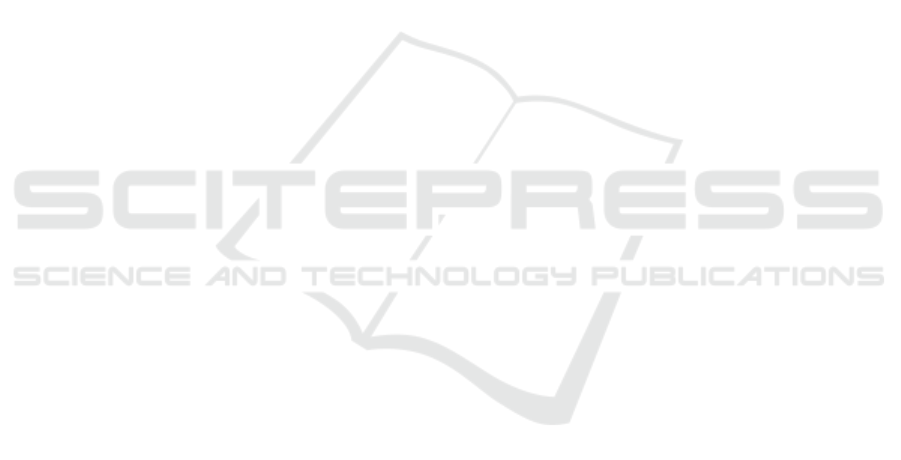
Finlayson, I. R., Lickley, R. J., and Corley, M. (2010). The
influence of articulation rate, and the disfluency of
others, on one’s own speech. In Watanabe, M. and
Rose, R., editors, DiSS-LPSS Joint Workshop 2010 -
5th Workshop on Disfluency in Spontaneous Speech
and 2nd International Symposium on Linguistic Pat-
terns in Spontaneous Speech, pages 119–122, Tokyo,
Japan. University of Tokyo; September 25-26, 2010.
Floridi, L. and Cowls, J. (2019). A Unified Framework of
Five Principles for AI in Society. Harvard Data Sci-
ence Review, 1(1).
Fraundorf, S. H. and Watson, D. G. (2011). The disfluent
discourse: Effects of filled pauses on recall. Journal
of Memory and Language, 65(2):161–175.
Giles, H. and Smith, P. M. (1979). Accommodation theory.
In Giles, H. and St. Clair, R. N., editors, Language
and social psychology, pages 45–65. Basil Blackwell,
Oxford, UK.
Goldman-Eisler, F. (1961). A comparative study of two hes-
itation phenomena. Language and Speech, 4(1):18–
26.
Goldman-Eisler, F. (1972). Pauses, clauses, sentences. Lan-
guage and Speech, 15(2):103–113.
Griffiths, R. (1991). The paradox of comprehensible input:
Hesitation phenomena in l2 teacher-talk. JALT Jour-
nal, 13(1):23–41.
G
¨
otz, S. (2013). Fluency in Native and Nonnative En-
glish Speech, volume 53 of Studies in Corpus Linguis-
tics. John Benjamins Publishing Company, Amster-
dam, Netherlands.
Henderson, P., Sinha, K., Angelard-Gontier, N., Ke, N. R.,
Fried, G., Lowe, R., and Pineau, J. (2018). Ethical
challenges in data-driven dialogue systems. In Pro-
ceedings of the 2018 AAAI/ACM Conference on AI,
Ethics, and Society, AIES ’18, page 123–129, New
York, NY, USA. Association for Computing Machin-
ery.
Jansen, F. and Janssen, D. (2013). Uw reservering is eh
komen te vervallen - experimenteel onderzoek naar
het effect van gevulde pauzes in voicemails met slecht
nieuws. Tijdschrift voor Taalbeheersing, 35(3):237–
253.
Jobin, A., Ienca, M., and Vayena, E. (2019). The global
landscape of ai ethics guidelines. Nature Machine In-
telligence, 1(9):389–399.
Kasl, S. V. and Mahl, G. F. (1965). Relationship of distur-
bances and hesitations in spontaneous speech to anx-
iety. Journal of Personality and Social Psychology,
1.
Levinson, S. C. (1983). Pragmatics. Cambridge University
Press, Cambridge, UK.
Lickley, R. J. (1995). Missing disfluencies. In Proceed-
ings of International Congress of Phonetic Science,
volume 4, pages 192–195, Stockholm.
Maclay, H. and Osgood, C. (1959). Hesitation phenomena
in spontaneous english speech. Word, 15:19–44.
Matsunaga, Y., Saeki, T., Takamichi, S., and Saruwatari,
H. (2022). Personalized filled-pause generation with
group-wise prediction models. In Proceedings of the
Thirteenth Language Resources and Evaluation Con-
ference, pages 385–392, Marseille, France. European
Language Resources Association.
O’Connell, D. and Kowal, S. (2004). The history of re-
search on the filled pause as evidence of ’the written
language bias in linguistics’ (linell, 1982). Journal of
Psycholinguistic Research, 33(6):459–474.
Ohshima, N., Kimijima, K., Yamato, J., and Mukawa, N.
(2015). A conversational robot with vocal and bodily
fillers for recovering from awkward silence at turn-
takings. In 2015 24th IEEE International Sympo-
sium on Robot and Human Interactive Communica-
tion (RO-MAN), pages 325–330.
Pfeifer, L. M. and Bickmore, T. (2009). Should agents
speak like, um, humans? the use of conversational
fillers by virtual agents. In Ruttkay, Z., Kipp, M.,
Nijholt, A., and Vilhj
´
almsson, H., editors, Intelligent
Virtual Agents. IVA 2009. Lecture Notes in Computer
Science, volume 5773, pages 460–466, Berlin, Hei-
delberg. Springer.
Qader, R., Lecorv
´
e, G., Lolive, D., and S
´
ebillot, P. (2018).
Disfluency insertion for spontaneous tts: Formaliza-
tion and proof of concept. In Dutoit, T., Mart
´
ın-Vide,
C., and Pironkov, G., editors, Statistical Language
and Speech Processing, pages 32–44, Cham. Springer
International Publishing.
Rose, R. L. (2019a). The structural signaling effect of silent
and filled pauses. In Rose, R. and Eklund, R., edi-
tors, The 9th Workshop on Disfluency in Spontaneous
Speech (DiSS 2019), pages 19–22, Budapest, Hun-
gary. ELTE E
¨
otv
¨
os Lor
´
and University; September 12-
13, 2019.
Rose, R. L. (2019b). The structural signalling effect of
filled pauses during reading. Poster presentation
at CUNY Human Sentence Processing Conference,
Boulder. CO, USA.
Shriberg, E. (1994). Preliminaries to a theory of speech
disfluencies. phd, University of California, Berkeley.
Smiley, C., Schilder, F., Plachouras, V., and Leidner, J. L.
(2017). Say the right thing right: Ethics issues in natu-
ral language generation systems. In Proceedings of the
First ACL Workshop on Ethics in Natural Language
Processing, pages 103–108, Valencia, Spain. Associ-
ation for Computational Linguistics.
Wang, S., Gustafson, J., and Sz
´
ekely,
´
E. (2022). Evaluating
sampling-based filler insertion with spontaneous TTS.
In Proceedings of the Thirteenth Language Resources
and Evaluation Conference, pages 1960–1969, Mar-
seille, France. European Language Resources Associ-
ation.
Yang, J., Yang, D., and Ma, Z. (2020). Planning and gener-
ating natural and diverse disfluent texts as augmenta-
tion for disfluency detection. In Proceedings of the
2020 Conference on Empirical Methods in Natural
Language Processing (EMNLP), pages 1450–1460,
Online. Association for Computational Linguistics.
Ethical Considerations in the Use of Disfluencies in AI-Generated Speech
135