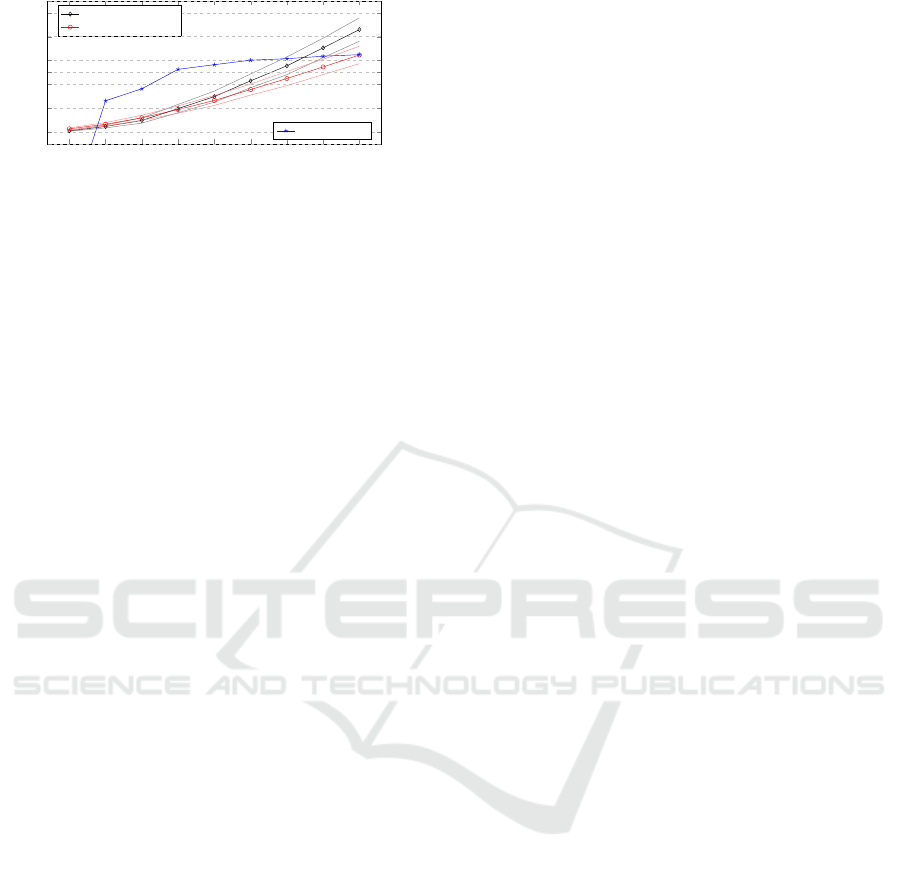
2 3 4
5 6
7 8 9 10
0
100
200
300
400
500
Group-size
Effort to reach consensus (E)
Effort without artefact
Effort using artefact
−100
−50
0
50
100
% improvement
% Improvement
Figure 14: (Effort to reach consensus with and without an
artefact. The bands indicate 1-σ, n=500.
4-group needs (on average) 42.8 actions without an
artefact and 60.4 actions with an artefact to reach con-
sensus, a 3-group needs 20.9 and 31.3 actions without
and with an artefact, and a 2-group needs 3.3 actions
without and 12.6 actions with an artefact.
On the other hand, any group with size larger than
5 show significant improvement in time t
max
when us-
ing an artefact, as can be seen from the % improve-
ment plotted in Figure 13 and Figure 14.
5 CONCLUSION
In this paper we described a simulator for studying or-
ganisational structure with the aim to model complex
organisations and the effects of team structure, organ-
isational structure and the use of artefacts to improve
project delivery. We presented theoretical and statisti-
cal models for polyarchical structures. We presented
the simulation results for modelling polyarchical or-
ganisations of various sizes.
Some of the interesting results we found was that
for a given problem size, a team of 6 will need ap-
proximately 10 times longer to reach consensus what
would a team of 2. A team of 10 will need 100 times
the effort to reach consensus compared to a team of
2. The use of artefacts to facilitate consensus discus-
sions greatly improve the time and effort needed to
reach consensus if the group is bigger than 5. Finally,
if the problem has a fixed size, then there is an upper
bound on the time needed to reach consensus, no mat-
ter how many people are involved (on the assumption
that every one is cooperative).
REFERENCES
Birko, S., Dove, E. S., and
¨
Ozdemir, V. (2015). Evaluation
of nine consensus indices in delphi foresight research
and their dependency on delphi survey characteristics:
a simulation study and debate on delphi design and
interpretation. PloS one, 10(8):e0135162.
Carter, D. R., DeChurch, L. A., Braun, M. T., and Con-
tractor, N. S. (2015). Social network approaches to
leadership: An integrative conceptual review. Journal
of Applied Psychology, 100(3):597–622.
Chang, M.-H. and Harrington, J. E. (2000). Centralization
vs. decentralization in a multi-unit organization: A
computational model of a retail chain as a multi-agent
adaptive system. Management Science, 46(11):1427–
1440.
Christensen, M. and Knudsen, T. (2010). Design of
decision-making organizations. Management Science,
56(1):71–89.
Den Boon, A. K. and Van Meurs, A. (1991). Measuring
opinion distributions: An instrument for the measure-
ment of perceived opinion distributions. Quality and
Quantity, 25(4):359–379.
Jones, S. L. and Shah, P. P. (2016). Diagnosing the locus of
trust: A temporal perspective for trustor, trustee and
dyadic influences on perceived trustworthiness. Jour-
nal of Applied Psychology, 101:392–414.
Keupp, M. M., Palmi
´
e, M., and Gassmann, O. (2012). The
strategic management of innovation: A systematic re-
view and paths for future research. International jour-
nal of management reviews, 14(4):367–390.
Kian, M. E., Sun, M., and Bosch
´
e, F. (2016). A consistency-
checking consensus-building method to assess com-
plexity of energy megaprojects. Procedia-social and
behavioral sciences, 226:43–50.
Lang, J. W., Bliese, P. D., and de Voogt, A. (2018). Mod-
eling consensus emergence in groups using longi-
tudinal multilevel methods. Personnel Psychology,
71(2):255–281.
Reagans, R., Miron-Spektor, E., and Argote, L. (2016).
Knowledge utilization, coordination, and team perfor-
mance. Organization Science, 27(5):1108–1124.
Rosell
´
o, L., Prats, F., Agell, N., and S
´
anchez, M. (2010).
Measuring consensus in group decisions by means of
qualitative reasoning. International Journal of Ap-
proximate Reasoning, 51(4):441–452.
S
´
aenz-Royo, C. and Lozano-Rojo, A. (2023). Authoritar-
ianism versus participation in innovation decisions.
Technovation, 124:102741.
Wei, Q., Wang, X., Zhong, X., and Wu, N. (2021). Consen-
sus control of leader-following multi-agent systems
in directed topology with heterogeneous disturbances.
IEEE/CAA Journal of Automatica Sinica, 8(2):423–
431.
Will, M. G., Al-Kfairy, M., and Mellor, R. B. (2019). How
organizational structure transforms risky innovations
into performance–a computer simulation. Simulation
Modelling Practice and Theory, 94:264–285.
Yan, H.-B., Ma, T., and Huynh, V.-N. (2017). On qualitative
multi-attribute group decision making and its consen-
sus measure: A probability based perspective. Omega,
70:94–117.
Young-Hyman, T. (2017). Cooperating without co-
laboring: How formal organizational power moder-
ates cross-functional interaction in project teams. Ad-
ministrative Science Quarterly, 62(1):179–214.
SIMULTECH 2023 - 13th International Conference on Simulation and Modeling Methodologies, Technologies and Applications
26