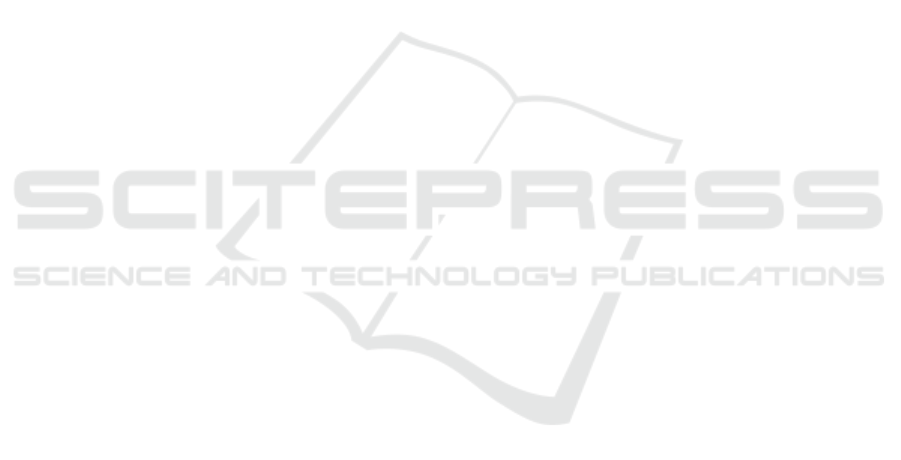
a global crisis. We found that communities using
hashtags in relation to anti-war and anti-Putin senti-
ments tend to exhibit a more negative tone than those
communities associated with expressions of support
for Ukraine. Additionally, the study revealed that
there was a concentration of communities around spe-
cific targets. International organizations and offices
such as @potus, @NATO, and @UN were frequently
mentioned by users and were typically addressed as
potential facilitators of a potential conflict resolution.
Moreover, our findings indicate that the reac-
tions within the top 5 communities were predomi-
nantly characterized by negative emotions, particu-
larly anger, and tend to spread more quickly and more
widely on Twitter than positive emotions. In our fu-
ture work, we plan to extend this study by applying
a temporal community detection algorithm to identify
the dynamics evolution of network communities and
also provide a more fine-grained analysis of related
user behavior.
REFERENCES
Back, M. D., K
¨
ufner, A. C., and Egloff, B. (2010). The emo-
tional timeline of september 11, 2001. Psychological
Science, 21(10):1417–1419.
Bedi, P. and Sharma, C. (2016). Community detection in
social networks. WIREs Data Mining and Knowledge
Discovery, 6(3):115–135.
Blondel, V. D., Guillaume, J.-L., Lambiotte, R., and Lefeb-
vre, E. (2008). Fast unfolding of communities in large
networks. Journal of statistical mechanics: theory
and experiment, 2008(10):P10008.
Cohn, M. A., Mehl, M. R., and Pennebaker, J. W. (2004).
Linguistic markers of psychological change surround-
ing september 11, 2001. Psychological science,
15(10):687–693.
Fani, H., Zarrinkalam, F., Bagheri, E., and Du, W. (2016).
Time-sensitive topic-based communities on twitter. In
Canadian conference on artificial intelligence, pages
192–204. Springer.
Flake, G. W., Lawrence, S., Giles, C. L., and Coetzee, F. M.
(2002). Self-organization and identification of web
communities. Computer, 35(3):66–70.
Girvan, M. and Newman, M. E. (2002). Community struc-
ture in social and biological networks. Proceedings of
the national academy of sciences, 99(12):7821–7826.
Gupta, A., Joshi, A., and Kumaraguru, P. (2012). Identi-
fying and characterizing user communities on twitter
during crisis events. In Proceedings of the 2012 work-
shop on Data-driven user behavioral modelling and
mining from social media, pages 23–26.
Javed, M. A., Younis, M. S., Latif, S., Qadir, J., and Baig,
A. (2018). Community detection in networks: A mul-
tidisciplinary review. Journal of Network and Com-
puter Applications, 108:87–111.
Ku
ˇ
sen, E. and Strembeck, M. (2019). An analysis of
emotion-exchange motifs in multiplex networks dur-
ing emergency events. Applied Network Science, 4.
Lee, J., Rehman, B. A., Agrawal, M., and Rao, H. R. (2015).
Sentiment analysis of twitter users over time: the case
of the boston bombing tragedy. In Workshop on E-
Business, pages 1–14. Springer.
Lingam, G., Rout, R. R., Somayajulu, D., and Das, S. K.
(2020a). Social botnet community detection: A novel
approach based on behavioral similarity in twitter net-
work using deep learning. In Proceedings of the 15th
ACM Asia Conference on Computer and Communica-
tions Security, ASIA CCS ’20, page 708–718, New
York, NY, USA. Association for Computing Machin-
ery.
Lingam, G., Rout, R. R., Somayajulu, D., and Das, S. K.
(2020b). Social botnet community detection: A novel
approach based on behavioral similarity in twitter net-
work using deep learning. In Proceedings of the 15th
ACM Asia Conference on Computer and Communica-
tions Security, ASIA CCS ’20, page 708–718, New
York, NY, USA. Association for Computing Machin-
ery.
McPherson, M., Smith-Lovin, L., and Cook, J. M. (2001).
Birds of a feather: Homophily in social networks. An-
nual review of sociology, pages 415–444.
Pennebaker, J. W., Booth, R. J., and Francis, M. E. (2007).
Linguistic inquiry and word count: Liwc [computer
software]. Austin, TX: liwc. net, 135.
Pennebaker, J. W., Mehl, M. R., and Niederhoffer, K. G.
(2003). Psychological aspects of natural language use:
Our words, our selves. Annual review of psychology,
54(1):547–577.
Raghavan, U. N., Albert, R., and Kumara, S. (2007).
Near linear time algorithm to detect community struc-
tures in large-scale networks. Physical review E,
76(3):036106.
R
´
ıos, S. A. and Mu
˜
noz, R. (2012). Dark web portal overlap-
ping community detection based on topic models. In
Proceedings of the ACM SIGKDD Workshop on Intel-
ligence and Security Informatics, ISI-KDD ’12, New
York, NY, USA. Association for Computing Machin-
ery.
Rosvall, M., Axelsson, D., and Bergstrom, C. (2009). The
map equation. Eur. Phys. J. Spec. Top., 178:13–23.
Rosvall, M. and Bergstrom, C. T. (2008). Maps of random
walks on complex networks reveal community struc-
ture. Proceedings of the national academy of sciences,
105(4):1118–1123.
S
´
anchez, D. L., Revuelta, J., Prieta, F. D. l., Gil-Gonz
´
alez,
A. B., and Dang, C. (2016). Twitter user clustering
based on their preferences and the louvain algorithm.
In International Conference on Practical Applications
of Agents and Multi-Agent Systems, pages 349–356.
Springer.
Schaeffer, S. E. (2007). Graph clustering. Computer Sci-
ence Review, 1(1):27–64.
Sch
¨
one, J. P., Parkinson, B., and Goldenberg, A. (2021).
Negativity spreads more than positivity on twitter af-
An Analysis of Twitter Communities Related to the 2022 War in Ukraine
35