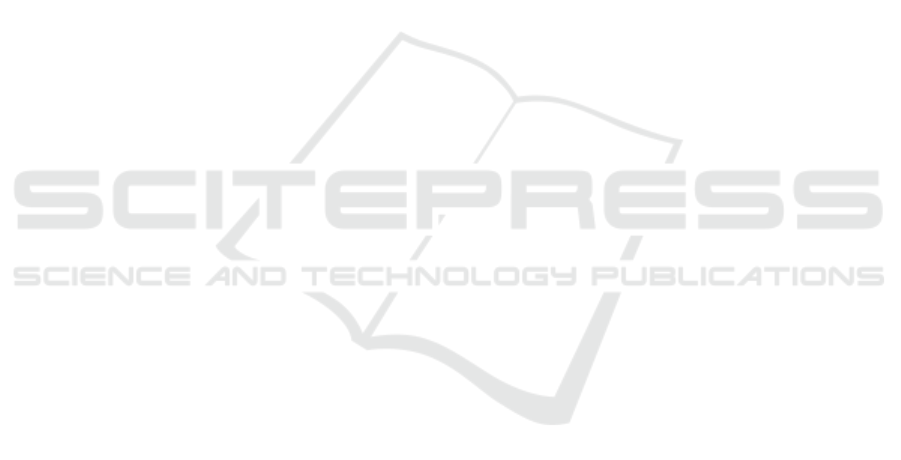
Garouani, M., Ahmad, A., Bouneffa, M., and Hamlich, M.
(2022a). AMLBID: An auto-explained automated ma-
chine learning tool for big industrial data. SoftwareX,
17:100919.
Garouani, M., Ahmad, A., Bouneffa, M., and Hamlich, M.
(2022b). Scalable meta-bayesian based hyperparame-
ters optimization for machine learning. In Communi-
cations in Computer and Information Science, pages
173–186. Springer International Publishing.
Garouani, M., Ahmad, A., Bouneffa, M., and Hamlich,
M. (2023a). Autoencoder-knn meta-model based data
characterization approach for an automated selection
of ai algorithms. Journal of Big Data, 10(14).
Garouani, M., Ahmad, A., Bouneffa, M., and Hamlich,
M. (2023b). Autoencoder-knn meta-model based data
characterization approach for an automated selection
of ai algorithms. Journal of Big Data.
Garouani, M., Ahmad, A., Bouneffa, M., Hamlich, M.,
Bourguin, G., and Lewandowski, A. (2022c). Towards
big industrial data mining through explainable auto-
mated machine learning. The International Journal
of Advanced Manufacturing Technology, 120:1169–
1188.
Garouani, M., Ahmad, A., Bouneffa, M., Hamlich, M.,
Bourguin, G., and Lewandowski, A. (2022d). Using
meta-learning for automated algorithms selection and
configuration: an experimental framework for indus-
trial big data. Journal of Big Data, 9:1169–1188.
Garouani., M., Ahmad., A., Bouneffa., M., Lewandowski.,
A., Bourguin., G., and Hamlich., M. (2021). Towards
the automation of industrial data science: A meta-
learning based approach. In Proceedings of the 23rd
International Conference on Enterprise Information
Systems - Volume 1: ICEIS, pages 709–716.
Garouani, M. and Zaysa, K. (2022). Leveraging the auto-
mated machine learning for arabic opinion mining: A
preliminary study on AutoML tools and comparison
to human performance. In Digital Technologies and
Applications, pages 163–171. Springer International
Publishing.
Hart, S. (1989). Shapley value. In Game Theory, pages
210–216. Palgrave Macmillan UK.
Kalousis, A. and Hilario, M. (2001). Feature Selection
for Meta-learning. In Cheung, D., Williams, G. J.,
and Li, Q., editors, Advances in Knowledge Discov-
ery and Data Mining, Lecture Notes in Computer Sci-
ence, pages 222–233. Springer.
Lundberg, S. and Lee, S.-I. (2017a). A unified approach to
interpreting model predictions.
Lundberg, S. M. and Lee, S.-I. (2017b). A unified approach
to interpreting model predictions. In Proceedings of
the 31st International Conference on Neural Informa-
tion Processing Systems, NIPS’17, page 4768–4777,
Red Hook, NY, USA. Curran Associates Inc.
Nural, M. V., Peng, H., and Miller, J. A. (2017). Using
meta-learning for model type selection in predictive
big data analytics. In 2017 IEEE International Con-
ference on Big Data (Big Data), pages 2027–2036.
Olsen, L. H. B., Glad, I. K., Jullum, M., and Aas, K.
(2022). Using shapley values and variational autoen-
coders to explain predictive models with dependent
mixed features. Journal of Machine Learning Re-
search, 23(213):1–51.
Samek, W., Montavon, G., Vedaldi, A., Hansen, L. K., and
M
¨
uller, K.-R., editors (2019). Explainable AI: Inter-
preting, Explaining and Visualizing Deep Learning.
Springer International Publishing.
Shao, X., Wang, H., Zhu, X., and Xiong, F. (2022).
Find:explainable framework for meta-learning.
Shao, X., Wang, H., Zhu, X., Xiong, F., Mu, T., and Zhang,
Y. (2023). EFFECT: Explainable framework for meta-
learning in automatic classification algorithm selec-
tion. Information Sciences, 622:211–234.
Wo
´
znica, K. and Biecek, P. (2020). Towards explainable
meta-learning.
ICEIS 2023 - 25th International Conference on Enterprise Information Systems
598