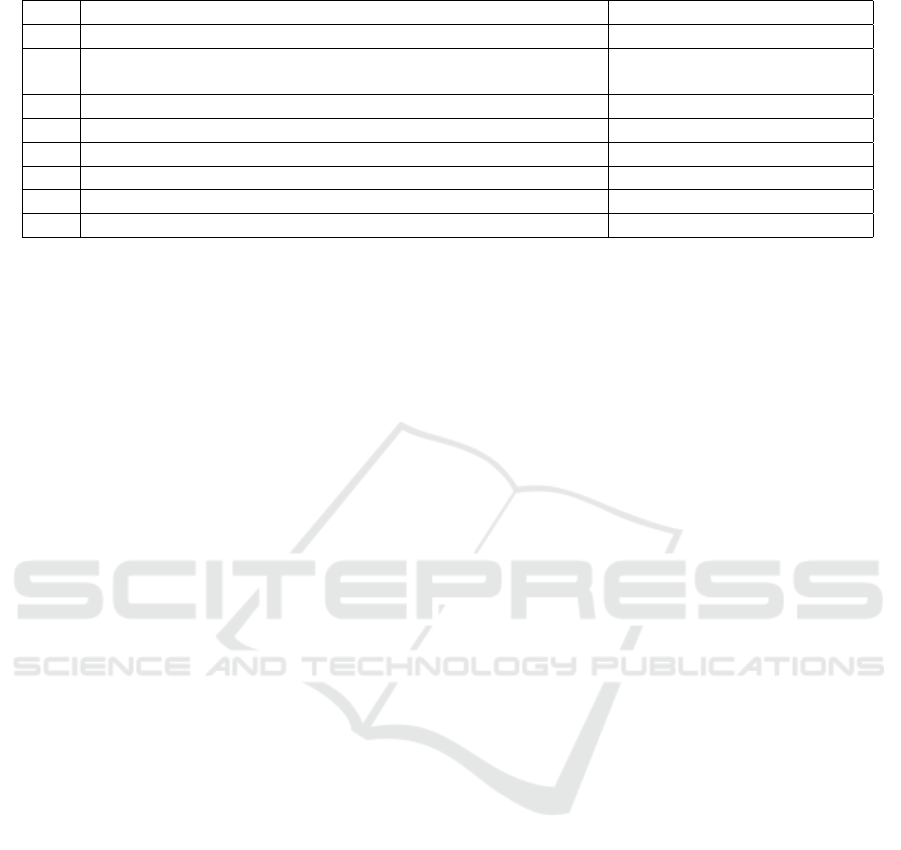
Table 7: Final threats from the automotive and web domains that we could not match to any LINDDUN threat.
F LB S
f
13a
Failure to meet contractual requirements with driver p
27a
f
41a
Violation of rules and regulations/Breach of legislation/
Abuse of driver personal data
p
28a
f
2w
Consent-related issues with driver rename(embrace(p
4w
, p
17w
))
f
4w
Inability of driver to access and modify data p
9w
f
7w
Insufficient data breach response from OEM p
3w
f
11w
Misleading content in OEM services p
16w
f
13w
Secondary use of driver data p
19w
f
14w
Sharing, transfer or processing through 3rd party of driver data rename(embrace(p
12w
, p
15w
))
threats from Section 4.1 are referred to as f
i
a, whilst
those from Section 4.2 are indicated as f
i
w, to distin-
guish the a(utomotive) domain from the w(eb appli-
cation) one. By taking off the phrases in italics, we
get a list of threats that are general enough to become
valid candidates as new nodes in the pertaining threat
tree(s) of an amended LINDDUN methodology. This
answers SRQ1, which required the execution of our
systematic method up to its final step.
This paper faced the challenge of threat modelling
in the automotive domain in two ways. It questioned
whether LINDDUN could suffice as an abstract-level
methodology, concluding that it may have to be ex-
tended with 8 new threats, thereby effectively answer-
ing SRQ1. It questioned how to build a list of de-
tailed threats in the same domain ensuring that the
list is complete with respect to chosen relevant best
practices, concluding with a list of 56 detailed, final
threats, thereby effectively answering SRQ2.
The paper has remarked consistently that its find-
ings are biased by the authors’ subjectivity. However,
all identified threats remain valid candidates for the
international community’s evaluation. While it seems
a stretch to imagine that the analyst’s role may be
emptied entirely, our future research looks at modern,
intelligent techniques from the area of Natural Lan-
guage Processing to improve the formalisation of the
various operations made through the steps of our sys-
tematic method. In particular, the upcoming steps in-
volve the application of Semantic Similarity to score
the relationship between threats based on their seman-
tic, hence ultimately reducing subjectivity.
REFERENCES
Bella, G., Biondi, P., and Tudisco, G. (2023). A double as-
sessment of privacy risks aboard top-selling cars. Au-
tomotive Innovation.
Chah, B., Lombard, A., Bkakria, A., Yaich, R., Abbas-
Turki, A., and Galland, S. (2022). Privacy threat anal-
ysis for connected and autonomous vehicles. Pro-
cedia Computer Science, 210:36–44. The 13th In-
ternational Conference on Emerging Ubiquitous Sys-
tems and Pervasive Networks (EUSPN) / The 12th In-
ternational Conference on Current and Future Trends
of Information and Communication Technologies in
Healthcare (ICTH-2022) / Affiliated Workshops.
Deng, M., Wuyts, K., Scandariato, R., Preneel, B., and
Joosen, W. (2011). A privacy threat analysis frame-
work: supporting the elicitation and fulfillment of
privacy requirements. Requirements Engineering,
16(1):3–32.
ENISA (2019). Good Practices for Security of Smart Cars.
https://www.enisa.europa.eu/publications/smart-cars.
GDPR (2016). Regulation (EU) 2016/679 General Data
Protection Regulation. https://eur-lex.europa.eu/legal-
content/EN/TXT/?uri=celex%3A32016R0679.
Microsoft (2009). The stride threat model.
OWASP (2021). Top 10 Privacy Risks.
https://owasp.org/www-project-top-10-privacy-risks/.
Pompigna, A. and Mauro, R. (2022). Smart roads: A state
of the art of highways innovations in the smart age.
Engineering Science and Technology, an International
Journal, 25:100986.
Raciti, M. and Bella, G. (2023). Github
repository with complete outcomes.
https://github.com/tsumarios/LINDDUN-threats-
completeness.
Toh, Sanguesa, C. and Martinez (2020). Advances in smart
roads for future smart cities. In Proceedings of the
Royal Society A: Mathematical, Physical and Engi-
neering Sciences, volume 476, 2233.
Van Landuyt, D. and Joosen, W. (2020). A descriptive
study of assumptions made in linddun privacy threat
elicitation. In Proceedings of the 35th Annual ACM
Symposium on Applied Computing, SAC ’20, page
1280–1287, New York, NY, USA. Association for
Computing Machinery.
Vasenev., A., Stahl., F., Hamazaryan., H., Ma., Z., Shan., L.,
Kemmerich., J., and Loiseaux., C. (2019). Practical
security and privacy threat analysis in the automotive
domain: Long term support scenario for over-the-air
updates. In Proceedings of the 5th International Con-
ference on Vehicle Technology and Intelligent Trans-
port Systems - VEHITS,, pages 550–555. INSTICC,
SciTePress.
How to Model Privacy Threats in the Automotive Domain
401