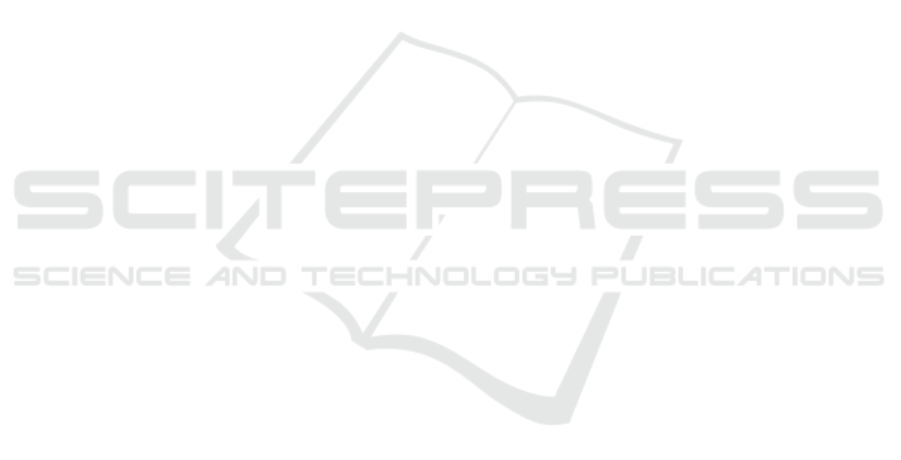
REFERENCES
Adomavicius, G. and Tuzhilin, A. (2005). Toward the
next generation of recommender systems: A sur-
vey of the state-of-the-art and possible extensions.
IEEE transactions on knowledge and data engineer-
ing, 17(6):734–749.
Blei, D. M., Ng, A. Y., and Jordan, M. I. (2003). Latent
Dirichlet Allocation. Journal of machine Learning re-
search, 3(Jan):993–1022.
Brank, J., Leban, G., and Grobelnik, M. (2017). Annotat-
ing documents with relevant Wikipedia concepts. Pro-
ceedings of SiKDD, 472.
Broisin, J., Brut, M., Butoianu, V., Sedes, F., and Vidal, P.
(2010). A personalized recommendation framework
based on cam and document annotations. Procedia
Computer Science, 1(2):2839–2848.
Cleuziou, G. and Flouvat, F. (2021). Learning student pro-
gram embeddings using abstract execution traces. In
14th International Conference on Educational Data
Mining, page 252–262.
Connes, V., de la Higuera, C., and Le Capitaine, H. (2021).
What should I learn next? ranking educational re-
sources. In 2021 IEEE 45th Annual Computers,
Software, and Applications Conference (COMPSAC),
page 109–114.
Crossley, S., Allen, L. K., Snow, E. L., and McNamara,
D. S. (2015). Pssst... textual features... there is more to
automatic essay scoring than just you! In Proceedings
of the Fifth International Conference on Learning An-
alytics And Knowledge, LAK ’15, page 203–207, New
York, NY, USA. Association for Computing Machin-
ery.
Devlin, J., Chang, M.-W., Lee, K., and Toutanova,
K. (2019). BERT: Pre-training of deep bidi-
rectional transformers for language understanding.
(arXiv:1810.04805). arXiv:1810.04805 [cs].
Drachsler, H., Verbert, K., Santos, O. C., and Manouselis,
N. (2015). Panorama of recommender systems to sup-
port learning, page 421–451. Springer.
Ferreira-Mello, R., Andr
´
e, M., Pinheiro, A., Costa, E., and
Romero, C. (2019). Text mining in education. WIREs
Data Mining and Knowledge Discovery, 9(6):e1332.
Hoffart, J., Yosef, M. A., Bordino, I., F
¨
urstenau, H., Pinkal,
M., Spaniol, M., Taneva, B., Thater, S., and Weikum,
G. (2011). Robust disambiguation of named entities
in text. In Proceedings of the 2011 conference on em-
pirical methods in natural language processing, page
782–792.
Hu, Z., Huang, P., Deng, Y., Gao, Y., and Xing, E. (2015).
Entity hierarchy embedding. In Proceedings of the
53rd Annual Meeting of the Association for Computa-
tional Linguistics and the 7th International Joint Con-
ference on Natural Language Processing (Volume 1:
Long Papers), page 1292–1300.
Khribi, M. K., Jemni, M., and Nasraoui, O. (2008). Au-
tomatic recommendations for e-learning personaliza-
tion based on web usage mining techniques and in-
formation retrieval. In 2008 Eighth IEEE Interna-
tional Conference on Advanced Learning Technolo-
gies, page 241–245.
Kirkwood, A. and Price, L. (2014). Technology-enhanced
learning and teaching in higher education: what is ‘en-
hanced’ and how do we know? a critical literature re-
view. Learning, media and technology, 39(1):6–36.
Le, Q. and Mikolov, T. (2014). Distributed representations
of sentences and documents. In Proceedings of the
31st International Conference on Machine Learning,
page 1188–1196. PMLR.
Lessa, L. F. and Brand
˜
ao, W. C. (2018). Filtering graduate
courses based on LinkedIn profiles. In Proceedings of
the 24th Brazilian Symposium on Multimedia and the
Web, page 141–147.
Li, J., Supraja, S., Qiu, W., and Khong, A. W. (2022). Grade
prediction via prior grades and text mining on course
descriptions: Course outlines and intended learning
outcomes. International Educational Data Mining So-
ciety.
Lin, F.-R., Hsieh, L.-S., and Chuang, F.-T. (2009). Dis-
covering genres of online discussion threads via text
mining. Computers & Education, 52(2):481–495.
Liu, Z., Lin, Y., and Sun, M. (2020). Representation learn-
ing for natural language processing. Springer Nature.
Ma, H., Wang, X., Hou, J., and Lu, Y. (2017). Course rec-
ommendation based on semantic similarity analysis.
In 2017 3rd IEEE International Conference on Con-
trol Science and Systems Engineering (ICCSSE), page
638–641. IEEE.
Merrill, W., Goldberg, Y., Schwartz, R., and Smith, N. A.
(2021). Provable limitations of acquiring meaning
from ungrounded form: What will future language
models understand? Transactions of the Association
for Computational Linguistics, 9:1047–1060.
Mukherjee, S. (2021). Sentiment Analysis, page 113–127.
Apress, Berkeley, CA.
Romero, C. and Ventura, S. (2013). Data mining in educa-
tion. WIREs Data Mining and Knowledge Discovery,
3(1):12–27.
Urdaneta-Ponte, M. C., Mendez-Zorrilla, A., and
Oleagordia-Ruiz, I. (2021). Recommendation
systems for education: Systematic review. Electron-
ics, 10(1414):1611.
Yamada, I., Asai, A., Sakuma, J., Shindo, H., Takeda,
H., Takefuji, Y., and Matsumoto, Y. (2020).
Wikipedia2Vec: An Efficient Toolkit for Learn-
ing and Visualizing the Embeddings of Words
and Entities from Wikipedia. (arXiv:1812.06280).
arXiv:1812.06280 [cs].
Yamada, I., Shindo, H., Takeda, H., and Takefuji, Y. (2016).
Joint learning of the embedding of words and enti-
ties for named entity disambiguation. arXiv preprint
arXiv:1601.01343.
Zhao, Q., Wang, C., Wang, P., Zhou, M., and Jiang, C.
(2018). A novel method on information recommenda-
tion via hybrid similarity. IEEE Transactions on Sys-
tems, Man, and Cybernetics: Systems, 48(3):448–459.
EKM 2023 - 6th Special Session on Educational Knowledge Management
446