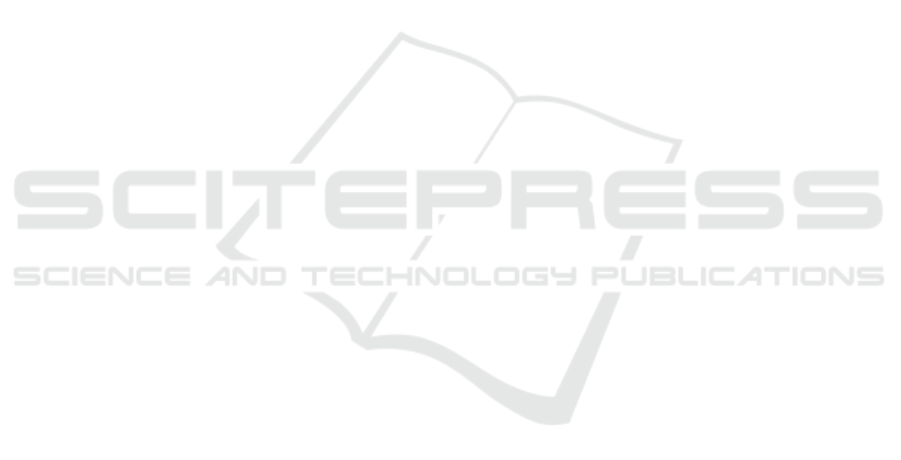
struction is further evidence of the variable-variable
nature of L2 vocabulary KCs. A practical concern
regarding the use feature focussing in CALL applica-
tions is that the distractors must be carefully selected
so as to be sufficiently similar to the target. This re-
quirement is reasonable when working with pseudo-
words, but is much harder to satisfy when working
with natural L2 vocabulary.
Rather than employ feature focussing in CALL
applications directly, we advocate for the use of auto-
mated methods that capitalize on the variable-variable
nature of L2 vocabulary KCs. We explore such meth-
ods in the following.
3.2 Implications for CALL
Rejecting the constant-constant KC conceptualiza-
tion of L2 vocabulary learning does not amount to
rejecting the use of spaced repetition algorithms in
CALL applications. As argued by (Koedinger et al.,
2012), the memory and fluency processes addressed
by spaced repetition are equally vital to the acquisi-
tion of variable-variable KCs, which could otherwise
be forgotten. Rather, we argue that spaced repetition
should be used in combination with learner models
that are sensitive to the fuzzy, implicit skills involved
in L2 vocabulary learning, i.e. the recognition and
production of particular sound and spelling patterns.
A contemporary approach would be to apply deep
learning skill-based learner models to L2 vocabulary
learning. Such models have generally been designed
with discrete skills in mind, with each exercise falling
under one or more skill categories (Piech et al., 2015;
Pu et al., 2020). These models can, however, be mod-
ified to work with vector representations of L2 vocab-
ulary items that are sensitive to L1 and L2 wordlike-
ness, such as the bilingual orthographic embeddings
proposed by (Severini et al., 2020).
Such a model could detect and adapt to the unique
learning burden experienced by learners with differ-
ent backgrounds; for example, an English-speaking
learner of Polish might struggle with particular con-
sonant clusters that are illegal under English spelling,
whereas a Czech-speaking learner of Polish might
be familiar with those letter combinations, but strug-
gle with an entirely different set of spelling patterns.
CALL applications equipped with a learner model
that has access to the spelling and sound patterns of
the words being learned could adapt to this unique
behaviour, and recommend personalized vocabulary
lists tuned to the sound and spelling patterns that each
learner is familiar with, thus lightening the learning
burden.
4 CONCLUSIONS
The dependency chain from KC type, to learning
process, to instructional method defined by the KLI
framework makes explicit the fact that how we con-
ceptualize the KCs involved in a particular subject
matter has consequences for the instructional methods
we choose to employ. The “vocabulary fact” concep-
tualization of L2 vocabulary learning functions both
to justify the use of spaced repetition algorithms, as
well as to argue against the use of more complex,
skill-based student models in CALL applications.
On the basis of extensive evidence of interaction
and transfer effects in L2 vocabulary learning, and
evidence of the efficacy of L2 vocabulary instruction
methods tailored to variable-variable KC acquisition,
we argue that L2 vocabulary learners develop gener-
alisable skills, and advocate for the use of skill-based
learner models in CALL applications. While steps in
this direction have already been taken, e.g. (Zylich
and Lan, 2021), such approaches are still in the mi-
nority, and we hope that the theoretical justification
presented here will encourage others to contribute to
this effort.
REFERENCES
Bartolotti, J. and Marian, V. (2017a). Bilinguals’ existing
languages benefit vocabulary learning in a third lan-
guage. Language Learning, 67(1):110–140.
Bartolotti, J. and Marian, V. (2017b). Orthographic knowl-
edge and lexical form influence vocabulary learning.
Applied Psycholinguistics, 38(2):427–456.
Baxter, P., Bekkering, H., Dijkstra, T., Droop, M., van den
Hurk, M., and Leon
´
e, F. (2022). Contrasting ortho-
graphically similar words facilitates adult second lan-
guage vocabulary learning. Learning and Instruction,
80:101582.
Baxter, P., Droop, M., van den Hurk, M., Bekkering, H.,
Dijkstra, T., and Leon
´
e, F. (2021). Contrasting similar
words facilitates second language vocabulary learn-
ing in children by sharpening lexical representations.
Frontiers in Psychology, 12:688160.
Belardi, A., Pedrett, S., Rothen, N., and Reber, T. P.
(2021). Spacing, feedback, and testing boost vocabu-
lary learning in a web application. Frontiers in Psy-
chology, 12.
Cepeda, N. J., Pashler, H., Vul, E., Wixted, J. T., and Rohrer,
D. (2006). Distributed practice in verbal recall tasks:
A review and quantitative synthesis. Psychological
Bulletin, 132(3):354–380.
Choffin, B., Popineau, F., Bourda, Y., and Vie, J.-J. (2019).
DAS3H: Modeling student learning and forgetting
for optimally scheduling distributed practice of skills.
arXiv:1905.06873.
CSEDU 2023 - 15th International Conference on Computer Supported Education
328