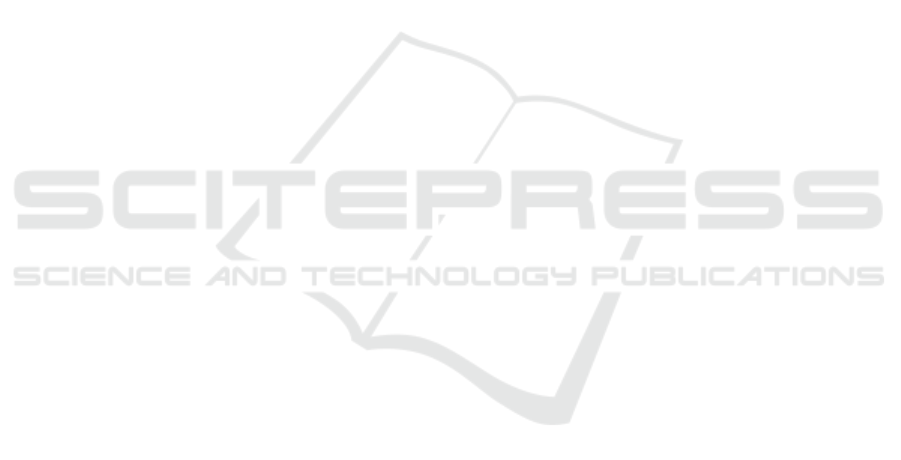
part 3-3: Application guide – life cycle costing. Stan-
dard, IEC.
ISO 13372:2012(E/F) (2012). Condition monitoring and
diagnostics of machines – vocabulary. Standard, ISO.
Javed, K., Gouriveau, R., Zerhouni, N., Zemouri, R., and
Li, X. (2012). Robust, reliable and applicable tool
wear monitoring and prognostic: Approach based on
an improved-extreme learning machine. In Confer-
ence on Prognostics and Health Management, pages
1–9. IEEE.
Kanungo, T., Mount, D. M., Netanyahu, N. S., Piatko,
C. D., Silverman, R., and Wu, A. Y. (2002). An effi-
cient k-means clustering algorithm: Analysis and im-
plementation. Transactions on Pattern Analysis and
Machine Intelligence, 24(7):881–892.
Kitchenham, B. A., Budgen, D., and Brereton, O. P. (2015).
Evidence-Based Software Engineering and Systematic
Reviews. Taylor and Francis.
Kotsiopoulos, T., Leontaris, L., Dimitriou, N., Ioanni-
dis, D., Oliveira, F., Sacramento, J., Amanatiadis,
S., Karagiannis, G., Votis, K., and Tzovaras, D.
(2021). Deep multi-sensorial data analysis for produc-
tion monitoring in hard metal industry. The Interna-
tional Journal of Advanced Manufacturing Technol-
ogy, 115(3):823–836.
Krishna, M. and Kannadaguli, P. (2020). Iot based cnc
machine condition monitoring system using machine
learning techniques. In Conference on Communica-
tion Systems and Network Technologies, pages 61–65.
IEEE.
Li, G., Wang, Y., Wang, J., He, J., and Huo, Y. (2021).
Tool wear prediction based on multidomain feature
fusion by attention-based depth-wise separable con-
volutional neural network in manufacturing. The In-
ternational Journal of Advanced Manufacturing Tech-
nology, pages 1–18.
Luo, B., Wang, H., Liu, H., Li, B., and Peng, F. (2018).
Early fault detection of machine tools based on deep
learning and dynamic identification. Transactions on
Industrial Electronics, 66(1):509–518.
Lutz, B., Kisskalt, D., Regulin, D., Reisch, R., Schiffler,
A., and Franke, J. (2019). Evaluation of deep learn-
ing for semantic image segmentation in tool condition
monitoring. In International Conference on Machine
Learning and Applications, pages 2008–2013. IEEE.
Mobley, R. K. (2002). An Introduction to Predictive Main-
tenance. Elsevier.
Mohanraj, T., Shankar, S., Rajasekar, R., Sakthivel, N. R.,
and Pramanik, A. (2020). Tool condition monitoring
techniques in milling process – a review. Journal of
Materials Research and Technology, 9(1):1032–1042.
Monostori, L., K
´
ad
´
ar, B., Bauernhansl, T., Kondoh, S., Ku-
mara, S., Reinhart, G., Sauer, O., Schuh, G., Sihn, W.,
and Ueda, K. (2016). Cyber-physical systems in man-
ufacturing. CIRP Annals, 65(2):621–641.
Motaghare, O., Pillai, A. S., and Ramachandran, K. (2018).
Predictive maintenance architecture. In International
Conference on Computational Intelligence and Com-
puting Research, pages 1–4. IEEE.
Patil, R. B., Patil, M. A., Ravi, V., and Naik, S. (2017). Pre-
dictive modeling for corrective maintenance of imag-
ing devices from machine logs. In International Con-
ference of the IEEE Engineering in Medicine and Bi-
ology Society, pages 1676–1679. IEEE.
Qiao, H., Wang, T., and Wang, P. (2020). A tool wear moni-
toring and prediction system based on multiscale deep
learning models and fog computing. The Interna-
tional Journal of Advanced Manufacturing Technol-
ogy, 108:2367–2384.
Schuh, G. and Scholz, P. (2019). Development of a frame-
work for the systematic identification of ai applica-
tion patterns in the manufacturing industry. In Inter-
national Conference on Management of Engineering
and Technology, pages 1–8. IEEE.
Schuh, G., Scholz, P., Leich, T., and May, R. (2020). Iden-
tifying and analyzing data model requirements and
technology potentials of machine learning systems in
the manufacturing industry of the future. In Interna-
tional Scientific Conference on Information Technol-
ogy and Management Science, pages 1–10. IEEE.
Schuh, G., Scholz, P., Schorr, S., Harman, D., M
¨
oller, M.,
Heib, J., and B
¨
ahre, D. (2019). Prediction of work-
piece quality: An application of machine learning in
manufacturing industry. In International Conference
on Computer Science, Engineering and Information
Technology, pages 189–202. CS & IT.
Serin, G., Sener, B., Ozbayoglu, A. M., and Unver, H. O.
(2020). Review of tool condition monitoring in ma-
chining and opportunities for deep learning. The In-
ternational Journal of Advanced Manufacturing Tech-
nology, 109(3):953–974.
Sevic, M. and Keller, P. (2019). Design of cnc milling ma-
chine as a base of industry 4.0 enterprise. MM Science
Journal, 12:3555–3560.
Soother, D. K. and Daudpoto, J. (2019). A brief review
of condition monitoring techniques for the induction
motor. Transactions of the Canadian Society for Me-
chanical Engineering, 43(4):499–508.
Tang, L.-A., Han, J., and Jiang, G. (2014). Mining sensor
data in cyber-physical systems. Tsinghua Science and
Technology, 19(3):225–234.
Theissler, A., P
´
erez-Vel
´
azquez, J., Kettelgerdes, M., and
Elger, G. (2021). Predictive maintenance enabled by
machine learning: Use cases and challenges in the au-
tomotive industry. Reliability Engineering & System
Safety, 215:107864.
VDI/VDE 2651 Part 1:2017-05 (2017). Plant asset manage-
ment (pam) in the process industry definition, model,
task, benefit. Standard, VDI/VDE-Gesellschaft Mess-
und Automatisierungstechnik (GMA).
Wang, J., Ma, Y., Zhang, L., Gao, R. X., and Wu, D. (2018).
Deep learning for smart manufacturing: Methods
and applications. Journal of Manufacturing Systems,
48:144–156.
Wuest, T., Weimer, D., Irgens, C., and Thoben, K. D.
(2016). Machine learning in manufacturing: Ad-
vantages, challenges, and applications. International
Journal of Production Research and Manufacturing
Research, 4(1):23–45.
Zonta, T., da Costa, C. A., da Rosa Righi, R., de Lima,
M. J., da Trindade, E. S., and Li, G. P. (2020). Pre-
dictive maintenance in the industry 4.0: A systematic
literature review. Computers & Industrial Engineer-
ing, 150:106889.
ICSOFT 2023 - 18th International Conference on Software Technologies
216