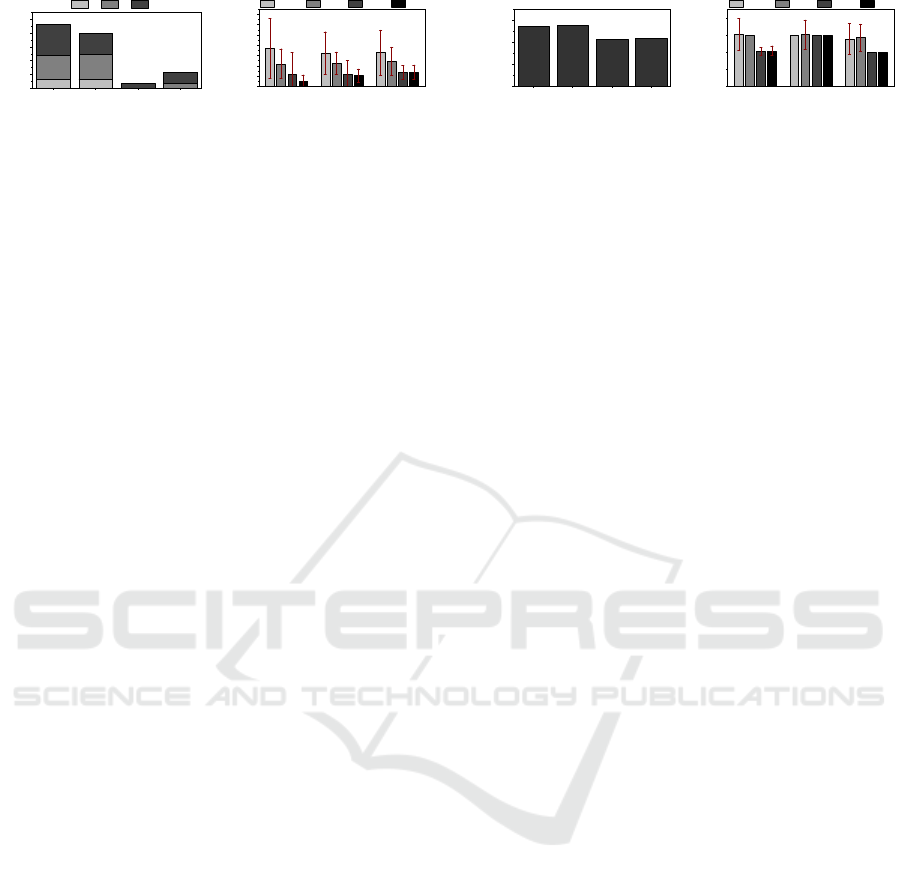
W o r s t F i t B e s t F i t N S G A - I I C l o v e r
0
1 0
2 0
3 0
4 0
5 0
S L A V i o l a t i o n s
A l g o r i t h m
1 2 3
(a) SLA Violations per
Chain Size.
0
1 0 0
2 0 0
3 0 0
4 0 0
5 0 0
6 0 0
7 0 0
L a t e n c y
W o r s t F i t B e s t F i t N S G A - I I C l o v e r
L a t e n c y S L A
(b) Latency per SLA.
9 6 0 0 0
9 6 7 0 0
7 5 4 0 0
7 6 2 0 0
W o r s t F i t B e s t F i t N S G A - I I C l o v e r
0
4 × 1 0
4
7 × 1 0
4
1 × 1 0
5
A l l o c a t i o n C o s t
A l g o r i t h m
(c) Overall Allocation Cost.
0
5 0
1 0 0
1 5 0
2 0 0
A l l o c a t i o n C o s t
W o r s t F i t B e s t F i t N S G A - I I C l o v e r
S e r v i c e T y p e
(d) Allocation Cost per Ser-
vice Type.
Figure 6: Experimental results comparing the evaluated strategies.
composite applications in geo-distributed cloud data
centers. Simulated experiments using real latency
traces from Amazon Web Services demonstrate that
Clover can achieve near-optimal results, reducing la-
tency issues and placement costs by 74.47% and
21.2%, respectively, compared to baseline strategies.
As future work, we indent to extend our approach,
considering other objectives such as high availabil-
ity, protecting applications against unfortunate events
such as cyberattacks and power outages, and mitigat-
ing vendor lock-in.
ACKNOWLEDGEMENT
This work was financed in part by the Coordenac¸
˜
ao
de Aperfeic¸oamento de Pessoal de N
´
ıvel Superior
- Brasil (CAPES) – Finance Code 001. Also,
this work was partially supported by Conselho
Nacional de Desenvolvimento Cient
´
ıfico e Tec-
nol
´
ogico – CNPq – 313111/2019-7, 404027/2021-
0. This work also received funding from S
˜
ao
Paulo Research Foundation (FAPESP) – 2021/06981-
0, 2020/05183-0, 2020/05115-4; and Rio Grande do
Sul Research Foundation (FAPERGS) – 19/2551-
0001266-7, 19/2551-0001224-1, 19/2551-0001689-
1, 21/2551-0000688-9.
REFERENCES
Anglano, C., Canonico, M., Castagno, P., Guazzone, M.,
and Sereno, M. (2020). Profit-aware coalition forma-
tion in fog computing providers: A game-theoretic ap-
proach. Concurrency and Computation: Practice and
Experience, 32(21):e5220. e5220 cpe.5220.
Aral, A., Demaio, V., and Brandic, I. (2021). Ares: Re-
liable and sustainable edge provisioning for wireless
sensor networks. IEEE Transactions on Sustainable
Computing, pages 1–12.
Blank, J. and Deb, K. (2020). Pymoo: Multi-objective op-
timization in python. IEEE Access, 8:89497–89509.
Blinowski, G., Ojdowska, A., and Przybyłek, A. (2022).
Monolithic vs. microservice architecture: A perfor-
mance and scalability evaluation. IEEE Access,
10:20357–20374.
Chaudhari, N., Bhadoria, R. S., and Prasad, S. (2016). In-
formation handling and processing using enterprise
service bus in service-oriented architecture system. In
2016 8th International Conference on Computational
Intelligence and Communication Networks (CICN),
pages 418–421.
De Donno, M., Tange, K., and Dragoni, N. (2019). Founda-
tions and evolution of modern computing paradigms:
Cloud, iot, edge, and fog. IEEE Access, 7:150936–
150948.
Deb, K., Pratap, A., Agarwal, S., and Meyarivan, T. (2002).
A fast and elitist multiobjective genetic algorithm:
Nsga-ii. IEEE Transactions on Evolutionary Compu-
tation, 6(2):182–197.
Dijkstra, E. W. et al. (1959). A note on two problems
in connexion with graphs. Numerische mathematik,
1(1):269–271.
Faticanti, F., Savi, M., Pellegrini, F. D., Kochovski, P.,
Stankovski, V., and Siracusa, D. (2020). Deployment
of application microservices in multi-domain feder-
ated fog environments. In 2020 International Con-
ference on Omni-layer Intelligent Systems (COINS),
pages 1–6.
Hagberg, A., Swart, P., and S Chult, D. (2008). Explor-
ing network structure, dynamics, and function using
networkx. Technical report, Los Alamos National
Lab.(LANL), Los Alamos, NM (United States).
Ilie, L., Pop, E., Caramihai, S. I., and Moisescu, M. A.
(2022). A soa based e-health services framework.
In 2022 E-Health and Bioengineering Conference
(EHB), pages 1–4.
Katsuno, Y. and Takahashi, H. (2015). An automated par-
allel approach for rapid deployment of composite ap-
plication servers. In 2015 IEEE International Confer-
ence on Cloud Engineering, pages 126–134.
Kozina, O. and Kozin, M. (2022). Simulation model of data
consistency protocol for multicloud systems. In 2022
IEEE 3rd KhPI Week on Advanced Technology (KhPI-
Week), pages 1–4.
Li, Y., Dai, W., Gan, X., Jin, H., Fu, L., Ma, H., and
Wang, X. (2022). Cooperative service placement
and scheduling in edge clouds: A deadline-driven ap-
proach. IEEE Transactions on Mobile Computing,
21(10):3519–3535.
Liu, J., Qiao, J., and Zhao, J. (2018). Femcra: Fine-grained
elasticity measurement for cloud resources allocation.
In 2018 IEEE 11th International Conference on Cloud
Computing (CLOUD), pages 732–739.
Mahmud, R., Srirama, S. N., Ramamohanarao, K., and
Buyya, R. (2020). Profit-aware application place-
CLOSER 2023 - 13th International Conference on Cloud Computing and Services Science
304