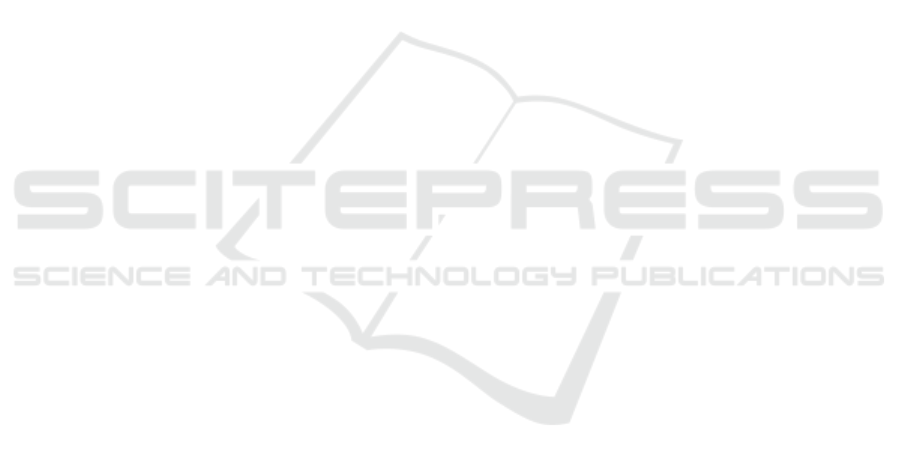
REFERENCES
An, P., Xu, S., Harmon, S. A., Turkbey, E. B., Sanford,
T. H., Amalou, A., Kassin, M., Varble, N., Blain,
M., Anderson, V., Patella, F., Carrafiello, G., Turkbey,
B. T., and Wood, B. J. (2020). CT Images in COVID-
19.
Beltran, R. A. (2005). The Gold Standard: The Challenge
of Evidence-Based Medicine and Standardization in
Health Care. Journal of the National Medical Associ-
ation, 97(1):110.
Bridge, P., Fielding, A., Rowntree, P., and Pullar, A.
(2016). Intraobserver Variability: Should We Worry?
Journal of Medical Imaging and Radiation Sciences,
47(3):217–220.
Campolo, A. and Crawford, K. (2020). Enchanted Deter-
minism: Power without Responsibility in Artificial In-
telligence. Engaging Science, Technology, and Soci-
ety, 6:1–19.
Donald, J. J. and Barnard, S. A. (2012). Common patterns
in 558 diagnostic radiology errors. Journal of Medical
Imaging and Radiation Oncology, 56(2):173–178.
Enni, S. A. and Herrie, M. B. (2021). Turning biases into
hypotheses through method: A logic of scientific dis-
covery for machine learning. Big Data and Society,
8(1).
Hey, T., Tansley, S., and Tolle, K. M. (2021). Fourth
Paradigm.
Kim, J. H., Han, S. G., Cho, A., Shin, H. J., and Baek,
S.-E. (2021). Effect of deep learning-based assistive
technology use on chest radiograph interpretation by
emergency department physicians: a prospective in-
terventional simulation-based study. BMC Medical
Informatics and Decision Making, 21(1):1–9.
Kitchin, R. (2014). Big Data, new epistemologies and
paradigm shifts. Big Data and Society, 1(1):1–12.
Kuhn, T. S. (1962). The structure of Scientific Revolution,
volume I,II.
Kuznetsova, A., Rom, H., Alldrin, N., Uijlings, J., Krasin,
I., Pont-Tuset, J., Kamali, S., Popov, S., Malloci, M.,
Kolesnikov, A., Duerig, T., and Ferrari, V. (2020). The
Open Images Dataset V4: Unified Image Classifica-
tion, Object Detection, and Visual Relationship De-
tection at Scale. International Journal of Computer
Vision, 128(7):1956–1981.
Leonelli, S. (2014). What difference does quantity make?
On the epistemology of Big Data in biology. Big Data
and Society, 1(1):1–11.
Litjens, G., Kooi, T., Bejnordi, B. E., Setio, A. A. A.,
Ciompi, F., Ghafoorian, M., van der Laak, J. A., van
Ginneken, B., and S
´
anchez, C. I. (2017). A survey
on deep learning in medical image analysis. Medical
Image Analysis, 42(December 2012):60–88.
Lizzi, F., Brero, F., Cabini, R. F., Fantacci, M. E., Piffer, S.,
Postuma, I., Rinaldi, L., and Retico, A. (2021). Mak-
ing data big for a deep-learning analysis: Aggrega-
tion of public COVID-19 datasets of lung computed
tomography scans. Proceedings of the 10th Interna-
tional Conference on Data Science, Technology and
Applications, DATA 2021, (Data):316–321.
Neri, E., Coppola, F., Miele, V., Bibbolino, C., and Grassi,
R. (2020). Artificial intelligence: Who is responsible
for the diagnosis? Radiologia Medica, 125(6):517–
521.
Pesapane, F., Codari, M., and Sardanelli, F. (2018). Artifi-
cial intelligence in medical imaging: threat or oppor-
tunity? Radiologists again at the forefront of innova-
tion in medicine. European Radiology Experimental,
2(1).
Renard, F., Guedria, S., Palma, N. D., and Vuillerme, N.
(2020). Variability and reproducibility in deep learn-
ing for medical image segmentation. Scientific Re-
ports, 10(1):1–16.
Standard DICOM (2021). DICOM standard.
Stevens, M., Wehrens, R., and de Bont, A. (2018). Con-
ceptualizations of Big Data and their epistemological
claims in healthcare: A discourse analysis. Big Data
and Society, 5(2):1–21.
WHO (2021). Ethics and Governance of Artificial Intelli-
gence for Health Ethics and Governance of Artificial
Intelligence for Health 2.
Deep Learning and Medical Image Analysis: Epistemology and Ethical Issues
179