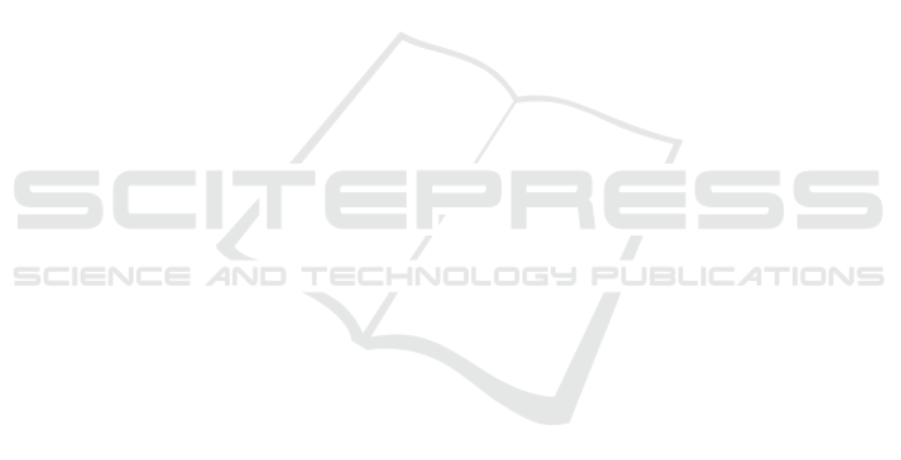
REFERENCES
(1999). Kdd cup 99. http://kdd.ics.uci.edu/databases/kddc
up99/kddcup99.html. Accessed: 2022-08-08.
Acosta, J. C., Medina, S., Ellis, J., Clarke, L., Rivas, V., and
Newcomb, A. (2021). Network data curation toolkit:
Cybersecurity data collection, aided-labeling, and rule
generation. In MILCOM 2021 - 2021 IEEE Military
Communications Conference (MILCOM), pages 849–
854.
Brauckhoff, D., Wagner, A., and May, M. (2008). Flame: A
flow-level anomaly modeling engine. In CSET.
Cermak, M., Jirsik, T., Velan, P., Komarkova, J., Spacek, S.,
Drasar, M., and Plesnik, T. (2018). Towards provable
network traffic measurement and analysis via semi-
labeled trace datasets. In 2018 Network Traffic Mea-
surement and Analysis Conference (TMA), pages 1–8.
Chou, D. and Jiang, M. (2022). A survey on data-driven net-
work intrusion detection. ACM Computing Surveys,
54(9):1–36.
Cordero, C. G., Vasilomanolakis, E., Milanov, N., Koch,
C., Hausheer, D., and M
¨
uhlh
¨
auser, M. (2015). Id2t: A
diy dataset creation toolkit for intrusion detection sys-
tems. In 2015 IEEE Conference on Communications
and Network Security (CNS), pages 739–740.
Cordero, C. G., Vasilomanolakis, E., Wainakh, A.,
M
¨
uhlh
¨
auser, M., and Nadjm-Tehrani, S. (2021). On
generating network traffic datasets with synthetic at-
tacks for intrusion detection. ACM Trans. Priv. Secur.,
24(2).
Engelen, G., Rimmer, V., and Joosen, W. (2021). Trou-
bleshooting an intrusion detection dataset: the ci-
cids2017 case study. In 2021 IEEE Security and Pri-
vacy Workshops (SPW), pages 7–12.
Ferriyan, A., Thamrin, A. H., Takeda, K., and Murai,
J. (2021). Generating network intrusion detection
dataset based on real and encrypted synthetic attack
traffic. Applied Sciences, 11(17).
Kenyon, A., Deka, L., and Elizondo, D. (2020). Are pub-
lic intrusion datasets fit for purpose characterising the
state of the art in intrusion event datasets. Computers
& Security, 99:102022.
Komisarek, M., Pawlicki, M., Kozik, R., Hołubowicz, W.,
and Chora
´
s, M. (2021). How to effectively collect and
process network data for intrusion detection? Entropy,
23(11).
Lanvin, M., Gimenez, P.-F., Han, Y., Majorczyk, F., M
´
e,
L., and Totel, E. (2022). Errors in the CICIDS2017
dataset and the significant differences in detection per-
formances it makes. In CRiSIS 2022 - International
Conference on Risks and Security of Internet and Sys-
tems, pages 1–16, Sousse, Tunisia.
Lavinia, Y., Durairajan, R., Rejaie, R., and Willinger, W.
(2020). Challenges in using ml for networking re-
search: How to label if you must. In Proceedings
of the Workshop on Network Meets AI & ML, NetAI
’20, page 21–27, New York, NY, USA. Association
for Computing Machinery.
Layeghy, S., Gallagher, M., and Portmann, M. (2021).
Benchmarking the benchmark – analysis of synthetic
nids datasets.
Moustafa, N., Hu, J., and Slay, J. (2019). A holistic re-
view of network anomaly detection systems: A com-
prehensive survey. Journal of Network and Computer
Applications, 128:33–55.
Moustafa, N. and Slay, J. (2015). Unsw-nb15: a compre-
hensive data set for network intrusion detection sys-
tems (unsw-nb15 network data set). In 2015 Mili-
tary Communications and Information Systems Con-
ference (MilCIS), pages 1–6.
Rajasinghe, N., Samarabandu, J., and Wang, X. (2018).
Insecs-dcs: A highly customizable network intrusion
dataset creation framework. In 2018 IEEE Canadian
Conference on Electrical & Computer Engineering
(CCECE), pages 1–4.
Ring, M., Wunderlich, S., Scheuring, D., Landes, D., and
Hotho, A. (2019). A survey of network-based in-
trusion detection data sets. Computers & Security,
86:147–167.
Sarhan, M., Layeghy, S., Moustafa, N., and Portmann,
M. (2020). Netflow datasets for machine learning-
based network intrusion detection systems. In Big
Data Technologies and Applications, pages 117–135.
Springer.
Sarhan, M., Layeghy, S., Moustafa, N., and Portmann, M.
(2021a). A cyber threat intelligence sharing scheme
based on federated learning for network intrusion de-
tection.
Sarhan, M., Layeghy, S., and Portmann, M. (2021b). Eval-
uating standard feature sets towards increased gener-
alisability and explainability of ml-based network in-
trusion detection.
Sarhan, M., Layeghy, S., and Portmann, M. (2021c). To-
wards a standard feature set for network intrusion de-
tection system datasets. Mobile Networks and Appli-
cations, 27(1):357–370.
Sharafaldin, I., Gharib, A., Lashkari, A. H., and Ghor-
bani, A. A. (2018a). Towards a reliable intrusion
detection benchmark dataset. Software Networking,
2018(1):177–200.
Sharafaldin, I., Lashkari, A. H., and Ghorbani, A. A.
(2018b). Toward generating a new intrusion detection
dataset and intrusion traffic characterization. ICISSp,
1:108–116.
Tavallaee, M., Bagheri, E., Lu, W., and Ghorbani, A. A.
(2009). A detailed analysis of the kdd cup 99 data
set. In 2009 IEEE symposium on computational intel-
ligence for security and defense applications, pages
1–6. Ieee.
Wolsing, K., Wagner, E., Saillard, A., and Henze, M.
(2021). Ipal: Breaking up silos of protocol-dependent
and domain-specific industrial intrusion detection sys-
tems.
Yang, Z., Liu, X., Li, T., Wu, D., Wang, J., Zhao, Y.,
and Han, H. (2022). A systematic literature review
of methods and datasets for anomaly-based network
intrusion detection. Computers & Security, page
102675.
SECRYPT 2023 - 20th International Conference on Security and Cryptography
658