
(2018). Time and activity sequence prediction of busi-
ness process instances. Comput., 100(9):1005–1031.
Ribeiro, M. T., Singh, S., and Guestrin, C. (2016). “why
should i trust you?” explaining the predictions of any
classifier. In Proc. of the 22nd ACM SIGKDD Int.
Conf. Knowl. Discovery Data Min., pages 1135–1144.
Rizzi, W., Di Francescomarino, C., and Maggi, F. M.
(2020). Explainability in predictive process monitor-
ing: When understanding helps improving. In Proc.
of the Bus. Process Manage. Forum, pages 141–158.
Robeer, M. J. (2018). Contrastive explanation for machine
learning. Master’s thesis, Utrecht University.
Roscher, R., Bohn, B., Duarte, M. F., and Garcke, J. (2020).
Explainable machine learning for scientific insights
and discoveries. IEEE Access, 8:42200–42216.
Sagi, O. and Rokach, L. (2020). Explainable decision
forest: Transforming a decision forest into an inter-
pretable tree. Inf. Fusion, 61:124–138.
Teinemaa, I., Dumas, M., Rosa, M. L., and Maggi, F. M.
(2019). Outcome-oriented predictive process moni-
toring: Review and benchmark. ACM Trans. Knowl.
Discovery Data, 13(2):1–57.
Verenich, I., Dumas, M., La Rosa, M., and Nguyen, H.
(2019a). Predicting process performance: A white-
box approach based on process models. J. of Software:
Evol. Process, 31(6).
Verenich, I., Dumas, M., Rosa, M. L., Maggi, F. M., and
Teinemaa, I. (2019b). Survey and cross-benchmark
comparison of remaining time prediction methods in
business process monitoring. ACM Transactions on
Intelligent Systems and Technology, 10(4).
Warmuth, C. and Leopold, H. (2022). On the potential of
textual data for explainable predictive process moni-
toring. In Proc. of 3rd Int. Workshop on Leveraging
Mach. Learn. in Process Min., pages 1–12.
Weinzierl, S., Zilker, S., Brunk, J., Revoredo, K., Matzner,
M., and Becker, J. (2020). Xnap: Making lstm-based
next activity predictions explainable by using lrp. In
Proc. of Workshop on Artif. Intell. Bus. Process Man-
age., pages 129–141.
Wickramanayake, B., He, Z., Ouyang, C., Moreira, C., Xu,
Y., and Sindhgatta, R. (2022). Building interpretable
models for business process prediction using shared
and specialised attention mechanisms. Knowledge-
Based Syst., 248.
ICEIS 2023 - 25th International Conference on Enterprise Information Systems
406