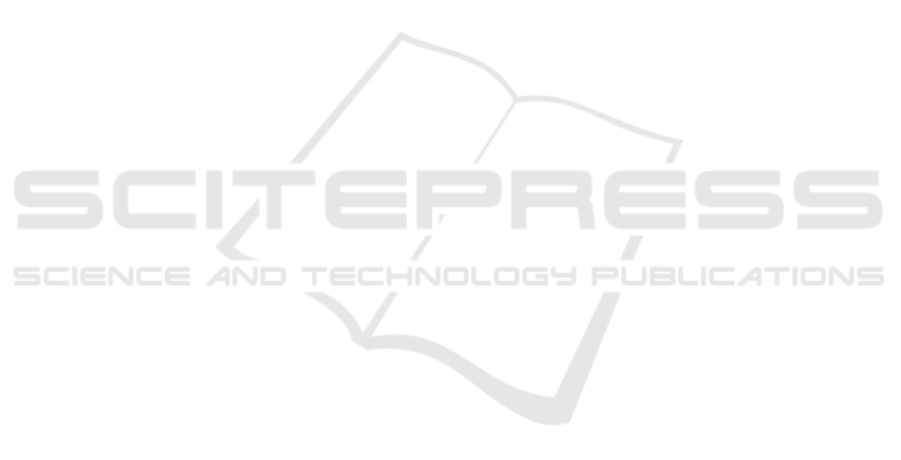
Learning phrase representations using rnn encoder-
decoder for statistical machine translation. arXiv
preprint arXiv:1406.1078.
Cimino, M. G., De Francesco, N., Mercaldo, F., San-
tone, A., and Vaglini, G. (2020). Model checking
for malicious family detection and phylogenetic anal-
ysis in mobile environment. Computers & Security,
90:101691.
Cimino., M. G. C. A., Galatolo., F. A., Parola., M., Per-
illi., N., and Squeglia., N. (2022). Deep learning
of structural changes in historical buildings: The
case study of the pisa tower. In Proceedings of
the 14th International Joint Conference on Compu-
tational Intelligence - NCTA,, pages 396–403. IN-
STICC, SciTePress.
computerworld.com (2016). New point-of-sale mal-
ware multigrain steals card data over dns.
https://www.computerworld.com/article/3059317/new-
point-of-sale-malware-multigrain-steals-card-data-
over-dns.html.
connectpos.com (2020). Types of pos (point of sale) sys-
tem for retailers. https://www.connectpos.com/types-
of-pos-system-connectpos/.
creditcards.com (2021). Payment method statistics.
https://www.creditcards.com/statistics/payment-
method-statistics-1276/.
CTU University (2016). The stratosphere ips project
dataset. https://www.stratosphereips.org/datasets-
malware.
d3security.com (2017). Risks affecting point of sale ter-
minals. https://d3security.com/blog/risks-affecting-
point-of-sale-terminals/.
Dainotti, A., Pescape, A., and Claffy, K. C. (2012). Issues
and future directions in traffic classification. IEEE net-
work, 26(1):35–40.
Devlin, J., Chang, M.-W., Lee, K., and Toutanova, K.
(2018). Bert: Pre-training of deep bidirectional trans-
formers for language understanding.
financemagnates.com (2016). The future of fintech: the
death of cash and bank branches but a boost to retail.
https://www.financemagnates.com/fintech/bloggers/
fintech-the-death-of-cash-and-bank-branches-but-a
-boost-to-retail/.
FirstData (2010). Payments 101: Credit and debit card
payments- key concepts and industry issues. http:
//euro.ecom.cmu.edu/resources/elibrary/epay/Pa
yments-101.pdf.
fitsmallbusiness.com (2022). Types of pos
systems: A guide for small businesses.
https://fitsmallbusiness.com/types-of-pos-systems/.
Galatolo., F. A., Cimino., M. G. C. A., and Vaglini., G.
(2022). Zero-shot mathematical problem solving via
generative pre-trained transformers. In Proceedings of
the 24th International Conference on Enterprise In-
formation Systems - Volume 1: ICEIS,, pages 479–
483. INSTICC, SciTePress.
Gomzin, S. (2014). Hacking Point of Sale: Payment Appli-
cation Secrets, Threats, and Solutions. John Wiley &
Sons.
intel.com (2022). Deliver seamless expe-
riences with multiple types of pos.
https://www.intel.com/content/www/us/en/internet-
of-things/iot-solutions/pos/types-of-pos.html.
ISO/IEC 7813:2006 (2006). Iso/iec 7813:2006-
information technology — identifica-
tion cards — financial transaction cards.
https://www.iso.org/standard/43317.html.
ISO/IEC 7813:2006 (2013). Iso/iec 18092:2013 infor-
mation technology — telecommunications and in-
formation exchange between systems — near field
communication — interface and protocol (nfcip-1).
https://www.iso.org/standard/56692.html.
Lichy, A., Bader, O., Dubin, R., Dvir, A., and Hajaj, C.
(2023). When a rf beats a cnn and gru, together—a
comparison of deep learning and classical machine
learning approaches for encrypted malware traffic
classification. Computers & Security, 124:103000.
Liu, C., He, L., Xiong, G., Cao, Z., and Li, Z. (2019). Fs-
net: A flow sequence network for encrypted traffic
classification. In IEEE INFOCOM 2019-IEEE Con-
ference On Computer Communications, pages 1171–
1179. IEEE.
Lopez-Martin, M., Carro, B., Sanchez-Esguevillas, A., and
Lloret, J. (2017). Network traffic classifier with con-
volutional and recurrent neural networks for internet
of things. IEEE access, 5:18042–18050.
Luong, M.-T., Pham, H., and Manning, C. D. (2015). Ef-
fective approaches to attention-based neural machine
translation. arXiv preprint arXiv:1508.04025.
Mar
´
ın, G., Caasas, P., and Capdehourat, G. (2021).
Deepmal-deep learning models for malware traffic de-
tection and classification. In Data Science–Analytics
and Applications, pages 105–112. Springer.
Martino, G. (2023). Ramscrapersattentiondetectors github.
https://github.com/GabMartino/RAMScrapersAttenti
onDetectors.
Mirsky, Y., Doitshman, T., Elovici, Y., and Shabtai, A.
(2018). Kitsune: an ensemble of autoencoders for
online network intrusion detection. arXiv preprint
arXiv:1802.09089.
Rodr
´
ıguez, R. J. (2017). Evolution and characterization of
point-of-sale ram scraping malware. Journal of Com-
puter Virology and Hacking Techniques, 13(3):179–
192.
Said Elsayed, M., Le-Khac, N.-A., Dev, S., and Jurcut,
A. D. (2020). Network anomaly detection using lstm
based autoencoder. In Proceedings of the 16th ACM
Symposium on QoS and Security for Wireless and Mo-
bile Networks, Q2SWinet ’20, page 37–45, New York,
NY, USA. Association for Computing Machinery.
Secure Technology Alliance (2014). Emv: Faq.
https://www.securetechalliance.org/publications-
emv-faq/#q1.
securebox.comodo.com (2016). New multi-
grain malware eats memory, steals pos
data. https://securebox.comodo.com/blog/pos-
security/new-multigrain-malware-eats-memory-
steals-pos-data/.
Using Deep Learning with Attention to Detect Data Exfiltration by POS Malware
647