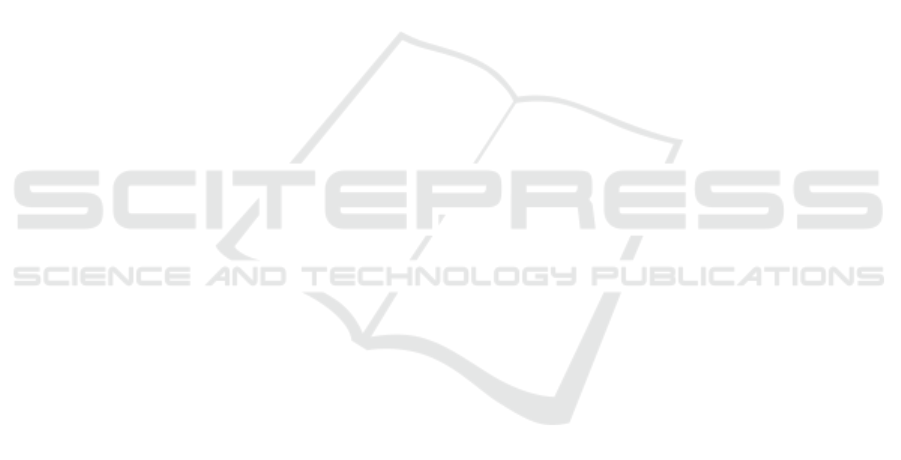
ACKNOWLEDGMENTS
We thank the reviewers for their helpful feedback and
our colleagues for insights on earlier drafts. The au-
thors acknowledge the financial support by the Fed-
eral Ministry of Education and Research of Germany
and by the Sächsische Staatsministerium für Wis-
senschaft Kultur und Tourismus in the program Cen-
ter of Excellence for AI-research "Center for Scal-
able Data Analytics and Artificial Intelligence Dres-
den/Leipzig", project identification: ScaDS.AI.
REFERENCES
Abadi, M., Chu, A., Goodfellow, I., McMahan, H. B.,
Mironov, I., Talwar, K., and Zhang, L. (2016). Deep
learning with differential privacy. In ACM SIGSAC.
Albert, E., Torres, I., Bueno, F., Huntley, D., Molla, E., F.-
F., M., Martínez, M., Poujois, S., Forqué, L., Valdivia,
A., S. A., C., Ferrer, J., Colomina, J., and Navarro, D.
(2021). Field evaluation of a rapid antigen test (pan-
bio™ covid-19 ag rapid test device) for covid-19 di-
agnosis in primary healthcare centres. CMI, 27(3).
Balthazar, T. (2018). Sharing health-data between hospitals
and other care-providers: Towards legal clarity about
what can be communicated to whom. NTvG, 74.
Bekkar, M., Djemaa, H. K., and Alitouche, T. A. (2013).
Evaluation measures for models assessment over im-
balanced data sets. Rev. inf. eng. appl., 3(10).
Carlini, N., Liu, C., Erlingsson, Ú., Kos, J., and Song,
D. (2019). The secret sharer: Evaluating and test-
ing unintended memorization in neural networks. In
USENIX Security Symposium (USENIX Security 19).
Chowdhury, M. E., Rahman, T., Khandakar, A., Mazhar,
R., Kadir, M. A., Mahbub, Z. B., Islam, K. R., Khan,
M. S., and Iqbal, A. (2020). Can ai help in screening
viral and covid-19 pneumonia? IEEE Access, 8.
Deng, J., Dong, W., Socher, R., Li, L.-J., Li, K., and Fei-
Fei, L. (2009). Imagenet: A large-scale hierarchical
image database. In CVPR. IEEE.
Dwork, C. (2008). Differential privacy: A survey of results.
In TAMC, pages 1–19. Springer.
Ganev, G., Oprisanu, B., and De Cristofaro, E. (2022).
Robin hood and matthew effects: Differential privacy
has disparate impact on synthetic data. In ICML.
He, K., Zhang, X., Ren, S., and Sun, J. (2016). Deep resid-
ual learning for image recognition. In CVPR. IEEE.
Ho, T., Tran, K., and Huang, Y. (2022). Fedsgdcovid: Fed-
erated SGD COVID-19 detection under local differ-
ential privacy using chest x-ray images and symptom
information. Sensors, 22(10):3728.
Homer, N., Szelinger, S., Redman, M., Duggan, D., Tembe,
W., Muehling, J., Pearson, J. V., Stephan, D. A., Nel-
son, S. F., and Craig, D. W. (2008). Resolving Individ-
uals Contributing Trace Amounts of DNA to Highly
Complex Mixtures Using High-Density SNP Geno-
typing Microarrays. PLOS Genetics, 4(8):e1000167.
Jagielski, M., Ullman, J., and Oprea, A. (2020). Auditing
differentially private machine learning: How private
is private sgd? NeurIPS, 33.
Jayaraman, B. and Evans, D. (2019). Evaluating differen-
tially private machine learning in practice. In 28th
USENIX Security Symposium (USENIX Security 19).
Jayaraman, B., Wang, L., Knipmeyer, K., Gu, Q., and
Evans, D. (2021). Revisiting Membership Inference
Under Realistic Assumptions. PETS, 2021(2).
Kermany, D., Goldbaum, M., Cai, W., Valentim, C., Liang,
H., Baxter, S., McKeown, A., Yang, G., Wu, X., and
Yan, F. (2018). Identifying medical diagnoses and
treatable diseases by image-based deep learning. Cell.
Kingma, D. P. and Ba, J. (2015). Adam: A method for
stochastic optimization. In ICLR.
Lange, L. (2022). Privacy-Preserving Detection of COVID-
19 in X-Ray Images. Master’s thesis, Leipzig Univ.
LeCun, Y., Bottou, L., Bengio, Y., and Haffner, P. (1998).
Gradient-based learning applied to document recogni-
tion. Proceedings of the IEEE, 86(11):2278–2324.
Malek, M., Mironov, I., Prasad, K., Shilov, I., and Tramer,
F. (2021). Antipodes of label differential privacy: Pate
and alibi. NeurIPS, 34.
Müftüo
˘
glu, Z., Kizrak, M. A., and Yildlnm, T. (2020). Dif-
ferential privacy practice on diagnosis of covid-19 ra-
diology imaging using efficientnet. In INISTA. IEEE.
Nasr, M., Song, S., Thakurta, A., Papernot, N., and Carlini,
N. (2021). Adversary instantiation: Lower bounds for
differentially private machine learning. In S&P. IEEE.
Papernot, N., Thakurta, A., Song, S., Chien, S., and Erlings-
son, Ú. (2021). Tempered sigmoid activations for deep
learning with differential privacy. In AAAI.
Rahman, M. A., Rahman, T., Laganière, R., Mohammed,
N., and Wang, Y. (2018). Membership inference at-
tack against differentially private deep learning model.
Transactions on Data Privacy, 11(1):61–79.
Rahman, T., Khandakar, A., Qiblawey, Y., Tahir, A., Ki-
ranyaz, S., Kashem, S. B. A., Islam, M. T., and
Al Maadeed, S. (2021). Exploring the effect of image
enhancement techniques on covid-19 detection using
chest x-ray images. Comput. Biol. Med.
Salem, A., Zhang, Y., Humbert, M., Fritz, M., and Backes,
M. (2019). Ml-leaks: Model and data independent
membership inference attacks and defenses on ma-
chine learning models. In NDSS. Internet Society.
Shokri, R., Stronati, M., Song, C., and Shmatikov, V.
(2017). Membership inference attacks against ma-
chine learning models. In S&P. IEEE.
Shorten, C. and Khoshgoftaar, T. (2019). A survey on image
data augmentation for deep learning. J. Big Data, 6(1).
Speranskaya, A. (2020). Radiological signs of a new coro-
navirus infection covid-19. Dia. rad. and rad., 11(1).
Yeom, S., Giacomelli, I., Fredrikson, M., and Jha, S. (2018).
Privacy risk in machine learning: Analyzing the con-
nection to overfitting. In CSF. IEEE.
Zhang, L., Shen, B., Barnawi, A., Xi, S., Kumar, N., and
Wu, Y. (2021). Feddpgan: Federated differentially
private generative adversarial networks framework for
the detection of covid-19 pneumonia. Inf. Syst. Front.
Privacy in Practice: Private COVID-19 Detection in X-Ray Images
633