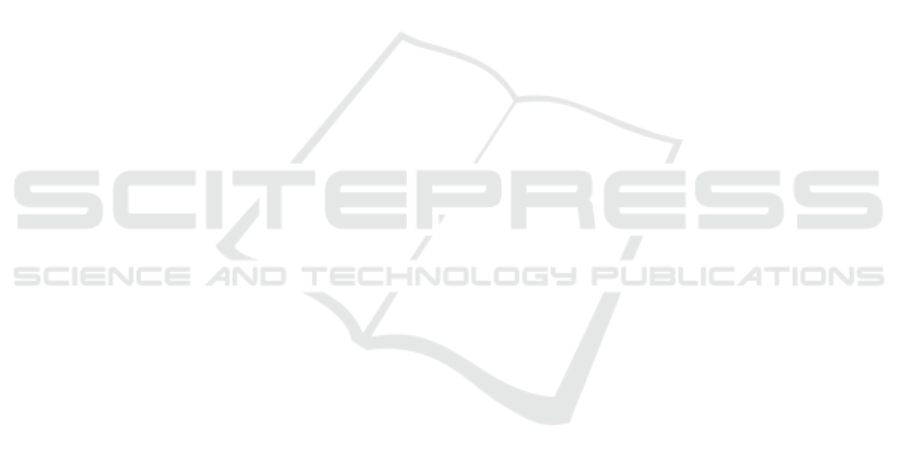
ACKNOWLEDGEMENTS
This work has been part supported by the visuAAL
project on Privacy-Aware and Acceptable Video-
Based Technologies and Services for Active and As-
sisted Living (https://www.visuaal-itn.eu/) funded by
the EU H2020 Marie Skłodowska-Curie grant agree-
ment No. 861091. The project has also been
part supported by the SFI Future Innovator Award
SFI/21/FIP/DO/9955 project Smart Hangar. Thanks
also to Luke Casey and Chizubere Lovelyn Ulogwara
for their help in deep learning and data capture.
REFERENCES
Alam, E., Sufian, A., Dutta, P., and Leo, M. (2022). Vision-
based human fall detection systems using deep learn-
ing: A review. Computers in Biology and Medicine,
146:105626.
An, J., Kim, J., Lee, H., Kim, J., Kang, J., Kim, M., Shin, S.,
Kim, M., Hong, D., and Woo, S. S. (2021). VFP290K:
A Large-Scale Benchmark Dataset for Vision-based
Fallen Person Detection. In Thirty-Fifth Conference
on Neural Information Processing Systems Datasets
and Benchmarks Track (Round 2).
Antonello, M., Carraro, M., Pierobon, M., and Menegatti,
E. (2017). Fast and robust detection of fallen people
from a mobile robot. In 2017 IEEE/RSJ International
Conference on Intelligent Robots and Systems (IROS),
pages 4159–4166.
Ariani, A., Redmond, S. J., Chang, D., and Lovell, N. H.
(2010). Software simulation of unobtrusive falls de-
tection at night-time using passive infrared and pres-
sure mat sensors. In 2010 Annual international con-
ference of the IEEE engineering in medicine and biol-
ogy, pages 2115–2118. IEEE.
Asif, U., Mashford, B., Von Cavallar, S., Yohanandan, S.,
Roy, S., Tang, J., and Harrer, S. (2020). Privacy Pre-
serving Human Fall Detection using Video Data. In
Dalca, A. V., McDermott, M. B., Alsentzer, E., Fin-
layson, S. G., Oberst, M., Falck, F., and Beaulieu-
Jones, B., editors, Proceedings of the Machine Learn-
ing for Health NeurIPS Workshop, volume 116 of Pro-
ceedings of Machine Learning Research, pages 39–
51. PMLR.
Auvinet, E., Rougier, C., Meunier, J., St-Arnaud, A., and
Rousseau, J. (2010). Multiple cameras fall dataset.
DIRO-Université de Montréal, Tech. Rep, 1350:24.
Cao, Z., Hidalgo, G., Simon, T., Wei, S.-E., and Sheikh,
Y. (2018). Openpose: Realtime multi-person 2d pose
estimation using part affinity fields.
Charfi, I., Miteran, J., Dubois, J., Atri, M., and Tourki, R.
(2012). Definition and performance evaluation of a ro-
bust svm based fall detection solution. In 2012 eighth
international conference on signal image technology
and internet based systems, pages 218–224. IEEE.
Charfi, I., Miteran, J., Dubois, J., Atri, M., and Tourki,
R. (2013). Optimized spatio-temporal descriptors for
real-time fall detection: comparison of support vector
machine and Adaboost-based classification. Journal
of Electronic Imaging, 22(4):041106.
Chen, Y., Kong, X., Meng, L., and Tomiyama, H. (2020).
An Edge Computing Based Fall Detection System for
Elderly Persons. Procedia Computer Science, 174:9–
14.
Deng, J., Dong, W., Socher, R., Li, L.-J., Li, K., and Fei-
Fei, L. (2009). Imagenet: A large-scale hierarchical
image database. In 2009 IEEE conference on com-
puter vision and pattern recognition, pages 248–255.
Ieee.
Duan, K., Bai, S., Xie, L., Qi, H., Huang, Q., and Tian, Q.
(2019). Centernet: Keypoint triplets for object detec-
tion. In Proceedings of the IEEE/CVF international
conference on computer vision, pages 6569–6578.
Dwyer, B. and Nelson, J. (2022). Roboflow (version 1.0)
[software].
Feng, Q., Gao, C., Wang, L., Zhao, Y., Song, T., and Li, Q.
(2020). Spatio-temporal fall event detection in com-
plex scenes using attention guided LSTM. Pattern
Recognition Letters, 130:242–249.
Gutiérrez, J., Rodríguez, V., and Martin, S. (2021). Com-
prehensive Review of Vision-Based Fall Detection
Systems. Sensors, 21(3):947.
Howard, A. G., Zhu, M., Chen, B., Kalenichenko, D.,
Wang, W., Weyand, T., Andreetto, M., and Adam,
H. (2017). Mobilenets: Efficient convolutional neu-
ral networks for mobile vision applications. arXiv
preprint arXiv:1704.04861.
Iuga, C., Dr
˘
agan, P., and Bus
,
oniu, L. (2018). Fall moni-
toring and detection for at-risk persons using a UAV.
IFAC-PapersOnLine, 51(10):199–204.
Lafuente-Arroyo, S., Martín-Martín, P., Iglesias-Iglesias,
C., Maldonado-Bascón, S., and Acevedo-Rodríguez,
F. J. (2022). RGB camera-based fallen person detec-
tion system embedded on a mobile platform. Expert
Systems with Applications, 197:116715.
Lin, T.-Y., Maire, M., Belongie, S., Hays, J., Perona, P.,
Ramanan, D., Dollár, P., and Zitnick, C. L. (2014).
Microsoft coco: Common objects in context. In Com-
puter Vision–ECCV 2014: 13th European Confer-
ence, Zurich, Switzerland, September 6-12, 2014, Pro-
ceedings, Part V 13, pages 740–755. Springer.
Maldonado-Bascón, S., Iglesias-Iglesias, C., Martín-
Martín, P., and Lafuente-Arroyo, S. (2019). Fallen
People Detection Capabilities Using Assistive Robot.
Electronics, 8(9):915.
Maldonado-Bascon, S., Iglesias-Iglesias, C., Martín-
Martín, P., and Lafuente-Arroyo, S. (2019). Fallen
people detection capabilities using assistive robot.
Electronics, 8(9):915.
Mann, W. C., Belchior, P., Tomita, M. R., and Kemp, B. J.
(2005). Use of personal emergency response systems
by older individuals with disabilities. Assistive tech-
nology, 17(1):82–88.
Ramachandran, A. and Karuppiah, A. (2020). A survey
on recent advances in wearable fall detection systems.
BioMed research international, 2020.
ICT4AWE 2023 - 9th International Conference on Information and Communication Technologies for Ageing Well and e-Health
268