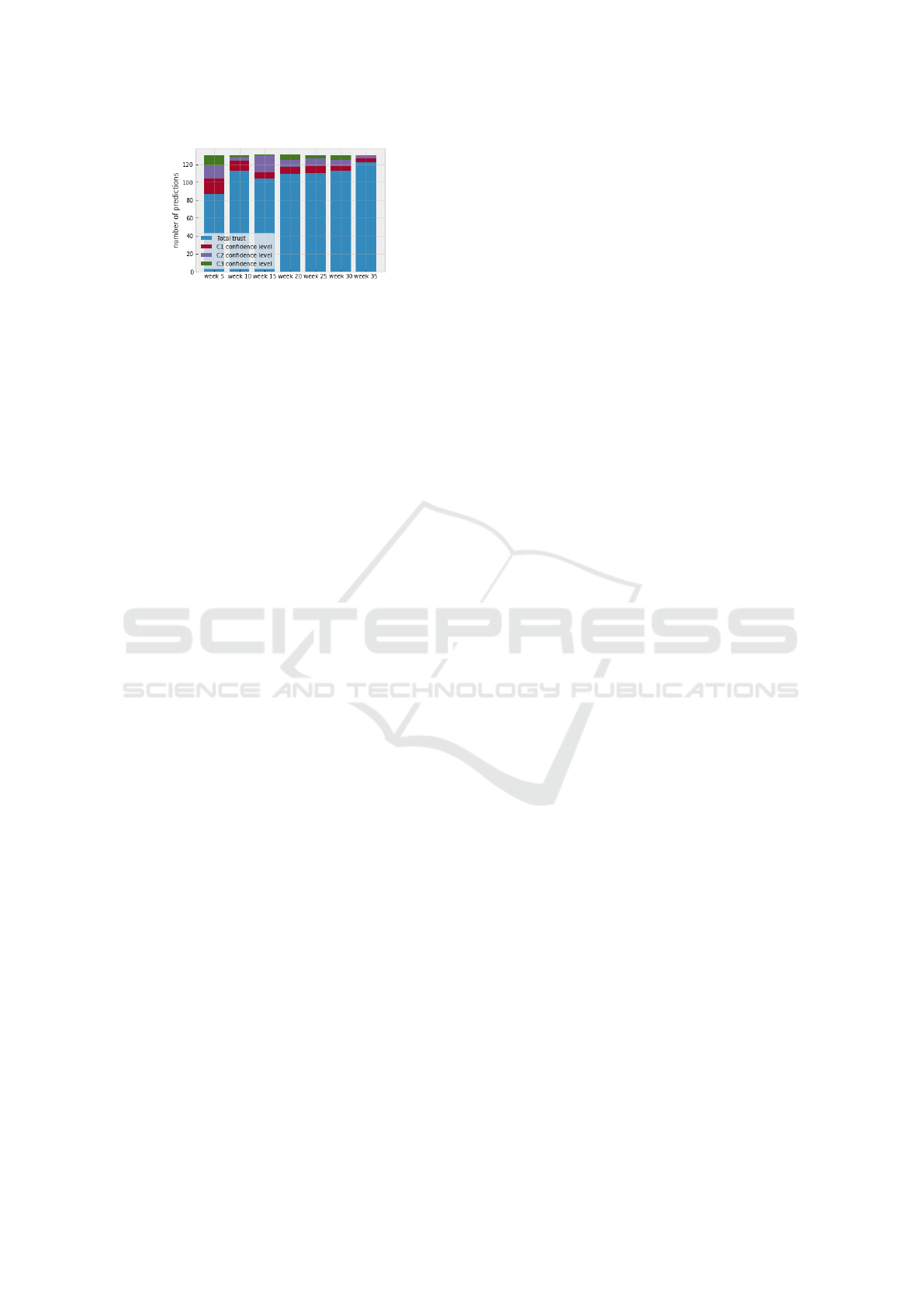
Figure 9: Number of prediction per each confidence level.
6.4 Discussion
In summary, the experimental study yielded the fol-
lowing results.
Following the Algorithm 1, we obtain confidence
levels that are more correlated with the overall accu-
racy of the system. As a result, we have narrow con-
fidence intervals and a more accurate TI of the over-
all trust granularity that is more representative of the
reliability of the system performance. For the same
system and at the same prediction time, the TI of the
prediction reliability is different from one class label
to another. This TI depends on the population of that
class and the ability of the system to correctly detect
the learners of that class. For the same system and at
the same prediction time, the TI of the prediction reli-
ability is different from a single data point to another.
In addition, the TI of a same data point changes over
time and can take on several values.
7 CONCLUSION
In this paper, we established an approach to measure
how far a teacher can trust the predictive performance
of an LA system. We started by defining three trust
granularities in an LA system, including : the overall
trust, trust per class label and trust per each predic-
tion made by the system. Then, for each trust gran-
ularity, we proceeded to the calculation of a TI us-
ing the confidence level and confidence intervals of
statistics. The experimental results show the impor-
tance of going through all these trust granularities to
get a detailed idea of the reliability of the system per-
formance. In fact, The TI of the overall trust gran-
ularity shows the teacher how much she/he can trust
the overall performance of a predictive system. Both
other trust granularities give a finer idea of how much
trust to be granted to the system when it comes to a
particular class or prediction. Indeed, at the same pre-
diction time, the TI is different from one class label
to another and also from one single prediction to an-
other. This notion of trust comes into play in order to
ensure an effective intervention adapted to the situa-
tion of each learner or group of learners.
As a perspective of this work we intend to propose
a global trust index for the whole system computed
from the TI of the three trust granularities.
REFERENCES
Baneres, D., Guerrero-Rold
´
an, A. E., Rodr
´
ıguez-Gonz
´
alez,
M. E., and Karadeniz, A. (2021). A predictive analyt-
ics infrastructure to support a trustworthy early warn-
ing system. Applied Sciences, 11(13):5781.
Ben Soussia, A., Labba, C., Roussanaly, A., and Boyer,
A. (2022). Time-dependent metrics to assess perfor-
mance prediction systems. The International Journal
of Information and Learning Technology, 39(5):451–
465.
Biedermann, D., Schneider, J., and Drachsler, H. (2018).
Implementation and evaluation of a trusted learning
analytics dashboard. In EC-TEL (Doctoral Consor-
tium).
Bitkina, O. V., Jeong, H., Lee, B. C., Park, J., Park, J., and
Kim, H. K. (2020). Perceived trust in artificial intel-
ligence technologies: A preliminary study. Human
Factors and Ergonomics in Manufacturing & Service
Industries, 30(4):282–290.
Lockey, S., Gillespie, N., Holm, D., and Someh, I. A.
(2021). A review of trust in artificial intelligence:
Challenges, vulnerabilities and future directions.
L
´
opez Zambrano, J., Lara Torralbo, J. A., Romero Morales,
C., et al. (2021). Early prediction of student learn-
ing performance through data mining: A systematic
review. Psicothema.
Petty, M. D. (2012). Calculating and using confidence
intervals for model validation. In Proceedings of
the Fall 2012 Simulation Interoperability Workshop,
pages 10–14.
Qin, F., Li, K., and Yan, J. (2020). Understanding user
trust in artificial intelligence-based educational sys-
tems: Evidence from china. British Journal of Edu-
cational Technology, 51(5):1693–1710.
Raschka, S. (2018). Model evaluation, model selection,
and algorithm selection in machine learning. arXiv
preprint arXiv:1811.12808.
Siau, K. and Wang, W. (2018). Building trust in artificial
intelligence, machine learning, and robotics. Cutter
business technology journal, 31(2):47–53.
Stanton, B., Jensen, T., et al. (2021). Trust and artificial
intelligence. preprint.
Susnjak, T., Ramaswami, G. S., and Mathrani, A. (2022).
Learning analytics dashboard: a tool for providing
actionable insights to learners. International Jour-
nal of Educational Technology in Higher Education,
19(1):12.
Tsai, Y.-S., Whitelock-Wainwright, A., and Ga
ˇ
sevi
´
c, D.
(2021). More than figures on your laptop:(dis) trust-
ful implementation of learning analytics. Journal of
Learning Analytics, 8(3):81–100.
How Far Can We Trust the Predictions of Learning Analytics Systems?
157