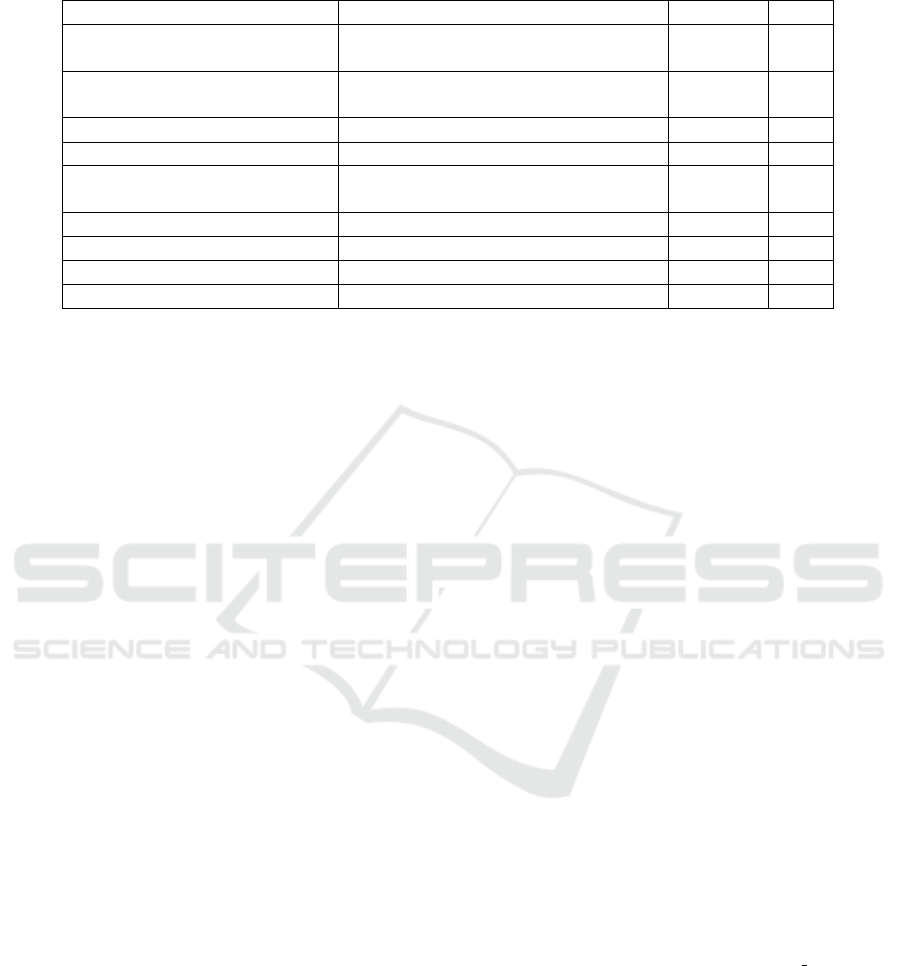
Table 3: Illustrative overview of the different methods available in the specialized literature to investigate the context of
radiographic images of COVID-19.
Reference Approach ACC (%) AUC
(Tuncer et al., 2020)
ResExLBP, RelieF,
SVM
100% -
(Kedia et al., 2021)
CoVNet-19, Ensemble Learning,
Transfer learning
99.71% 0.99
(Ashour et al., 2021)
Bag of features, Ensemble
98.60% 0.98
(Deb et al., 2022) Multi model ensemble architecture 98.58% 0.95
Proposed
Transfer learning, DenseNet-201,
RelieF, SMO
98.38% 0.98
(Walvekar et al., 2020)
ResNet-50
96.23% 0.96
(Shamila Ebenezer et al., 2022)
EfficientNet-b0, Image Enchancement
94.56% 0.93
(Rajpal et al., 2021)
Handpicked Features, ResNet-50
94.40% 0.97
(Hemdan et al., 2020)
DenseNet-121, VGG19
90.00% 0.90
ACKNOWLEDGEMENTS
This study was financed in part by the: National
Council for Scientific and Technological Develop-
ment CNPq (Grants #313643/2021-0, #311404/2021-
9 and #120993/2020-1); State of Minas Gerais Re-
search Foundation - FAPEMIG (Grant #APQ-00578-
18); WZTECH NETWORKS, S
˜
ao Jos
´
e do Rio Preto,
S
˜
ao Paulo.
REFERENCES
Ashour, A. S., Eissa, M. M., Wahba, M. A., Elsawy, R. A.,
Elgnainy, H. F., Tolba, M. S., and Mohamed, W. S.
(2021). Ensemble-based bag of features for auto-
mated classification of normal and covid-19 cxr im-
ages. Biomedical Signal Processing and Control,
68:102656.
Atkeson, C., Moore, A., and Schaal, S. (1996). Locally
weighted learning. AI Review.
Breiman, L. (2001). Random forests. Machine learning,
45(1):5–32.
Cohen, J. P., Morrison, P., and Dao, L. (2020). Covid-19
image data collection. arXiv 2003.11597.
Deb, S. D., Jha, R. K., Jha, K., and Tripathi, P. S. (2022). A
multi model ensemble based deep convolution neural
network structure for detection of covid19. Biomedi-
cal Signal Processing and Control, 71:103126.
Deng, J., Dong, W., Socher, R., Li, L.-J., Li, K., and Fei-
Fei, L. (2009). Imagenet: A large-scale hierarchical
image database. In 2009 IEEE conference on com-
puter vision and pattern recognition, pages 248–255.
Ieee.
Fomin, V., Anmol, J., Desroziers, S., Kriss, J., and Te-
jani, A. (2020). High-level library to help with train-
ing neural networks in pytorch. https://github.com/
pytorch/ignite.
Frank, E., Hall, M., and Pfahringer, B. (2003). Locally
weighted naive bayes. In 19th Conference in Uncer-
tainty in Artificial Intelligence, pages 249–256. Mor-
gan Kaufmann.
Frank, E. and Kirkby, R. (2023).
RandomTree Classifier. Weka.
https://weka.sourceforge.io/doc.dev/weka/classifiers/
trees/randomtree.html.
Hall, M., Frank, E., Holmes, G., Pfahringer, B., Reutemann,
P., and Witten, I. H. (2009). The weka data min-
ing software: An update. SIGKDD Explor. Newsl.,
11(1):10–18.
He, K., Zhang, X., Ren, S., and Sun, J. (2016). Deep resid-
ual learning for image recognition. In Proceedings of
the IEEE conference on computer vision and pattern
recognition, pages 770–778.
Hemdan, E. E.-D., Shouman, M. A., and Karar, M. E.
(2020). Covidx-net: A framework of deep learning
classifiers to diagnose covid-19 in x-ray images.
Huang, G., Liu, Z., van der Maaten, L., and Weinberger,
K. Q. (2016). Densely connected convolutional net-
works.
Kedia, P., Anjum, and Katarya, R. (2021). Covnet-19:
A deep learning model for the detection and anal-
ysis of covid-19 patients. Applied Soft Computing,
104:107184.
Organization, W. H. (2023). Coronavirus disease (COVID-
19). World Health Organization. https://www.who.
int/health-topics/coronavirus\#tab=tab\ 1.
Paszke, A., Gross, S., Massa, F., Lerer, A., Bradbury, J.,
Chanan, G., Killeen, T., Lin, Z., Gimelshein, N.,
Antiga, L., Desmaison, A., Kopf, A., Yang, E., De-
Vito, Z., Raison, M., Tejani, A., Chilamkurthy, S.,
Steiner, B., Fang, L., Bai, J., and Chintala, S. (2019).
Pytorch: An imperative style, high-performance deep
learning library. In Advances in Neural Information
Processing Systems 32, pages 8024–8035. Curran As-
sociates, Inc.
Pereira dos Santos, F. and Antonelli Ponti, M. (2019).
Alignment of local and global features from multiple
An Investigation of Deep-Learned Features for Classifying Radiographic Images of COVID-19
681