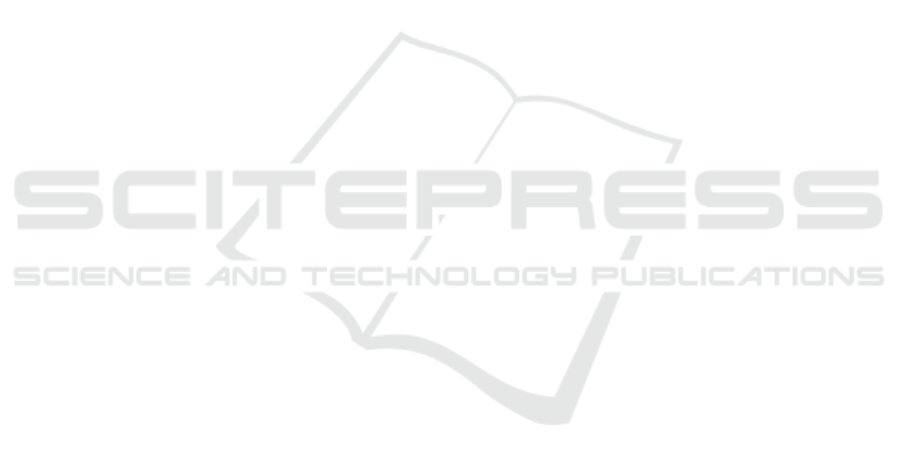
(Society for the Study of Artificial Intelligence and the
Simulation of . . . .
Briot, J.-P. and Pachet, F. (2020). Deep learning for music
generation: challenges and directions. Neural Com-
puting and Applications, 32(4):981–993.
Bull, S. (2020). There are Open Learner Models
About! IEEE Transactions on Learning Technologies,
13(2):425–448.
Carnovalini, F. (2019). Open Challenges in Musical
Metacreation. In Proceedings of the 5th EAI Inter-
national Conference on Smart Objects and Technolo-
gies for Social Good, pages 124–125, Valencia Spain.
ACM.
Carnovalini, F., Harley, N., Homer, S. T., Rod
`
a, A., and
Wiggins, G. A. (2021a). Meta-Evaluating Quantita-
tive Internal Evaluation: a Practical Approach for De-
velopers. In Proceedings of the 12th International
Conference on Computational Creativity, page 5,
M
´
exico Virtual. Association for Computational Cre-
ativity.
Carnovalini, F. and Rod
`
a, A. (2020). Computational Cre-
ativity and Music Generation Systems: An Introduc-
tion to the State of the Art. Frontiers in Artificial In-
telligence, 3:14.
Carnovalini, F., Rod
`
a, A., Harley, N., Homer, S. T., and
Wiggins, G. A. (2021b). A New Corpus for Compu-
tational Music Research andA Novel Method for Mu-
sical Structure Analysis. In Audio Mostly 2021 (AM
’21), page 4, virtual/Trento Italy. ACM.
Chiu, M.-L. (2002). An organizational view of design com-
munication in design collaboration. Design Studies,
23(2):187–210.
Cho, K., Van Merri
¨
enboer, B., Gulcehre, C., Bahdanau, D.,
Bougares, F., Schwenk, H., and Bengio, Y. (2014).
Learning phrase representations using RNN encoder-
decoder for statistical machine translation. arXiv
preprint arXiv:1406.1078.
Herremans, D. and Chew, E. (2017). MorpheuS: gener-
ating structured music with constrained patterns and
tension. IEEE Transactions on Affective Computing,
10(4):16.
Jordanous, A. (2012). A standardised procedure for evalu-
ating creative systems: Computational creativity eval-
uation based on what it is to be creative. Cognitive
Computation, 4(3):246–279.
Kaleli, Y. S. (2020). The Effect of Computer-Assisted
Instruction on Piano Education: An Experimental
Study with Pre-Service Music Teachers. Interna-
tional Journal of Technology in Education and Sci-
ence, 4(3):235–246. Publisher: ERIC.
Nakamura, E. and Yoshii, K. (2018). Statistical piano re-
duction controlling performance difficulty. APSIPA
Transactions on Signal and Information Processing,
7.
Nierhaus, G. (2009). Algorithmic Composition: Paradigms
of Automated Music Generation. Springer Science &
Business Media. Google-Books-ID: jaowAtnXsDQC.
Ramoneda, P., Tamer, N. C., Eremenko, V., Serra, X.,
and Miron, M. (2022). Score Difficulty Analysis
for Piano Performance Education based on Finger-
ing. In ICASSP 2022 - 2022 IEEE International Con-
ference on Acoustics, Speech and Signal Processing
(ICASSP), pages 201–205, Singapore. IEEE.
Rigby, L., W
¨
unsche, B. C., and Shaw, A. (2020). piARno
- An Augmented Reality Piano Tutor. In 32nd Aus-
tralian Conference on Human-Computer Interaction,
pages 481–491, Sydney NSW Australia. ACM.
Roberts, A., Engel, J., Raffel, C., Hawthorne, C., and Eck,
D. (2018). A hierarchical latent vector model for
learning long-term structure in music. In International
conference on machine learning, pages 4364–4373.
PMLR.
Smoliar, S. W., Waterworth, J. A., and Kellock, P. R. (1995).
pianoFORTE: a system for piano education beyond
notation literacy. In Proceedings of the third ACM
international conference on Multimedia - MULTIME-
DIA ’95, pages 457–465, San Francisco, California,
United States. ACM Press.
S
´
ebastien, V., Ralambondrainy, H., S
´
ebastien, O., and Con-
ruyt, N. (2012). Score analyzer: Automatically de-
termining scores difficulty level for instrumental e-
learning. In ISMIR 2012, pages 571–576.
Takidaira, S., Shoji, Y., and D
¨
urst, M. J. (2022). Slow, Re-
peat, Voice Guidance: Automatic Generation of Prac-
tice Charts to Improve Rhythm Action Games. In
Fang, X., editor, HCI in Games, volume 13334, pages
119–138. Springer International Publishing, Cham.
Series Title: Lecture Notes in Computer Science.
Temperley, D. (2011). Composition, Perception, and
Schenkerian Theory. Music Theory Spectrum,
33(2):146–168.
Wiggins, G. A. and Trewin, S. (2000). A System for Con-
cerned Teaching of Musical Aural Skills. In Goos, G.,
Hartmanis, J., van Leeuwen, J., Gauthier, G., Frasson,
C., and VanLehn, K., editors, Intelligent Tutoring Sys-
tems, volume 1839, pages 494–503. Springer Berlin
Heidelberg, Berlin, Heidelberg. Series Title: Lecture
Notes in Computer Science.
CSME 2023 - 4th International Special Session on Computer Supported Music Education
412