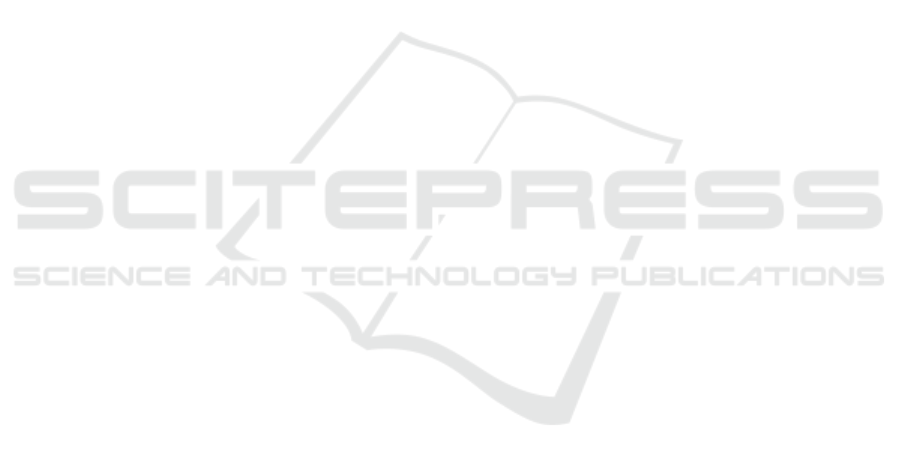
braking behavior in the network. Overall, the method-
ology provides a valuable tool for analyzing braking
behavior and identifying potential hotspots for safety
concerns.
During our investigation into the real-world dy-
namics and potential of data analytics in an urban traf-
fic grid, we encountered the challenge of sparse data
caused by the limited deployment of Connected Ve-
hicle (V2X) technology. Nevertheless, this research
sheds light on the enormous potential of utilizing
Connected Vehicle data to optimize traffic flow and
improve road safety, opening up a promising future
for transportation and traffic management.
REFERENCES
Baldanzini, N., Huertas Leyva, P., Savino, G., and Pierini,
M. (2016). Rider behavioral patterns in braking
manoeuvres. Transportation Research Procedia,
14:4374–4383.
Banerjee, T., Chen, K., Almaraz, A., Sengupta, R., Kar-
nati, Y., Grame, B., Posadas, E., Poddar, S., Schenck,
R., Dilmore, J., Srinivasan, S., Rangarajan, A., and
Ranka, S. (2022). A modern intersection data ana-
lytics system for pedestrian and vehicular safety. In
2022 IEEE 25th International Conference on Intel-
ligent Transportation Systems (ITSC), pages 3117–
3124.
Biddlestone, S., Redmill, K., Miucic, R., and Ozguner,
¨
U.
(2012). An integrated 802.11p wave dsrc and vehicle
traffic simulator with experimentally validated urban
(los and nlos) propagation models. IEEE Transactions
on Intelligent Transportation Systems, 13(4):1792–
1802.
D’Andrea, E. and Marcelloni, F. (2017). Detection of traf-
fic congestion and incidents from gps trace analysis.
Expert Systems with Applications, 73:43–56.
DSRC (2020). DSRC Technical Committee On-Board Sys-
tem Requirements for V2V Safety Communications.
FDOT. Intersection operations and safety, https://www.fdot
.gov/traffic/trafficservices/intersection-operations.sh
tm.
FHWA. Intersection safety, https://highways.dot.gov/safet
y/intersection-safety.
Haas, C., Mahmassani, H., Khoury, J., Haynes, M., Rioux,
T., and Logman, H. (2001). Evaluation of automatic
vehicle identification for san antonio’s transguide for
incident detection and advanced traveler information
systems.
Hofmann-Wellenhof, B., Lichtenegger, H., and Collins, J.
(2012). Global positioning system: theory and prac-
tice. Springer Science & Business Media.
Hsu, M. and Shih, A. (2015). A traffic signal control mech-
anism in a connected vehicle environment. In 2015
IEEE International Conference on Consumer Elec-
tronics - Taiwan, pages 256–257.
Huang, X., He, P., Rangarajan, A., and Ranka, S. (2020). In-
telligent intersection: Two-stream convolutional net-
works for real-time near-accident detection in traffic
video. ACM Transactions on Spatial Algorithms and
Systems (TSAS), 6(2):1–28.
Kan, Z., Tang, L., Kwan, M.-P., Ren, C., Liu, D., and Li,
Q. (2019). Traffic congestion analysis at the turn level
using taxis’ gps trajectory data. Computers, Environ-
ment and Urban Systems, 74:229–243.
Kenney, J. B. (2011). Dedicated short-range communica-
tions (dsrc) standards in the united states. Proceedings
of the IEEE, 99(7):1162–1182.
Lamas, J., Castro-Castro, P.-M., Dapena, A., and Vazquez-
Araujo, F. (2015). Vehicle classification using the dis-
crete fourier transform with traffic inductive sensors.
Sensors, 15.
Li, P., Abdel-Aty, M., and Yuan, J. (2021). Using bus crit-
ical driving events as surrogate safety measures for
pedestrian and bicycle crashes based on gps trajectory
data. Accident Analysis & Prevention, 150:105924.
Liu, Y., Jia, R., Ye, J., and Qu, X. (2022). How ma-
chine learning informs ride-hailing services: A sur-
vey. Communications in Transportation Research,
2:100075.
Mandava, S., Boriboonsomsin, K., and Barth, M. (2009).
Arterial velocity planning based on traffic signal in-
formation under light traffic conditions. In 2009 12th
International IEEE Conference on Intelligent Trans-
portation Systems, pages 1–6.
Staputis, A. and
ˇ
Zuraulis, V. (2023). Research of efficiency
of anti-lock braking system during emergency corner-
ing manoeuver. In TRANSBALTICA XIII: Transporta-
tion Science and Technology: Proceedings of the 13th
International Conference TRANSBALTICA, Septem-
ber 15-16, 2022, Vilnius, Lithuania, pages 224–235.
Springer.
Sun, S., Chen, J., and Sun, J. (2019). Traffic conges-
tion prediction based on gps trajectory data. In-
ternational Journal of Distributed Sensor Networks,
15(5):1550147719847440.
Wang, X., Sanner, S., and Abdulhai, B. (2022). A criti-
cal review of traffic signal control and a novel unified
view of reinforcement learning and model predictive
control approaches for adaptive traffic signal control.
arXiv preprint arXiv:2211.14426.
Wang, Y., Cao, J., Li, W., and Gu, T. (2016). Mining traf-
fic congestion correlation between road segments on
gps trajectories. In 2016 IEEE International Confer-
ence on Smart Computing (SMARTCOMP), pages 1–
8. IEEE.
Wolf, J., Bachman, W., Oliveira, M., Auld, J., Mohamma-
dian, A. K., Vovsha, P., and Zmud, J. (2014). Apply-
ing gps data to understand travel behavior, volume ii:
guidelines. Technical report.
Yong-chuan, Z., Xiao-qing, Z., Zhen-ting, C., et al. (2011).
Traffic congestion detection based on gps floating-car
data. Procedia Engineering, 15:5541–5546.
Zhao, D., Dai, Y., and Zhang, Z. (2012). Computational
Intelligence in Urban Traffic Signal Control: A Sur-
vey. IEEE Transactions on Systems, Man, and Cyber-
netics, Part C (Applications and Reviews), 42(4):485–
494.
Using DSRC Road-Side Unit Data to Derive Braking Behavior
427