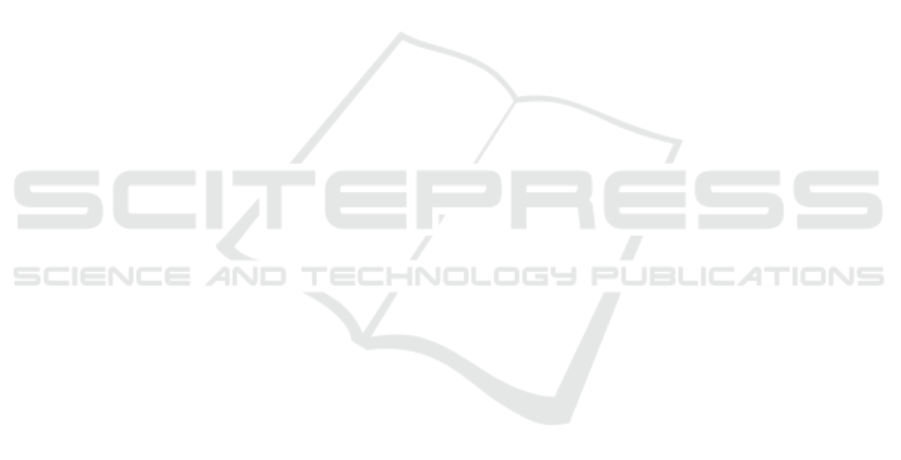
GC is supported by PON Initiative 2014-2020 action
IV.6 funded by the Italian Ministry of University and
Research at the University of Milan-Bicocca (Milan,
Italy).
REFERENCES
Altuwaijri, G. A. and Muhammad, G. (2022). A multi-
branch of convolutional neural network models for
electroencephalogram-based motor imagery classifi-
cation. Biosensors, 12(1).
Beraldo, G., Tonin, L., Mill
´
an, J. d. R., and Menegatti, E.
(2022). Shared intelligence for robot teleoperation via
BMI. IEEE Transactions on Human-Machine Sys-
tems.
Blankertz, B., Dornhege, G., Krauledat, M., M
¨
uller, K.-R.,
and Curio, G. (2007). The non-invasive Berlin Brain-
Computer Interface: Fast acquisition of effective per-
formance in untrained subjects. NeuroImage, 37:539–
50.
Blei, D. M., Kucukelbir, A., and McAuliffe, J. D.
(2017). Variational inference: A review for statisti-
cians. Journal of the American statistical Association,
112(518):859–877.
Bressan, G., Cisotto, G., M
¨
uller-Putz, G. R., and Wriess-
negger, S. C. (2021). Deep learning-based classifica-
tion of fine hand movements from low frequency eeg.
Future Internet, 13(5):103.
Cisotto, G., Capuzzo, M., Guglielmi, A. V., and Zanella,
A. (2022). Feature stability and setup minimiza-
tion for EEG-EMG-enabled monitoring systems.
EURASIP Journal on Advances in Signal Processing,
2022(1):103.
Deng, X., Zhang, B., Yu, N., Liu, K., and Sun, K.
(2021). Advanced TSGL-EEGNet for motor imagery
EEG-based Brain-Computer Interfaces. IEEE Access,
9:25118–25130.
Hinton, G. E. and Salakhutdinov, R. R. (2006). Reducing
the dimensionality of data with neural networks. sci-
ence, 313(5786):504–507.
Kai Keng Ang, Zheng Yang Chin, Haihong Zhang, and
Cuntai Guan (2008). Filter bank common spatial
pattern (FBCSP) in Brain-Computer Interface. In
2008 IEEE Int. Joint Conf. on Neural Networks (IEEE
World Congress on Computational Intelligence).
Kingma, D. P. and Welling, M. (2013). Auto-encoding vari-
ational bayes. arXiv preprint arXiv:1312.6114.
Lawhern, V., Solon, A., Waytowich, N., Gordon, S., Hung,
C., and Lance, B. (2016). EEGNet: A compact convo-
lutional network for EEG-based Brain-Computer In-
terfaces. Journal of Neural Engineering, 15.
Lazurenko, D., Kiroy, V., Aslanyan, E., Shepelev, I.,
Bakhtin, O., and Minyaeva, N. (2018). Electrographic
properties of movement-related potentials. Neu-
roscience and Behavioral Physiology, 48(9):1078–
1087.
Li, D., Wang, J., Xu, J., and Fang, X. (2019a). Densely
feature fusion based on convolutional neural networks
for motor imagery EEG classification. IEEE Access,
7:132720–132730.
Li, H., Huang, G., Lin, Q., Zhao, J.-L., Lo, W.-L. A., Mao,
Y.-R., Chen, L., Zhang, Z.-G., Huang, D.-F., and Li,
L. (2018). Combining movement-related cortical po-
tentials and event-related desynchronization to study
movement preparation and execution. Frontiers in
neurology, 9:822.
Li, Y., Pan, Q., Wang, S., Peng, H., Yang, T., and Cambria,
E. (2019b). Disentangled variational auto-encoder
for semi-supervised learning. Information Sciences,
482:73–85.
Loshchilov, I. and Hutter, F. (2019). Decoupled weight
decay regularization. In International Conference on
Learning Representations.
Magnuson, J. R. and McNeil, C. J. (2021). Low-frequency
neural activity at rest is correlated with the movement-
related cortical potentials elicited during both real
and imagined movements. Neuroscience Letters,
742:135530.
Manjunatha, H. and Esfahani, E. T. (2021). Extracting in-
terpretable eeg features from a deep learning model to
assess the quality of human-robot co-manipulation. In
2021 10th International IEEE/EMBS Conference on
Neural Engineering (NER), pages 339–342. IEEE.
Olivas, B. E. and Chacon, M. (2018). Classification of mul-
tiple motor imagery using deep convolutional neural
networks and spatial filters. Applied Soft Computing,
75.
Pfurtscheller, G., Brunner, C., Schl
¨
ogl, A., and Da Silva,
F. L. (2006). Mu rhythm (de) synchronization and
EEG single-trial classification of different motor im-
agery tasks. NeuroImage, 31(1):153–159.
Riyad, M., Khalil, M., and Adib, A. (2021). MI-EEGNET:
A novel convolutional neural network for motor im-
agery classification. Journal of Neuroscience Meth-
ods, 353:109037.
Sakhavi, S., Guan, C., and Yan, S. (2018). Learning
temporal information for brain-computer interface us-
ing convolutional neural networks. IEEE Trans-
actions on Neural Networks and Learning Systems,
29(11):5619–5629.
Scapin, D., Cisotto, G., Gindullina, E., and Badia, L.
(2022). Shapley value as an aid to biomedical ma-
chine learning: a heart disease dataset analysis. In
2022 22nd IEEE International Symposium on Cluster,
Cloud and Internet Computing (CCGrid), pages 933–
939. IEEE.
Schirrmeister, R. T., Springenberg, J. T., Fiederer, L. D. J.,
Glasstetter, M., Eggensperger, K., Tangermann, M.,
Hutter, F., Burgard, W., and Ball, T. (2017). Deep
learning with convolutional neural networks for EEG
decoding and visualization. Human Brain Mapping.
Schneider, T., Wang, X., Hersche, M., Cavigelli, L., and
Benini, L. (2020). Q-EEGNet: An energy-efficient
8-bit quantized parallel EEGNet implementation for
edge motor-imagery brain-machine interfaces. In
2020 IEEE International Conference on Smart Com-
puting (SMARTCOMP), pages 284–289. IEEE.
vEEGNet: A New Deep Learning Model to Classify and Generate EEG
251